Diagnosing aneurysms before they rupture is critical for the prognosis of patients. When aneurysms rupture, they can lead to life-threatening subarachnoid hemorrhages, a type of stroke.
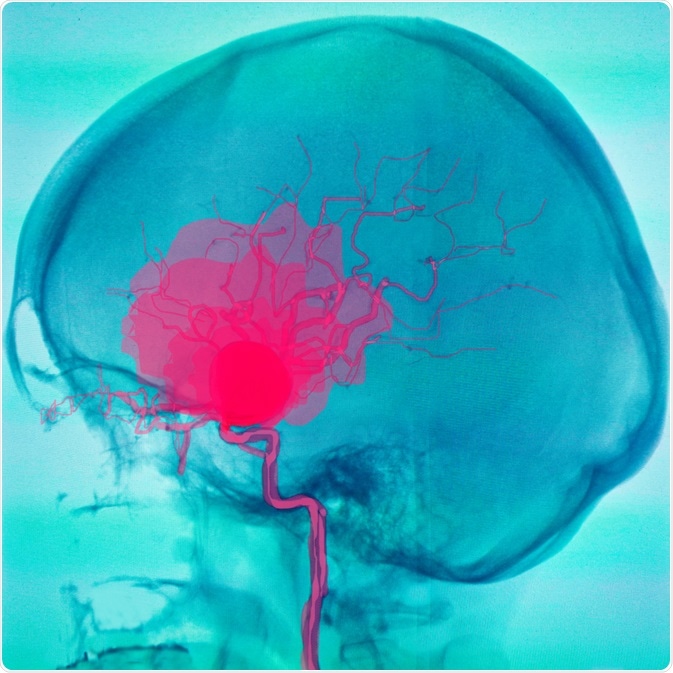
Image Credit: Semnic/Shutterstock.com
Current techniques used to diagnose unruptured aneurysms include CT scans, however, interpreting these scans are difficult even for the most trained radiologists and there is low interrater agreement among experts.
Artificial Intelligence (AI) and Reliable Diagnoses
A recent study published in JAMA Netw Open by Park and colleagues from Stanford University set out to investigate whether augmenting CT scan angiogram data with Artificial Intelligence (AI) deep learning models improved the diagnosis rates for patients with aneurysms.
Over 9000 CT scan angiograms performed at Stanford University Medical center were retrospectively assessed by the researchers. Other types of stroke and other vascular findings in addition to trauma-related incidents were excluded. These images were all examined by a board-certified radiologist specializing in neurology with over 12 years of experience, producing the reference standard for all examinations. The aneurysms were manually marked by the radiologists over a series of slices.
An AI model was developed called HeadXNet that segments aneurysms from CT scans. This model calculates the probability of an aneurysm in each voxel (3D-pixel) and maps a volume by expanding a low-resolution encoding to a full resolution segmentation volume. (To read more about the model development and training, see Methods section of the link to the paper).
The Study
The researchers performed a small diagnostic accuracy study. They asked 8 clinicians, of which 6 were radiologists to assess 115 images, with and without model augmentation. These clinicians were blinded to the case reports and asked to score the presence of at least one clinically significant aneurysm (having a diameter greater than 3mm). The time it took them to make a diagnosis was also logged. With the AI augmentation, a region of interest was overlaid on top of the CT scans.
Without AI augmentation, the clinicians collectively achieved a detection score of 0.831 with an accuracy of 0.893. With AI augmentation the clinicians achieved a detection score of 0.893 and an accuracy of 0.932. The model itself had a detection score of 0.949 and an accuracy of 0.809. As such, with AI augmentation there was a significant increase to the average detection sensitivity and accuracy in detecting aneurysms by the clinicians. Furthermore, the interrater reliability of diagnosis i.e. the consensus between clinicians was also significantly improved.
From this study, it is safe to conclude that this specific AI model was not only able to successfully detect clinically significant aneurysms from CT angiograms but also enhanced and improved interrater reliability of diagnosing clinically relevant aneurysms between a cohort of clinicians, surgeons, and radiologists. Therefore, integrating AI-assisted diagnostic tools may augment clinical performance that is reliable and accurate. However, it should be noted that the study was small, and the cohort was mixed in training and ability.
It is also important to note that whilst this AI model has great sensitivity for aneurysms, its performance on other cerebrovascular pathologies, or in the presence of stents, coils and other medical devices are unknown. The work was carried out from a cohort of patients and clinicians from one institution with specific scanners and imaging protocols, which is not the case with other institutes and hospitals. However, it is a promising starting point, and with further training of different clinical presentations, singular or in combination, as well as inputs from different scanning techniques, the AI will only become better over time.
Sources:
- Park et al, 2019. Deep Learning-Assisted Diagnosis of Cerebral Aneurysms Using the HeadXNet Model. JAMA Netw Open. 2(6):e195600. https://www.ncbi.nlm.nih.gov/pubmed/31173130