The rapid diagnosis of coronavirus disease 2019 (COVID-19) is a high research priority, with the rapid increase in infections and deaths as winter approaches.
A new study from Athens, Greece, describes the use of a deep learning algorithm that uses chest X-rays to diagnose COVID-19 pneumonia. This preprint, available on the medRxiv* server, presents experimental results that suggest a high-performing approach for COVID-19 diagnostic radiography.
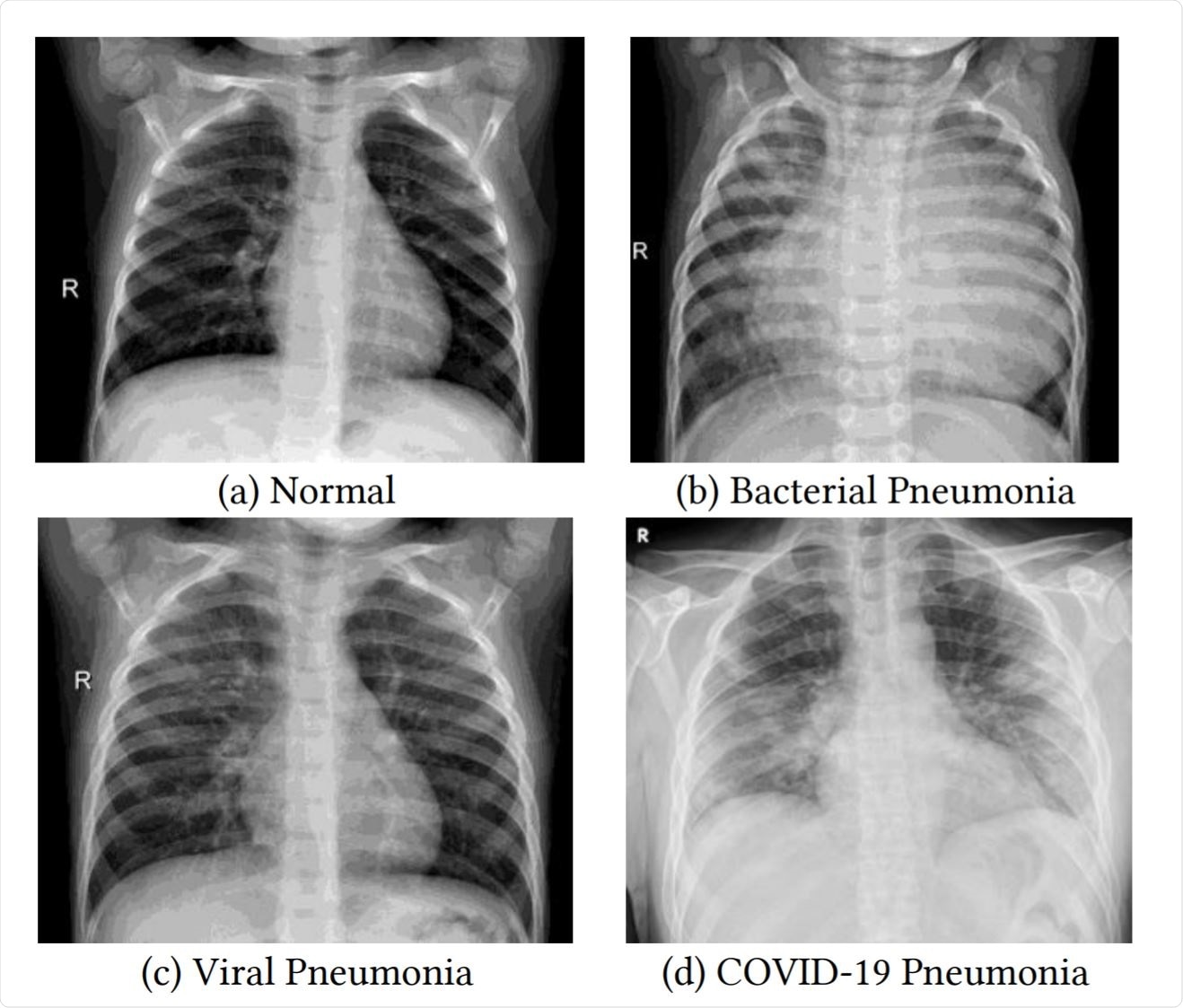
Examples of frontal-view chest X-Ray images from the datasets. Image Credit: https://www.medrxiv.org/content/10.1101/2020.12.14.20248158v1.full.pdf

*Important notice: medRxiv publishes preliminary scientific reports that are not peer-reviewed and, therefore, should not be regarded as conclusive, guide clinical practice/health-related behavior, or treated as established information.
Chest X-rays in COVID-19
COVID-19 affects the lungs, primarily, although it can damage multiple organs in severe or critical disease. Thus, chest X-rays play a large part in the diagnosis of COVID-19 pneumonia. Important findings in this condition include increased whiteness of the lungs, proportional to the severity of the disease.
The typical radiographic appearance of the lungs in COVID-19 pneumonia is of ground-glass opacities and sometimes of linear opacities in the lung periphery, with the lung markings being somewhat obscured. As the condition becomes more severe, these markings become invisible, or ‘whited-out’.
The detection of lung consolidation in combination with laboratory and clinical assessments could be useful in diagnosing this disease early and reliably.
Deep learning in COVID-19 diagnosis
Deep learning has been extensively employed in medical imaging over the past decade, and intensive efforts have enabled it to deliver highly accurate reports in many conditions. This includes Alzheimer’s dementia, diabetic retinopathy, and brain hemorrhage, among others.
In fact, its use has allowed the diagnostic standard of the machine to exceed that of medical professionals, as shown in a study where a deep learning model was able to detect 11/14 chest diseases with greater or comparable accuracy relative to human radiologists. The basic task here is to classify the computer image.
The researchers in the current study used convolutional neural networks (CNNs) to investigate chest X-rays in COVID-19 patients, to classify them as COVID-19 or non-COVID-19 images.
Transfer learning
The fundamental issue with all such deep learning algorithms is the necessity for large datasets to train the classifiers and to assess their function. Since there are only a relatively small number of COVID-19 chest X-rays, the researchers chose to use transfer learning for training the deep CNNs.
“The idea of transfer learning lies in the fact that, in machine learning, we can utilize knowledge gained from one problem A and then apply it to another related problem B.”
For example, using the knowledge of how cats are detected to learn to detect dogs is transfer learning, based on the presence of similar low-level image features.
By using this, not only did they not have to initiate training from the very start, but they overcame the paucity of training data by using knowledge pulled from other tasks. “Through transfer learning, we can create accurate deep models on small datasets, by leveraging existing networks, already trained on large datasets.”
Predicting the diagnosis
The researchers aimed to display the effectiveness of cutting-edge CNNs trained in this manner in diagnosing COVID-19 pneumonia using chest X-rays taken from the front.
The models would then be assessed for their ability to distinguish COVID-19 pneumonia from bacterial pneumonia. They used large databases of chest radiographs showing bacterial and viral pneumonia. They also used knowledge from already trained networks based on very large datasets of images, like the ResNet-50.
They constructed a simple CNN, which was first trained to classify normal, bacterial pneumonia, and viral pneumonia. Using the same design, two other CNNs were trained, one from scratch and the other using transfer learning. Lastly, a ResNet-50 network was trained using generic knowledge gained on ImageNet.
They evaluated the results in terms of four performance parameters, namely, predictive correctness; the percentage of correct recall among total relevant images; the relative proportion of correct predictions to total predictions, and F1-score, the harmonic mean of recall and score.
Conclusion
They found that the third model, pre-trained on ImageNet, had ~98% of correct prediction and recall ability, because of the more complex design and the transfer learning ability. Overall, the error rate of the deep learning model shown here fell to half.
“The results of this study indicate that the transfer learning approach outperforms the performance obtained without transfer learning, for the COVID-19 classification task.”

*Important notice: medRxiv publishes preliminary scientific reports that are not peer-reviewed and, therefore, should not be regarded as conclusive, guide clinical practice/health-related behavior, or treated as established information.