A large problem to be overcome in improving cancer treatment is tumor heterogeneity.
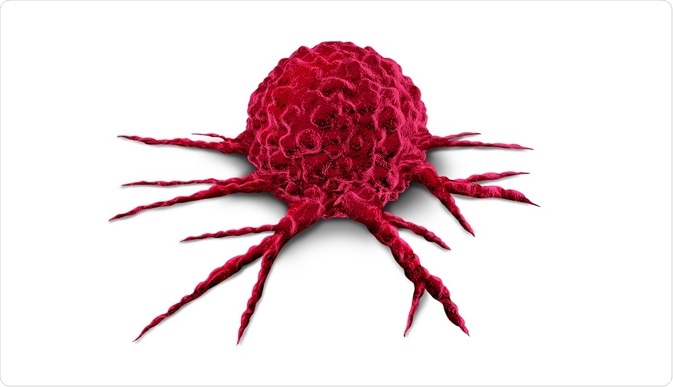
Credit: Lightspring/Shutterstock.com
As cancer progresses, the tumor begins to consist of more diverse cells with a range of molecular signatures and variable sensitivity to treatment.
Cancer evolution and resistance to treatment is caused by tumor heterogeneity, therefore a greater comprehension of the underlining dynamics that drive cancer cell variation is fundamental to the development of new, more effective therapies.
Resistance to treatment can be caused by the growth of pre-existing sub-clonal populations or the evolution of cells resistant to drugs.
Heterogeneous tumors are divided into spatial and temporal types depending on whether the non-uniform distribution of cancer cells is dispersed across and within disease sites, or if there is cell variation over time.
Current methods of examining tumor heterogeneity analyze bulk specimens but are limited because of the admixture of diverse cancer cell types and non-malignant cells. The advent of single-cell sequencing, which provides the ability to characterize individual cells within a diverse population, can now define complex clonal relationships.
Causes of tumor heterogeneity
Cancer is not a stagnant disease and the genomic instability within tumor cells provides the genetic diversity underpinning tumor heterogeneity.
Genomic instability ranges from single-base substitutions to the doubling of whole genomes. It is caused by exposure to mutagens such as UV radiation or faults in the internal regulation of processes, including DNA replication and repair.
Though not a baseline contributor, chemotherapy may also create genomic instability by increasing the mutational spectrum of the tumor. Studies have found that some cancers integrate endogenous homostatic processes to increase the overall mutational burden. This indicates that tumorigenesis is connected to a higher spontaneous mutation rate.
Genomic instability within brain tumors is produced from chromosomal changes, meaning that whole genome-segments are removed or doubled because of segregation errors formed during cell division.
The clonal evolution/selection framework
The clonal evolution/selection framework was developed in 1976 to explain how clonal diversity is maintained and describes two patterns of evolution. During linear tumor evolution there is a successive acquisition of mutations that provide a survival advantage or growth promotion.
Genetic instability produces a new clone type with a fitness advantage that is then outcompeted by the next emerging subclones. Nonetheless, recent studies have challenged the assumption that subclones must be in competition.
Cooperation between subpopulations is necessary for tumor propagation where there are non-autonomous initiating events. The second pattern that can emerge is branching evolution where multiple genetically distinct populations form from a common ancestral clone.
Branching evolution inherently produces an environment that is more likely to create a heterogeneous tumor. Linear evolution is most commonly found in haematological malignancies whilst branched evolution often forms solid tumors.
Overcoming heterogeneity during cancer treatment
Higher levels of tumor heterogeneity can lead to a reduced effectiveness of cancer therapies, including the use of targeted agents. One method of combating this problem is the utilization of mathematical modeling.
Simulations can determine the optimal dosage schedule for the withdrawal of targeted therapies that prevent selection of drug resistant cells and provide time for vulnerable cells to repopulate. Modeling can also be employed for designing successful combinational anticancer treatments.
Studies have found that optimal drug combination for maximum tumor cell death with minimal outgrowth of clonal subpopulations can be defined through RNA inference models. Short hairpin RNA knockdowns are used to model individual loss-of-function events with combined subpopulations reflecting the heterogeneous tumor population.
The model then utilizes a data set of known short hairpin RNA knockdown responses to specific chemotherapeutic agents. The computational simulations can then supply an optimal drug combination for treating the heterogeneous tumor population.
Sources:
- Dagogo-Jack, I. & Shaw, A.T. 2018. Tumour heterogeneity and resistance to cancer therapies, Nature Reviews Clinical Oncology, 15, pp. 81-94.
- Jamal-Hanjani, M. et al. 2017. Tracking the Evolution of Non–Small Cell Lung Cancer, New England Journal of Medicine, 376, pp. 2109-2121.
- Francis, J.M. et al. 2014. EGFR variant heterogeneity in glioblastoma resolved through single-nucleus sequencing, Cancer Discovery, 4, pp. 956-971.
- Meacham, C.E. & Morrison, S.J. 2013. Tumour heterogeneity and cancer cell plasticity, Nature, 501, pp. 328-337.
- Zhao, B et al. 2014. Addressing genetic tumor heterogeneity through computationally predictive combination therapy, Cancer Discovery, 4, pp. 166-174.
Further Reading