A new study published on the preprint server medRxiv* discusses the use of multiple biomarkers taken over the course of hospitalization in coronavirus disease 2019 (COVID-19) patients to create a prognostic model for the risk of mortality. This differs from the temporally restricted biomarkers that have been analyzed in many studies so far and may provide a foundation for the development of more accurate and representative models in the future.
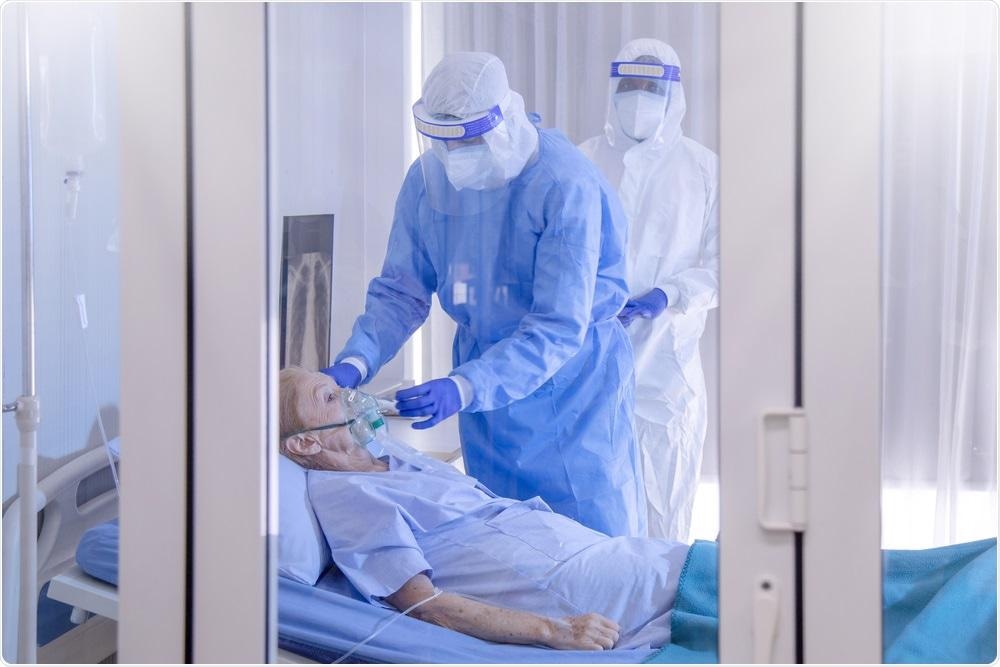
Study: Using Patient Biomarker Time Series to Determine Mortality Risk In Hospitalised Covid-19 Patients: A Comparative Analysis Across Two New York Hospitals. Image Credit: C.Lotongkum / Shutterstock.com

This news article was a review of a preliminary scientific report that had not undergone peer-review at the time of publication. Since its initial publication, the scientific report has now been peer reviewed and accepted for publication in a Scientific Journal. Links to the preliminary and peer-reviewed reports are available in the Sources section at the bottom of this article. View Sources
Background
The continuing rise in infections with the severe acute respiratory syndrome coronavirus 2 (SARS-CoV-2) has driven hospitalizations to breaking points across countries. This remains true as new variants continue to emerge and threaten the success of vaccination programs. To manage medical care better, it is essential to be able to predict the risk of death, so as use resources optimally when they are scarce.
Earlier studies have proposed a number of prognostic factors including ethnic, socioeconomic, and comorbid factors. Many of these apply only in a narrow setting.
Even when multiple variables are used, it remains probable that many unknown but important variables exert confounding effects, thus making these models difficult to apply beyond the sample tested.
The current study used biomarker measurements plotted throughout the course of hospitalization for over 1,500 patients in two New York Hospitals including the State University of New York (SUNY) Downstate Health Sciences University and Maimonides Medical Center. The use of these time-dependent biomarker changes could help predict the risk of adverse outcomes.
The researchers had already created a Bayesian Markov to measure the daily mortality risk and how biomarker changes affect this risk. This was fitted to data from SUNY. In the current study, the model is fit to patient data from Maimonides Medical Center (MMC) to provide external validation and evaluate how far dynamic changes in biomarkers over the course of the disease are useful in prognostic models.
Study findings
The two patient cohorts had different demographic and comorbidity characteristics. Most SUNY patients were Black, whereas most MMC patients were White.
Diabetes dominated the comorbidity list in SUNY patients, whereas coronary artery disease and congestive heart failure were the most commonly present comorbidities in MMC patients, with differences in the percentage of patients who showed multiple health conditions. When the admission variables were compared, the odds of death associated with both diagnostic factors and laboratory test results were similar for both hospitals.
Changes in laboratory values were then considered for both hospitals, thereby showing that there were significant differences between patients, regardless of the outcome. The temporal patterns for each hospital cohort showed good correspondence for most variables.
Comparing univariate mortality ORs across the hospitals. The two panels compare ORs associated with demographic and disease history variables (panel A) and initial laboratory test values (panel B). Points show the posterior median ORs; the whiskers display the 25% and 75% posterior quantiles. The orange dashed lines show the OR = 1 cases; the dashed black lines indicate equality in the ORs across the two hospitals. The blue line shows least squares regression lines using the posterior median ORs.
At 15 days from admission, only a few patients were still in the hospital. Furthermore, the percentage change in laboratory values showed a good correlation with the outcome, whether death or discharge.
The researchers looked at the risk of daily mortality for each biomarker across both hospitals. This showed that each marker was positively correlated with the estimated risk for both cohorts. The majority of the risk estimates pointed in the same direction, while less than half had an odds ratio (OR) above or below one in both sets.
With the rest of the variables, the risk for patients in the MMC cohort tended to be lower than for SUNY. The highest increases in risk were seen with an increase in the red cell size, reduced counts of lymphocytes and platelets, and decreased carbon dioxide levels over the length of the hospital stay.
The investigators then sought to identify the strongest predictive factors using the odds ratios (ORs) from the Markov model and comparing them with the full set of dynamic biomarker measurements for both hospitals. This showed much less correlation between the two sets of estimated ORs.
This result was due to the difference in the ORs based on just two parameters, the mean corpuscular hemoglobin concentration (MCHC), and the mean corpuscular volume (MCV), which showed a significant difference between the two cohorts. The removal of these two parameters caused a positive correlation between the estimates to appear.
Are these predictions of mortality risk generalizable? To answer this question, the scientists looked at predictive accuracy across both hospitals.
This showed that the larger number of MMC patients increased the accuracy when this set of data was used as compared to SUNY data. When dynamic changes in laboratory measures were factored in, the accuracy went up markedly as compared to the use of only admission data.
Using as many post-admission variables as possible to evaluate the predictive power and pooling predictions across both sets, the researchers concluded that this provided a more sensitive prediction for discharge, but less so for mortality risk, at 79% versus 61%, respectively. When the data was used to predict the outcome in another hospital rather than the same one, the predictive accuracy was lower; however, the difference was not very large.
This suggests that the model performs relatively well when used in a different setting.
Using only data on patients who eventually failed to survive, the investigators explored the factors influencing the accuracy of the predictions. This led to the identification of time since COVID-19-related admission, history of coronary artery disease (CAD), and non-Caucasian ethnicity as the three most important factors affecting accuracy.
When linear regression was performed, they found that only a history of CAD improved the median predictive accuracy.
Implications
The researchers showed that a number of biomarkers whose measures show changes over the hospital course of a COVID-19 patient are useful prognostic factors by externally validating their performance. Several single markers were measured at the single point of admission to associate with a higher mortality risk for both cohorts, notably reduced eosinophils, lymphocytes, and platelets, with high blood urea levels.
Conversely, some variables that contribute little if only their admission values are used were identified, though their dynamic changes do have significant predictive accuracy. These include the higher mean corpuscular volume (MCV) and the lower mean corpuscular hemoglobin concentration (MCHC) over time. Importantly, this was not validated in the other study cohort.
These findings demonstrate that dynamic biomarkers are potentially very important in assessing patient mortality risk in quantitative terms, as well as underlining the need to validate all such predictive models externally.
The Bayesian Markov model used here was more accurate in predicting discharge than death, thereby indicating that more work must be conducted to develop an acceptable prognostic model for hospitalized COVID-19 patients. Some factors that are important for this purpose, but are measured only at presentation, need to be evaluated dynamically.
These include markers of inflammation, myocardial injury, acute respiratory distress syndrome (ARDS), and coagulopathy, as well as chemokines and cytokines, as these all indicate progressive disease, which is an important factor in mortality risk.
The changes in some of these biomarkers following treatment were also not factored in. For instance, mechanical ventilation could affect carbon dioxide (CO2) values and some anti-inflammatory therapies such as tocilizumab or corticosteroids could drive down inflammatory markers.
Again, the effects of novel variants and the phase of the pandemic need to be included, as well as the type of treatment setting, which could improve survival odds over time.
The model used in the current study provided reasonably good estimates in both cohorts. This does not mean that it should be adopted for use in a clinical setting, as it has to be trained across a much larger set of patients, evaluated in much larger datasets, and over many different settings.
Following such validation, the simplicity of use, its cost-effectiveness, superiority, if any, to existing models, and the eventual benefit to the patient in terms of improved outcome, must be shown before it can be widely adopted.

This news article was a review of a preliminary scientific report that had not undergone peer-review at the time of publication. Since its initial publication, the scientific report has now been peer reviewed and accepted for publication in a Scientific Journal. Links to the preliminary and peer-reviewed reports are available in the Sources section at the bottom of this article. View Sources
Journal references:
- Preliminary scientific report.
Lambert, B., Stopard, I. J., Momeni-Boroujeni, A., et al. (2021). Using Patient Biomarker Time Series to Determine Mortality Risk In Hospitalised Covid-19 Patients: A Comparative Analysis Across Two New York Hospitals. medRxiv. doi:10.1101/2021.11.12.21266248. https://www.medrxiv.org/content/10.1101/2021.11.12.21266248v1.
- Peer reviewed and published scientific report.
Lambert, Ben, Isaac J. Stopard, Amir Momeni-Boroujeni, Rachelle Mendoza, and Alejandro Zuretti. 2022. “Using Patient Biomarker Time Series to Determine Mortality Risk in Hospitalised COVID-19 Patients: A Comparative Analysis across Two New York Hospitals.” Edited by Bryan C Daniels. PLOS ONE 17 (8): e0272442. https://doi.org/10.1371/journal.pone.0272442. https://journals.plos.org/plosone/article?id=10.1371/journal.pone.0272442.
Article Revisions
- May 9 2023 - The preprint preliminary research paper that this article was based upon was accepted for publication in a peer-reviewed Scientific Journal. This article was edited accordingly to include a link to the final peer-reviewed paper, now shown in the sources section.