A new study from an artificial intelligence (AI) scientist describes a method to predict COVID-19 from a single chest CT shot. The development of such sophisticated tools for the diagnosis of this infection, with protean manifestations, could help reduce the need for invasive testing.
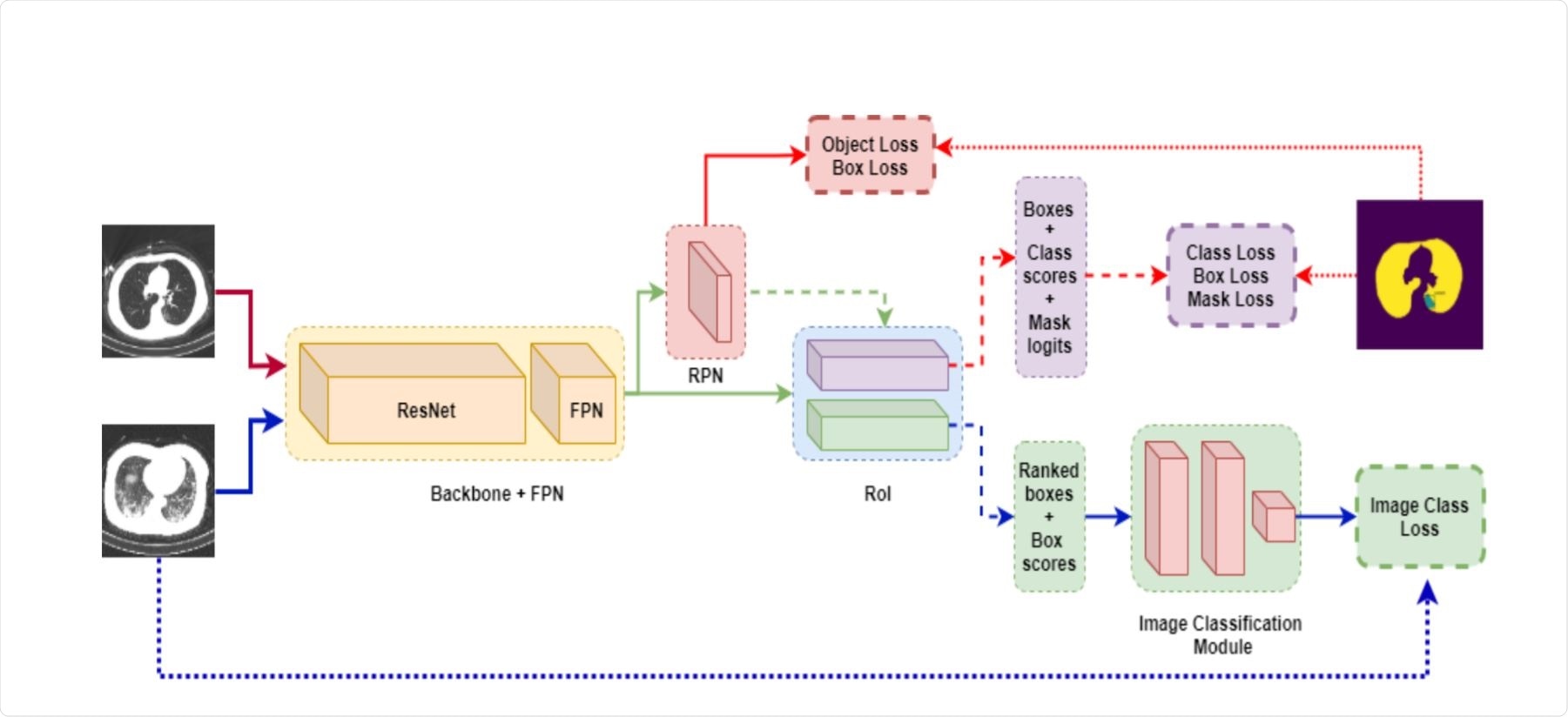
Architecture of the Single Shot Model with two parallel RoI branches Image Credit: medrxiv.org/content/10.1101/2020.12.01.20241786v1.full.pdf

This news article was a review of a preliminary scientific report that had not undergone peer-review at the time of publication. Since its initial publication, the scientific report has now been peer reviewed and accepted for publication in a Scientific Journal. Links to the preliminary and peer-reviewed reports are available in the Sources section at the bottom of this article. View Sources
A definitive diagnosis of COVID-19 is carried out by reverse transcriptase-polymerase chain reaction (RT PCR) testing for viral RNA. Radiography of the chest is also a useful tool.
Early in the pandemic, investigators recommended the use of chest CT scans and it has remained among the most accurate methods of predicting the presence of severe acute respiratory syndrome coronavirus 2 (SARS-CoV-2) infection before the results of the viral RNA test is available. In fact, at some stages of the disease, chest CT may be more sensitive than the PCR test.
Using AI for COVID-19 Diagnosis
Many researchers have turned to the use of AI in the form of deep machine learning models such as convolutional neural networks (convnets) to help interpret chest CT scans for the presence of COVID-19, as well as for the segmentation of the observed lesions.
In most cases, the parameters used to classify the findings make use of feature extractors like ResNet coupled with a logit output that is specific to the problem, such as COVID-19 vs Common Pneumonia.
When it comes to segmenting the lesions, most models employ a semantic segmentation model like U-Net that can make predictions of the masks for different classes at the level of pixels, or enhance the classifier through the addition of deep features to the mask prediction.
Using Image Segmentation/Detection/Classification Together
In the current paper, published as a preprint on the medRxiv* pre-print server in December 2020, the author describes a single-shot architecture. The technique makes use of a recent model, COVID-CT-Mask-Net, which adds a classification feature to models like the cutting-edge detection/segmentation Mask R-CNN.
Mask R-CNN is capable of detecting and segmenting separate objects in images. It can ‘understand’ separate objects but cannot make predictions of image class.
The classification module is based on the ability of the detector to predict bounding boxes and masks, fitted with normalized confidence scores, within the regions of interest (ROIs). The result is that the mask predicts the class of the detected object.
The predictions are converted, batch-wise, into a vector denoting the features that train the classification module. Earlier work by this researcher resulted in several modified versions of this model that are truncated and lightweight but can predict COVID-19 and segment the lesions just as accurately as the base model.
However, this model still uses two stages, first, training the segmentation model (Mask R-CNN) using ground truth masks, and then deriving a classification model that is then trained on the labeled-image data (viz.COVID-19, Common Pneumonia, and Control images).
Simplifying to a One-Step Approach
To avoid this two-phase approach, the paper uses a different solution whereby a single-shot model (SSM) is trained to segment the lesions in the chest CT and predict image class, using a single-short model. The foundation of this technique is the ability of Mask R-CNN to detect the object and to segment its mask.
The researchers tested two possible methods. With one, the segmentation model required pretraining, but the other avoids this step.
For the classification phase, three methods were examined. The segmentation branch of the RoI could be used again to create an image classification batch. Or else, the RoI module could be equipped with a parallel classification branch to create the ROIs for the image classifier. The third way is to allow simultaneous adaptation of both branches, segmentation, and classification, without pretraining.
These are represented as follows by the researchers:
1. pretrained segmentation branch + single branch for segmentation+classification, 2. pretrained segmentation branch + two parallel branches for segmentation+classification
3. training from scratch+two parallel branches for segmentation+classification
The third is the easiest in terms of training since it dispenses with pretraining.
Advantages of Single-Shot Model
The paper claims several benefits to the use of the SSM.
- By using a single-shot model, the researchers can avoid pretraining and allow both the detection and segmentation of the lesion as well as prediction of the diagnosis of COVID-19, Common Pneumonia or Control, from the chest CT.
- The models are less than 10M, allowing for rapid training and evaluation. One CT slice of 512x512 can be processed for both segmentation and image class prediction in 3.8 – 7.1 seconds on a CPU.
- New data can be input easily with simple adaptations to the model.
This fast and reliable model has several novel features and uses truncated models which have been shown to enhance the quality of classification as well as image class prediction. It balances sensitivity for COVID-19 prediction, at 93%, with sensitivity, at ~96.8%.
Thus, this model with only 8.27M parameters is as accurate in image class prediction as to the full-size classifier COVID-CT-Mask-Net [TS20b], with a larger ResNet50+FPN backbone. It is also able to achieve the segmentation precision of Mask R-CNN with ResNet50+FPN.
What are the Implications?
The paper claims to be “the first paper that presents a single-shot solution for both lesion instance segmentation and COVID-19 classification.” This could speed up the prediction of COVID-19 based on chest CT scans, and enhance the already great utility of this tool.
These results exploited the strength of bounding boxes, for lesion localization, and confidence score, for lesion strength. Future studies will focus on the use of other characteristics of the lesions, such as their configuration and features, including the crazy paving appearance, diffuse pattern, and attenuation, which are outside the scope of the bounding boxes used. This could be through the use of masks of the objects to enable a more accurate idea of their shapes.

This news article was a review of a preliminary scientific report that had not undergone peer-review at the time of publication. Since its initial publication, the scientific report has now been peer reviewed and accepted for publication in a Scientific Journal. Links to the preliminary and peer-reviewed reports are available in the Sources section at the bottom of this article. View Sources
Article Revisions
- May 18 2023 - The preprint preliminary research paper that this article was based upon was accepted for publication in a peer-reviewed Scientific Journal. This article was edited accordingly to include a link to the final peer-reviewed paper, now shown in the sources section.