In a recent study published on the medRxiv* preprint server, researchers estimate the probabilities of hospitalization and mortality from coronavirus disease 2019 (COVID-19) according to individual risk factors and the number of BNT162b2 messenger ribonucleic acid (mRNA) vaccine doses received.
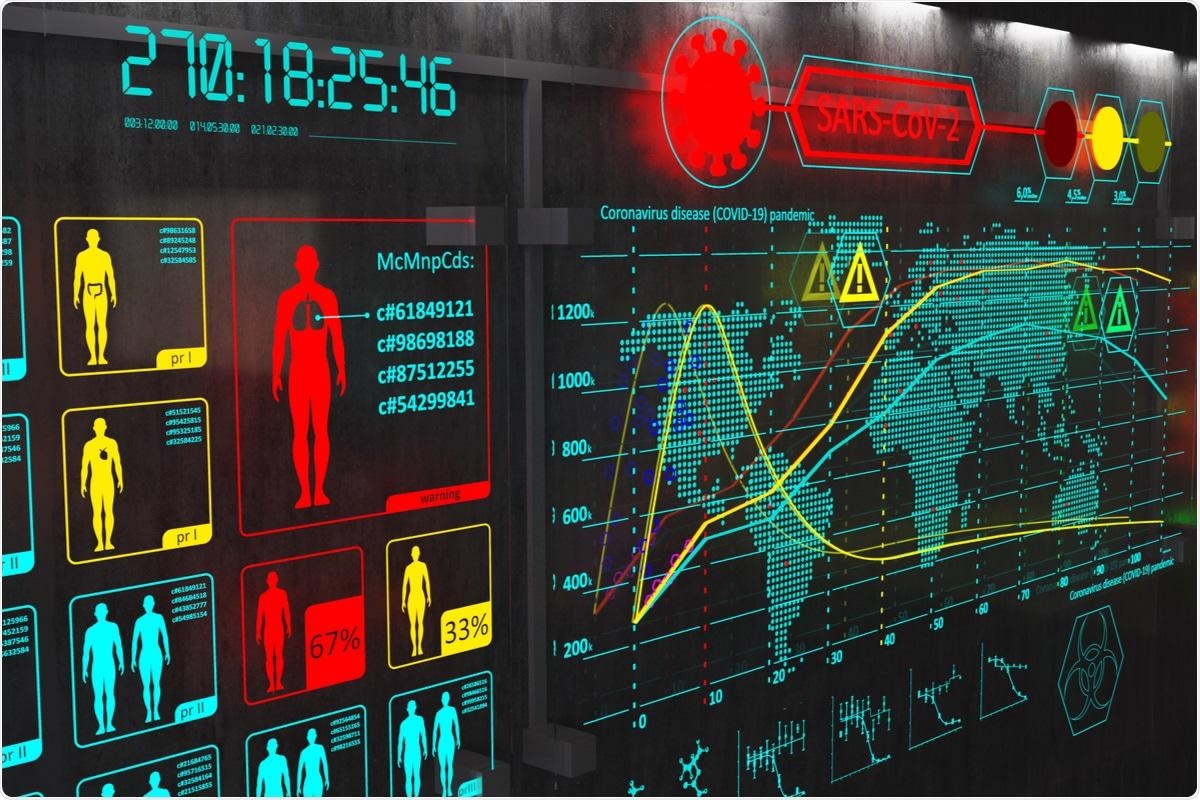
Study: COVID-19 severity prediction based on patient risk factors and number of vaccines received. Image Credit: Corpulentti / Shutterstock.com

This news article was a review of a preliminary scientific report that had not undergone peer-review at the time of publication. Since its initial publication, the scientific report has now been peer reviewed and accepted for publication in a Scientific Journal. Links to the preliminary and peer-reviewed reports are available in the Sources section at the bottom of this article. View Sources
Given the limited supply of vaccines and medications for COVID-19 in several countries, a model that can estimate the need for hospitalization and mortality for any severe acute respiratory syndrome coronavirus 2 (SARS-CoV-2)-infected individual could help save lives and reduce the burden on healthcare facilities. This type of model will also help provide early treatment for COVID-19, especially for high-risk individuals. Israel presents a unique opportunity for such assessments due to extreme variation in vaccine uptake among its population.
About the study
The current study was retrospectively conducted from April 1, 2020, to November 30, 2021, on electronic health records of 101,034 SARS-CoV-2-infected individuals from the Leumit Health Services (LHS) in Israel. Of these records, 1,752 patients only required hospitalization and 393 patients died during hospitalization or within 30 days of SARS-CoV-2 infection.
The researchers used multivariable logistic regression models based on the number of vaccine doses received and only a few risk factors. Importantly, the authors of this study deliberately limited the analysis to a few but the most significant COVID-19 severity risk factors such as hypertension, chronic obstructive pulmonary disease (COPD), and malignancy (solid or hematologic) to make these models simpler, interpretable, and deployable in all types of health provider information systems.
The current study also provided regression coefficients and odds ratio (OR) for each risk factor to enable the calculation of risk estimates for any COVID-19-infected individual using a simple formula.
Study findings
The hospitalization risk model predicted that an increase in age was significantly associated with the risk of COVID-19-related hospitalization. Each year increased the odds of hospitalization by a multiplicative factor of 1.058, which implied that a 60-year-old was ten times more likely to get hospitalized due to COVID-19 as compared to a 20-year-old having similar risk factors.
Receiver Operating Curve for hospitalization risk model The ROC curve shows the sensitivity and the specificity of the hospitalization model as its discrimination threshold is varied. With a threshold of 8.71% for risk, 50% of the COVID-19 episodes necessitating hospitalization can be identified (sensitivity=50%), and specificity is 95.3% (false-positive rate=4.7%); with a risk threshold of 2.39%, sensitivity is 80% and specificity is 82.2% (false-positive rate=7.8%); with a risk threshold of 1.14%, sensitivity is 90% and specificity is 70.2% (false-positive rate=30.8%).
The odds of hospitalization in females were reduced by 34% compared to males. While obesity, diabetes mellitus, and impaired kidney function gradually increased the hospitalization risk, the hospitalization risk increased substantially due to hypertension and cancer. With an area under the curve (AUC) of 0.889, the performance of the hospitalization risk model was remarkably accurate.
The mortality risk due to COVID-19 was 50% less in females than males. Again, advanced age was more strongly associated with increased mortality risk.
However, each year of age increased the odds for death by a factor of 1.103, which implied that a 60-year-old patient was 51 times more likely to die of the disease compared to a 20-year-old having other similar risk factors. In addition, extremely obese and underweight patients were at an increased risk of COVID-19-related death. The mortality risk model estimated an AUC of 0.967 and predicted 50%, 80%, and 90% of death events, with specificities of 98.6%, 94.9%, and 91.2%, respectively.
The current study also predicted the outcome for newly infected individuals with remarkable accuracy. Furthermore, the findings suggested that although breakthrough infections could occur, three vaccination doses significantly reduced the risk of hospitalization by 66% (OR=0.336) and death by 78% (OR=0.220).
Conclusions
The model proposed in the current study had several benefits, as it did not include country-specific demographic variables and was generalizable to other populations. The model also used a limited number of input variables to recognize clinical factors associated with COVID-19 severity and yet generated highly accurate AUCs.
Importantly, these mortality and hospitalization risk estimates could be given at the time of COVID-19 diagnosis. This would subsequently allow for the identification of patients at the highest risk so that they could be aligned for treatment early in the infection.
The estimations provided by this model enabled rapid identification of individuals and population segments at high risk for hospitalization and death when infected. Furthermore, they were also found to prove useful in prioritizing patients who needed booster vaccination or COVID-19 medications, which are sparsely available.
The current study also demonstrated that the immune response elicited by the COVID-19 vaccine has a lasting effect on hospitalization and mortality risk as compared to its effect on the risk of symptomatic infection following exposure to the SARS-CoV-2.
The web-based calculator based on the study models and the fully described approach to run and adapt these models is publically available online at http://covidest.web.app.

This news article was a review of a preliminary scientific report that had not undergone peer-review at the time of publication. Since its initial publication, the scientific report has now been peer reviewed and accepted for publication in a Scientific Journal. Links to the preliminary and peer-reviewed reports are available in the Sources section at the bottom of this article. View Sources
Journal references:
- Preliminary scientific report.
Israel, A., Schaffer, A. A., Merzon, E., et al. (2021). COVID-19 severity prediction based on patient risk factors and number of vaccines received. medRxiv. doi:10.1101/2021.12.31.21268575. https://www.medrxiv.org/content/10.1101/2021.12.31.21268575v1.
- Peer reviewed and published scientific report.
Israel, Ariel, Alejandro A. Schäffer, Eugene Merzon, Ilan Green, Eli Magen, Avivit Golan-Cohen, Shlomo Vinker, and Eytan Ruppin. 2022. “A Calculator for COVID-19 Severity Prediction Based on Patient Risk Factors and Number of Vaccines Received.” Microorganisms 10 (6): 1238. https://doi.org/10.3390/microorganisms10061238. https://www.mdpi.com/2076-2607/10/6/1238.
Article Revisions
- May 10 2023 - The preprint preliminary research paper that this article was based upon was accepted for publication in a peer-reviewed Scientific Journal. This article was edited accordingly to include a link to the final peer-reviewed paper, now shown in the sources section.