De novo protein structure prediction uses algorithms to determine the tertiary structure of a protein based on it's primary sequence.
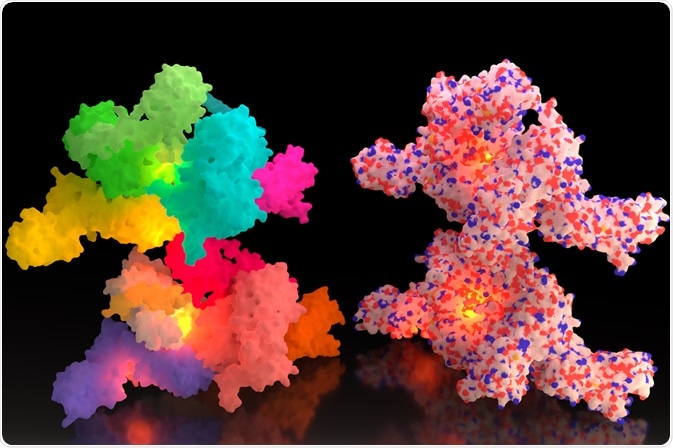
Oleg Nikonov | Shutterstock
The development of successful algorithms means that it is now possible to predict the folds of small, single-domain proteins to an accurate degree, at atomic resolution.
Computational-based Rosetta method
De novo methods require a large amount of computational power to solve relatively small proteins. De novo prediction is differentiated from other forms by the absence of a starting template.
At present, the computational power and algorithms available are not complex enough to be able to predict the structure of larger proteins. This method is therefore restricted to smaller proteins.
The Rosetta method is a popular technique for de novo protein structure prediction.This technique is based on the observation that in some organisms, interacting proteins are encoded by separate genes, whereas in other organisms, their orthologues are fused into a single polypeptide chain. The the structure of the protein is determined by looking a fragment of sequence with kinetic and thermodynamic constraints.
Predicting protein function from structure
De novo function prediction requires amino acids from the protein of interest to be spatially organized. This process is guided by several functions and sequence-dependent biases and restrictions to generate a series of possible candidate structures called ‘Decoys’. From these, the most native-like structures are selected using scoring functions.
There are two principal scoring functions: 1) physics-based functions which use mathematical means of modeling physics-based molecular interactions, and 2) knowledge-based functions which are based on statistical models that define the properties of the native-like conformation.
Resolving Levinthal's paradox to predict protein structure
The main bottleneck associated with de novo methods is the number of possible conformations. In theory, a single amino acid can occupy an abundance of geometrically possible structures. For example, a protein of 100 amino acids in length where each amino acid can adopt only 3 possible conformations would have 3100 = 5 x 1047 possible conformations.
If the time taken to switch between each of these conformations is 10-13, then the time required to test all conformations would be 5 x 1034 seconds, or 1027 years. The age of the universe is 1010, and so the folding of a small protein should, in theory, require almost three universe lifetimes.
Experimentally however, a protein folds within fractions of a second. This disconnect between the theorised folding of a protein and the experimentally determined time-frame is called the Levinthal’s Paradox’, named after the molecular biologist who initially proposed it.
A resolution to Levinthal’s Paradox is offered by the research that has proven that proteins do not follow a random sampling of the conformational space to arrive at their native structure. Instead, proteins organize themselves as individual sections or clusters, based on local forces which cause pulling and repulsion. This causes neighboring clusters form, and the process repeats.
As one follows the path of protein folding the possible conformations become fewer as the protein organizes and moves towards increasing stability.
Through many studies, researchers now understand general rules for how proteins fold and the speed at which they are able to fold. However, in practice, it is difficult to predict how a protein will fold. Therefore, de novo methods begin by specifying the attractive and repulsive forces for each amino acid, then calculate a structure by solving the equations to determine the energy of this structure. The process is repeated until the conformation with the lowest possible energy is obtained.
The future of accurate protein structure prediction
The accuracy of the predictions depends on the resolution and the most stable and native conformation. Modelers then must balance the increase in resolution by mapping the positions of all atoms and the expense of limiting the amount of sampling carried out by an algorithm.
As such, the issue in protein folding is limited by the computational power available; once supercomputers have been developed that are able to carry out complex and extensive protein-folding simulations, the protein folding prediction problem remains to be solved.
Further Reading