Please can you explain what deep learning algorithms are and how they could help to uncover disease-causing genetic mutations?
To understand deep learning in the context of genetic disease, you need to understand shallow learning first. Shallow learning relates mutations to diseases by looking for mutations that commonly occur in patients with a disease. It’s a commonly used method.
However, doing that almost always fails to identify the mutation that causes the disease, because mutations occur in clusters and the true causal one will be buried in a cluster of non-causal mutations that are all correlated with the disease.
The true causal mutation leads to disease through a cascade of cellular processes that occur within living cells and deep learning can be used to train a computer system to account for this cascade of processes.
By the way, identifying the true causal mutation behind a disease is important for confidently determining that a patient has the disease.
Also, it’s important for figuring out how to treat the disease by reversing the effect of the mutation using a drug or gene editing.
Recent data shows the chances of success of pharmaceutical R&D could be increased by over three times by accounting for this kind of important genetic information.
Why is predicting protein binding particularly important when trying to assess how likely a genetic mutation is to cause a problem?
DNA is often called the “book of life.” How do cells read that book? Well, they make use of proteins, which scan DNA, recognize words within the DNA, bind to those words and then cause other things to happen. So proteins play an important role in the cascade of cellular processes that I just described.
DNA mutations often prevent proteins from binding where they should bind, or they cause proteins to bind when they shouldn’t. For this reason, the ability to accurately ascertain when and where proteins will bind is very important in understanding and treating disease.
Why is it difficult to predict when and where proteins will bind to DNA and RNA sequences?
It’s very hard! Whether or not a protein binds is determined by biochemical reactions that are very complex and are delicately tuned to the text within the DNA or RNA sequence.
In fact, even whether a protein binds is a consequence of a cascade of processes that involves the presence of certain words within the DNA or RNA sequence, combinations of those words, and the presence of other proteins. This makes predicting protein binding a hard problem.
Can you please give an overview of DeepBind and explain how it computes whether a protein would bind to a sequence and thereby influence cellular processes?
DeepBind, as the name suggests, uses deep learning to combine patterns identified within DNA or RNA sequences in a cascade of effects, to determine whether or not a protein will bind.
The cascade of effects that are learnt by DeepBind may or may not correspond to what goes on within the cell, but what’s important is that DeepBind can account for complex combinations of words within DNA or RNA.
Since actual cellular processes can involve these complex combinations, DeepBind can find relationships that can’t be found using shallow learning techniques.
You know, DeepBind doesn’t make perfect predictions, but we compared it against other techniques using the most comprehensive dataset ever examined, and DeepBind performed best.
What impact has DeepBind had so far in aiding analysis of human genetic data?
We’re excited by the possibilities that DeepBind can open up for clinical and R&D work around the world. We and others have used DeepBind to identify mutations that disrupt protein binding within the context of disease.
At this point, it is clear that DeepBind can identify relationships between mutations and disease that can’t be found by industry-standard methods. For instance, we have used DeepBind to examine mutations that disrupt protein binding or cause erroneous protein binding, in cases of familial hypercholesterolemia and ovarian cancer.
How does DeepBind build upon Deep Genomics’ first tool in the system SPIDEX, which focuses on splicing? Will the two tools be linked in the computational system?
It’s much more than that! Deep Genomics has an aggressive science and technology roadmap for building a computational system that links together many components that account for different cellular processes.
Think about the Google search engine, but for human mutations. Our first product, SPIDEX, is just one part of that system. In fact, SPIDEX already relies on a simple system that predicts where proteins will bind. We will replace that system with DeepBind and produce SPIDEX data that is much more accurate. All of these components interact and improving one of them boosts the performance of the others. That’s also how deep learning works in general.
What further tools do Deep Genomics plan to develop and what do you think the future holds for deep learning and genome biology?
That’s a good question. Actually, we aren’t planning on developing tools! At least, not in the normal sense of the word.
We’ve realized that the technology that we’re developing is much bigger than a tool. Each component that we develop could be used as a tool, but it’s much more valuable for it to be used as a dynamic component within a large “engine.”
Genetic data that is fed into the Deep Genomics engine can be used to generate crucial information about a patient’s disease, to identify therapeutic treatments, or to connect together patients, but it can also be used to improve the engine.
Just like connections between web pages form the basis of the Google search engine, connections between cellular processes form the basis of the Deep Genomics engine. As we gather more data, we can improve the biological accuracy of these connections.
An important issue is patient confidentiality, but there are ways for ensuring that the data for patients can be examined for their benefit, without transferring information to our database.
What does the future hold for machine learning and genome biology? I believe that genome biology, and more specifically genomic medicine, is the next big frontier for deep learning. Ten years ago, I gave a talk at one of the early workshops on deep learning organized by the leaders in the field.
The other attendees were studying computer vision, speech recognition and text processing. I pointed out that while those are compelling problems to study using deep learning, humans are already very good at them. In contrast, humans are not good at understanding the text of the genome.
So, I explained, using deep learning to understand the genome and how genetic mutations lead to disease takes deep learning to a new level: understanding things that human’s cannot.
You know, it’s not a coincidence that one of those “fathers of deep learning”, Yann LeCun, who is the Director of AI at Facebook, is on the scientific advisory board of Deep Genomics. Deep learning is going to transform genomic medicine.
Where can readers find more information?
The company web site: Deep Genomics
About Brendan Frey, PhD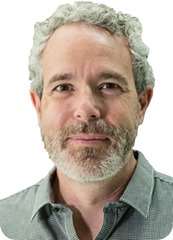
Brendan has made fundamental contributions to the fields of machine learning and genome biology, both in research and in industry. He led the team that developed a deep learning method for identifying the splicing-related genetic determinants of disease, which was published in the January 9, 2015 edition of Science Magazine.
In the past twenty years, he has co-authored over 12 papers in Science, Nature and Cell, including one of the first papers on deep learning (Science, 1995) and one of the first papers describing a computer system that can predict the cellular process of alternative splicing (Nature 2010).
Brendan is a co-inventor of the affinity propagation algorithm and of the factor graph notation for graphical models. He has consulted for over a dozen machine learning-powered companies, has served on the technical advisory board of Microsoft Research, holds seven patents, and has served as an expert witness in patent litigation.
Brendan is most proud of his former team members, which include entrepreneurs, industrial researchers, cool programmers, and professors at highly recognized centers in Canada, the United States, England and Europe.