Can you please give an overview of the recent meta-analysis published in Nature Genetics that identified 44 variants associated with migraines?
There's a big debate right now in the migraine community around the underlying cause of the disease. The question is whether it is vascular, and has something to do with our vessels, or whether it has to do with our neurons not functioning correctly or misfiring.
There's not a lot of genetic evidence to support either hypothesis. This study was aimed at trying to, firstly, find genetic determinants of migraine and then, to use those discoveries to understand more about the underlying mechanisms of migraine.
The basic idea behind this study was to do a meta-analysis. A meta-analysis is when you simply take a bunch of studies, in this case twenty-two, and combine the results so that you end up with a more powerful study.
We call that increasing statistical power. You could think about it as just increasing your ability to discover things. By combining a bunch of different studies, you effectively increase the number of individuals that are contributing to the final results and you end up increasing your ability to discover.
The end result is that we identified 45 association signals that encompass 38 different regions of the genome. About 28 of these were novel, meaning they were not previously known from other studies, so this is quite a significant finding in the genome-wide association world.
One of the most interesting takeaways from this is that many of the regions identified harbor genes that have something to do with maintaining the vasculature, so maintaining blood vessels and their structure. That lends evidence to the hypothesis that migraine is vascular in nature.
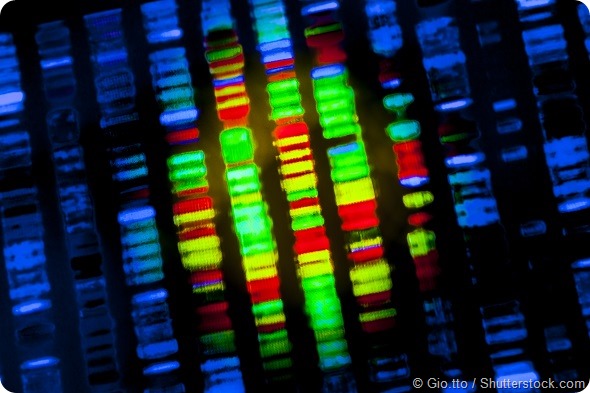
Do the results mean that migraines are genetic in part? Are environmental factors also at play?
In the statistical genetics world, we refer to traits like migraine as 'complex traits.' These are traits that are caused by genetic factors and also by lifestyle and environment.
We typically try to quantify “how genetic” a trait is by estimating something we call heritability. I'm not sure people are familiar with that concept, but heritability can be thought of as the fraction of the disease that's caused by genetics or influenced by genetics.
The heritability for migraine is estimated to be about 40%. That number would vary depending on where you look, but if you look in this paper, for example, I think they quoted 42%. That means that approximately 60% of migraine is probably non genetic and very well could have something to do with lifestyle or environment.
How does that compare to other conditions?
A good reference would be height. That’s kind of the canonical complex genetic trait. The heritability for height is about 80%. We think of height as being a very genetic trait, but it's only 80%, so I would say that heritability of migraines being 40% is actually pretty substantial.
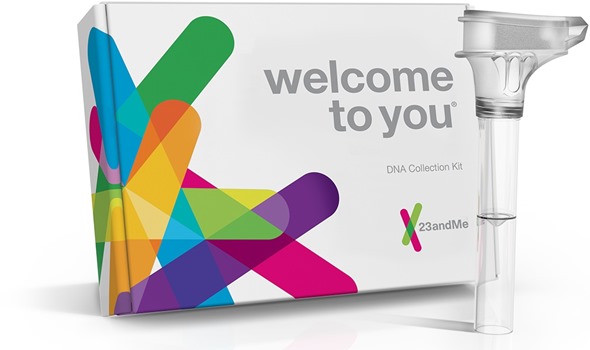
What impact do you think the study’s findings will have on migraine treatment?
Generally, these kinds of studies can lead to a deeper understanding of how migraine or other diseases are caused. Once we understand that, we can also start to understand sub-types of the disease.
We know that migraine has a lot of variation at the phenotypic level. When people go to the doctor, there are lots of questions they can ask about the nature of their migraines, when they occur, what their triggers are, how long they last and lots more.
However, the underlying molecular mechanisms that are fueling those differences between people are not really understood.
If we gain a better understanding of those, then we can start to have a more fine-grained sub-typing of the phenotype, which will then lead to development of better treatments, treatments that are specific to different sub-types and also just a better understanding of what works for different people.
I think a lot of people with migraines, for example, self-experiment. They try to figure out what their triggers are and what things they should avoid.
If we understand the underlying biology, then we can potentially use the genetic results as a starting point for understanding the sub-types and then help people understand what works for them as far as treatments go and so on.
What role did data from 23andMe customers play in this study?
As I mentioned, this is a meta-analysis, so it combined 22 previous studies or 22 separate groups of summary statistics.
23andMe was one of these collaborators and I think, in all, we contributed data that was derived from about a 170,000 people consented to participate in 23andMe research, which was the largest contribution.
Second, I believe, was deCODE Genetics which was a little over maybe 100,000 people. Other ones were a little bit smaller than that.
In addition to being used by its own scientists, 23andMe have opened up the use of its data to others including researchers in Australia, the UK and Europe. Can you please outline 23andMe’s vision for this data sharing?
First of all, it's important to mention that data privacy is something we take very seriously, so when we talk about data sharing, what we mean is that we're only ever sharing de-identified, summary level data or aggregate statistics with collaborators.
The only time we don't do this, it's very explicit and we have to re-consent our participants, so they know that their individual level data would be shared.
However, that's a rare occurrence and it's something that we have to deal with explicitly. When we talk about data sharing for this and for other academic collaborations, we're always talking about summary level data.
When it comes to summary level data, we share these kind of results with both academic collaborators who are interested in just basic research and also industry partners. One of our motivations for this kind of sharing is that we realize how much value there is in the data.
I came straight here from grad school and it was just unbelievable to me the kind of signal you could get out of really large sample sizes. We realize internally the value of that data, and that it can provide us with results that lead to a better understanding of how genetics influences diseases.
We don't really have the resources to analyze all the data that we have and we don't have the experts in-house that know everything about migraine or everything about heart disease and so forth.
We found that a really good way to use the data in a way that could benefit the scientific community is to do academic collaborations like this. It's one of our main motivators.
We also share summary level data with industry partners. We collaborate with some pharma companies and also smaller companies that are trying to create new types of products and things.
All of it is aimed at ultimately helping people, whether that's finding a drug or creating something new that could help influence someone's wellness, that's what we're interested in.
Currently, we evaluate the opportunities for collaborating and data sharing on a case by case basis.
What other biotech applications have you found for 23andMe’s data?
We have two main technologies that I think drive a lot of potential applications. One is the technology that was used in this migraine paper, which is a genome-wide association study or GWAS. The second is the phenome-wide association study or PheWAS.
In a GWAS, we ask what genetic variations across the genome are associated or potentially have some influence on a phenotype such as migraine. In a PheWAS study, we ask a different question. We say, given a genetic variation or given a gene, what phenotypes do we see that are associated with it?
For instance, we could look at the gene that we identified as being associated with migraine and then we could look across thousands of the phenotypes, to see what others are associated with that gene.
If we look at that gene and look across all of our phenotypes, maybe we will see that this gene is also associated with cardiovascular disease, stroke and so forth. Both the GWAS and the PheWAS technologies can be used in conjunction to help us understand how gene networks affect phenotypic networks.
Those are the two things that drive a lot of potential applications. I view it as two core technologies and the information coming out of it is very general. The applications vary widely and there are therapeutic and preventative applications of that kind of information.
We can also use these types of data to develop predictive models. So, if we're interested in trying to predict whether somebody is more likely to get a disease than someone else based on their genetics, then that kind of data can drive statistical models that could do that. That's something that we're constantly working on.
Please can you tell us about 23andMe data and Apple's ResearchKit?
We have a research module for any kind of researcher to use and develop applications for IOS, for Apple devices essentially. People are using it already. There's an asthma study and a researcher is using our research module to collect data with the mobile application. Also, Stanford is doing a heart study that's utilizing it.
It's definitely something researchers can take advantage of. It's not something that we would build; it's kind of a framework that researchers can use to build applications for studies and then be able to incorporate 23andMe data if the 23andMe member were willing to do so and consented to the research protocol and so on.
How important do you think genetic data will be in developing future treatments for migraines and other conditions?
I'm incredibly biased because this is why I'm in this whole industry in the first place! When I got into this, I thought, and still think, that genetics just has so much potential future power for really changing medicine.
I think genetics is obviously going to be really important for discovering how things work and what the molecular mechanisms behind diseases are, but also for treatment of diseases, development of new therapies, and prevention.
As I've said a couple of times, studies such as the migraine one can lead us to the genes that underlie diseases and we'll start to understand better how these things work, but the more future promise I think of genetics lies in the personalized medicine space, which has been promised for quite some time now and hasn't really been realized. I think it's on the way, or hopefully is, but I'm not sure what the timescale is.
One of the things I think about is, for example, that right now you go to the doctor and they determine your baseline risk for something, based on high-level demographic features.
They look at your age, your gender and your family history and then do a calculation. Sometimes, that's a rigorous calculation and sometimes it’s a kind of back of the envelope calculation, to determine what your baseline risk is. Then they figure out what actions you should take based on that.
I see a future where we can evaluate someone's baseline risk based on their genetic makeup and this could have huge implications.
For example, if you're more likely to have high cholesterol at baseline, then your doctor might make a different decision about giving you statins at a certain age compared to somebody who was more likely to have low cholesterol at baseline.
Or, if you had a strong predisposition for having blood clots, then it might be more important for you to really listen to the advice that you should wear compression socks when on the airplane.
I see these types of studies as being really the first step towards that world, towards understanding how all these genetic variations work in conjunction with our environment and our lifestyle to determine what our risk for disease is and then how we can potentially mitigate that risk.
Where can readers find more information?
https://blog.23andme.com/23andme-research/new-genetic-associations-found-for-migraines/
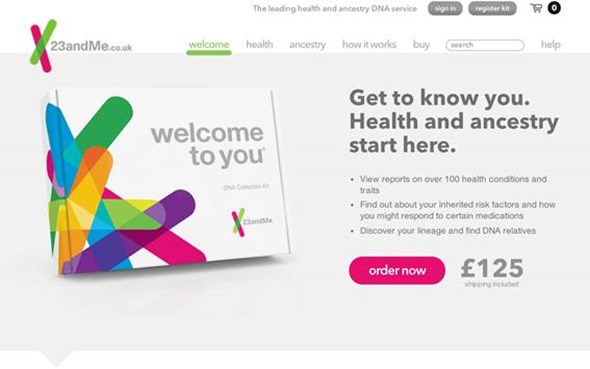
About Nick Furlotte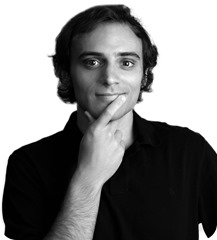
Nick Furlotte, Senior Scientist Statistical Geneticist and Health R&D
Originally from: Memphis, TN
Education: BA: Computer Science, University of Memphis
MS: Bioinformatics, University of Memphis
PhD: Computer Science, UCLA
His interests are in computational and statistical genetics, complex trait analysis and risk prediction, Machine learning, and linear mixed models.
For more, and his CV go to: http://whatmind.com/ (His CV has a list of papers he's published or presented.)