An interview with Dr Martin Hemberg, Wellcome Trust Sanger Institute, conducted by April Cashin-Garbutt, MA (Cantab)
What is single-cell RNA sequencing and how can it help define cell types?
RNA sequencing is basically the isolation of RNA from cells and the use of reverse transcriptase to turn the RNA into DNA. You can then use your standard DNA sequencing technologies to quantify the cDNA that you obtained from the reverse transcription reaction. The assay allows you to quantify the amount of RNA in a global- and genome-wide manner.
This technology became popular around ten years ago, but around say two or three years ago, the cost of the technologies, as well as the technologies themselves, were improved to the extent that it has become feasible for many labs to do this type of assay at a resolution of individual cells. This is quite different from what was done previously, where millions of cells were required to get enough RNA.
Before you had single cell, the problem was that having whole tissue, or a whole sample, which was almost certainly heterogeneous, is the equivalent of taking your sample and putting it through a blender. You then have a big, smooth sauce coming out of it and you don't really know what the original components were.
With single cell, you can identify each individual cell that went into your sample. By characterizing individual cells, it's possible to compare them and find cells that look similar and they are the ones that you'll define as specific cell types.
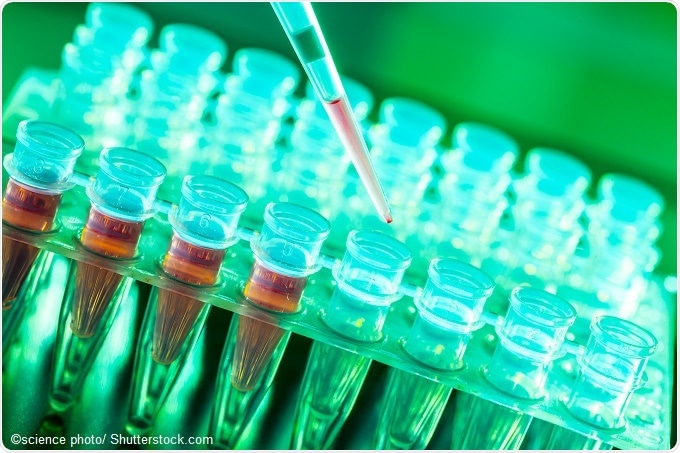
Why has it been difficult to fully exploit single-cell RNA sequence data? What have been the main challenges?
I think the main challenge is that whenever you do an experiment, you'll have some technical noise that introduces some degree of variability. However, we also know there is a lot of variability that is biological. No two cells are identical.
Some of this variability is inherent and biological and, when analyzing the data, the problem is that we don't have a good model of the technical noise. Therefore, deconvoluting the variability and figuring out which part is biological and hence meaningful and interesting, and which parts are technical, and potentially artefacts, has been very hard.
Can you please outline the new analysis tool that has been developed?
From a mathematical point of view, identifying a cell type from a sample of single cell transcriptomes is an unsupervised clustering problem. Those problems are considered difficult, especially if you don't have any training examples that you can use to learn from.
What we have done is used relatively standard machine learning methods for carrying out this task, but we've been able to do careful benchmarking and testing to be able to identify a robust method that seems to perform very well across the large number of different samples and experimental platforms that we've tried it on.
How does this tool overcome previous problems?
Part of it is implied in the name, SC3, which stands for single cell consensus clustering. In order to achieve higher robustness and accuracy, we use a large number of methods instead of a single method and then we combine them and find out what the methods seem to agree on.
We have a large number of methods and each method gets a vote, so to speak, over whether cell A and cell B belong to the same cluster, for example. Then we look at the consensus across all these different methods, which is more robust and more accurate than if you were to rely on a single method.
How accurate did your study show SC3 to be?
It's hard to give a precise number because the accuracy depends on which data sets you're using to benchmark it. For some benchmark data sets, we get what we believe is 100% accuracy. I think the important answer is that we seem to perform at least as well, or better than, all of the other methods that we benchmarked ourselves against.
How user-friendly is the tool?
We think that one of the biggest strengths of the method is that it is more user friendly than anything else out there. It has very nice integration with other packages for single cell analysis.
Before you use the SC3 tool, you need to do some pre-processing of your data to ensure that you have removed poor quality cells. We have seamless integration with the scater package which is one of the more popular tools for that purpose, so that's very helpful.
We also have a very nice graphical user interface, which makes it very easy to get an overview, not just of clustering outcome, where you can get a visual of how good the solution seems to be, but it helps you do further downstream analysis of these clusters.
It's one thing to mathematically identify what appears to be the best clusters, but it is usually much more difficult to figure out what the biological meaning of these clusters is. Our method helps with that by identifying the genes, referred to as marker genes, that are the most important genes for each cluster.
What impact do you think SC3 will have?
We hope that it will greatly facilitate analysis of single cell RNA sequence data. We designed it with experimentalists in mind, so that people who don't have expertise in computational biology, should be able to download and use this tool to analyze their data by themselves.
This specific task of unsupervised clustering of your single cell RNA sequence data is one that is very common; it comes up in most studies that involve single cell RNA sequence data and is an operation that you want to do. We think that this will significantly lower the threshold by allowing people easy access to an accurate and user-friendly tool.
What do you think the future holds for analyzing single-cell RNA data?
I think there is a very great need for additional computational methods and, at this point, the field has reached the stage where it is relatively easy and cheap to do the experimental work. Most labs are able to carry out the experiments themselves, but in order for them to be able to take full advantage and maximize the potential of the data sets, they need to be able to analyze it properly. Currently, all the tools that are needed for this don't exist.
I think this is just the way the field of transcriptomics and genomics works: first, the technologies need to be established and then it takes a few years for the computational community to be able to catch up and produce the set of tools required for the technology to be able to reach its full potential.
So far, most of the use of single cell RNA sequence data has been to support basic biology, but I think there is a lot of hope that it will also be possible to use this in the clinic and that there will be more translational applications.
I think one of the most interesting ones is for cancer. We have an application where it should be possible to characterize tumors, not just based on their mutational profiles, but also based on their transcriptome profiles, which will hopefully allow us to characterize them better and allow for better drugs to be developed.
Where can readers find more information?
About Dr Martin Hemberg
Martin Hemberg is a Group Leader at the Wellcome Trust Sanger Institute, and his research interests are centered around quantitative models of gene expression and gene regulation. He is particularly interested in stochastic models and analysis of single-cell data. His group has developed several software packages, and collaborated with experimentalists to help analyze single-cell data. Another line of research involves analyzing the role of non-coding transcripts and sequences.
In addition to working at the Wellcome Trust Sanger Institute, Martin is also an associate faculty member at the Wellcome Trust/CRUK Gurdon Institute. Martin trained as an undergraduate in engineering physics at Chalmers University of Technology in Gothenburg, Sweden before carrying out graduate studies in applied mathematics under the supervision of Mauricio Barahona at Imperial College London. Subsequently he received post-doctoral training in computational biology in the Kreiman lab at Boston Children's Hospital.
Image credit: Sanger Institute: Genome Research Ltd.