A new deep learning model has been created by a team of researchers at Massachusetts General Hospital (MGH). Early evidence shows that the system is more accurate at predicting a woman’s risk of developing breast cancer than current risk assessment tools.
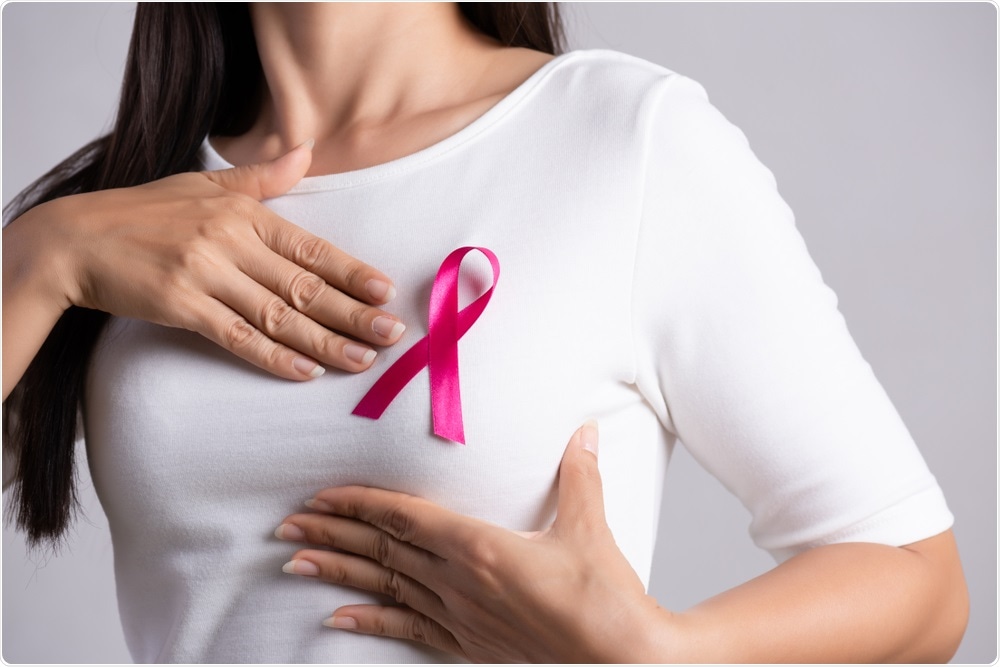
Image Credit: siam.pukkato/Shutterstock.com
Using deep learning to recognize imaging biomarkers in mammograms presents an opportunity to detect the early warning signs of breast cancer in women who may not be considered at-risk, allowing intervention strategies to begin early, potentially improving prognosis.
Deep learning presents a big opportunity for healthcare
Deep learning, a subset of machine learning, has already made big waves in the field of medicine and healthcare. It uses artificial neural networks to solve highly complex problems that are out of the realm of capabilities for traditional computers to solve.
Already, many applications of deep learning have been established in biomedical applications, such as personalized treatment, diagnostics, drug discovery, clinical trials, and management of epidemic outbreaks.
Now, in a study that will be presented at the annual meeting of the Radiological Society of North America (RSNA), a team of US-based scientists demonstrates how deep learning can be used as a risk assessment tool for breast cancer.
Improving traditional risk assessment processes
The team of researchers recognized that current risk assessment tools for breast cancer are limited and are only able to combine data from a limited number of sources such as previous biopsies, family history, and hormonal and reproductive history. Data from the screening mammogram itself is not fully considered by current risk assessment tools, with only breast density data incorporated into these models.
Therefore, traditional assessment tools are missing out on leveraging a rich dataset that could improve risk assessment for breast cancer. Current models are limited in that they can only allocate women into subgroups regarding their level of risk, rather than being precise on an individual level.
The study’s senior author, Constance D. Lehman, M.D., Ph.D., and division chief of breast imaging at MGH highlights the opportunity held in mammogram data to enhance risk assessments to make them personal to the individual.
Every woman's mammogram is unique to her just like her thumbprint. It contains imaging biomarkers that are highly predictive of future cancer risk, but until we had the tools of deep learning, we were not able to extract this information to improve patient care.”
The team, led by breast radiologist at MGH Dr. Leslie Lamb, M.D., M.Sc., developed a new risk cancer assessment tool by creating a deep learning algorithm capable of predicting breast cancer risk. The algorithms were trained on data collected across five MGH breast cancer screening sites in the US.
Overall, 245,753 consecutive 2D digital bilateral screening mammograms were included in the study. The images were collected between 2009 and 2016 from a total of 80,818 patients. The mammograms were split into three groups, with 210,819 images being used to train the deep learning system, 25,644 for testing, and 9,290 for validation.
The accuracy of the newly developed deep learning model in predicting the risk of future breast cancer within five years was analyzed and compared with the currently available traditional risk assessment model, Tyrer-Cuzick version 8. The results showed that the deep learning model was more accurate than the traditional model, achieving a predictive rate of 0.71, significantly higher than the traditional risk model which achieved 0.61.
The new deep learning model can identify a wide range of biomarkers present in mammograms to predicts a woman’s future risk of developing breast cancer at higher accuracies than current models. The model has already been validated in Sweden and Taiwan, and further studies are in the pipeline to validate the model in African-American and minority populations.
The deep learning model could prove incredibly valuable in highlighting at-risk women earlier on, potentially improving their disease outcomes.