As an example of precision public health, a new study published in the preprint server medRxiv* in July 2020 reports the successful detection of a potential new local COVID-19 case cluster, through the innovative adaptation of existing case tracing technology.
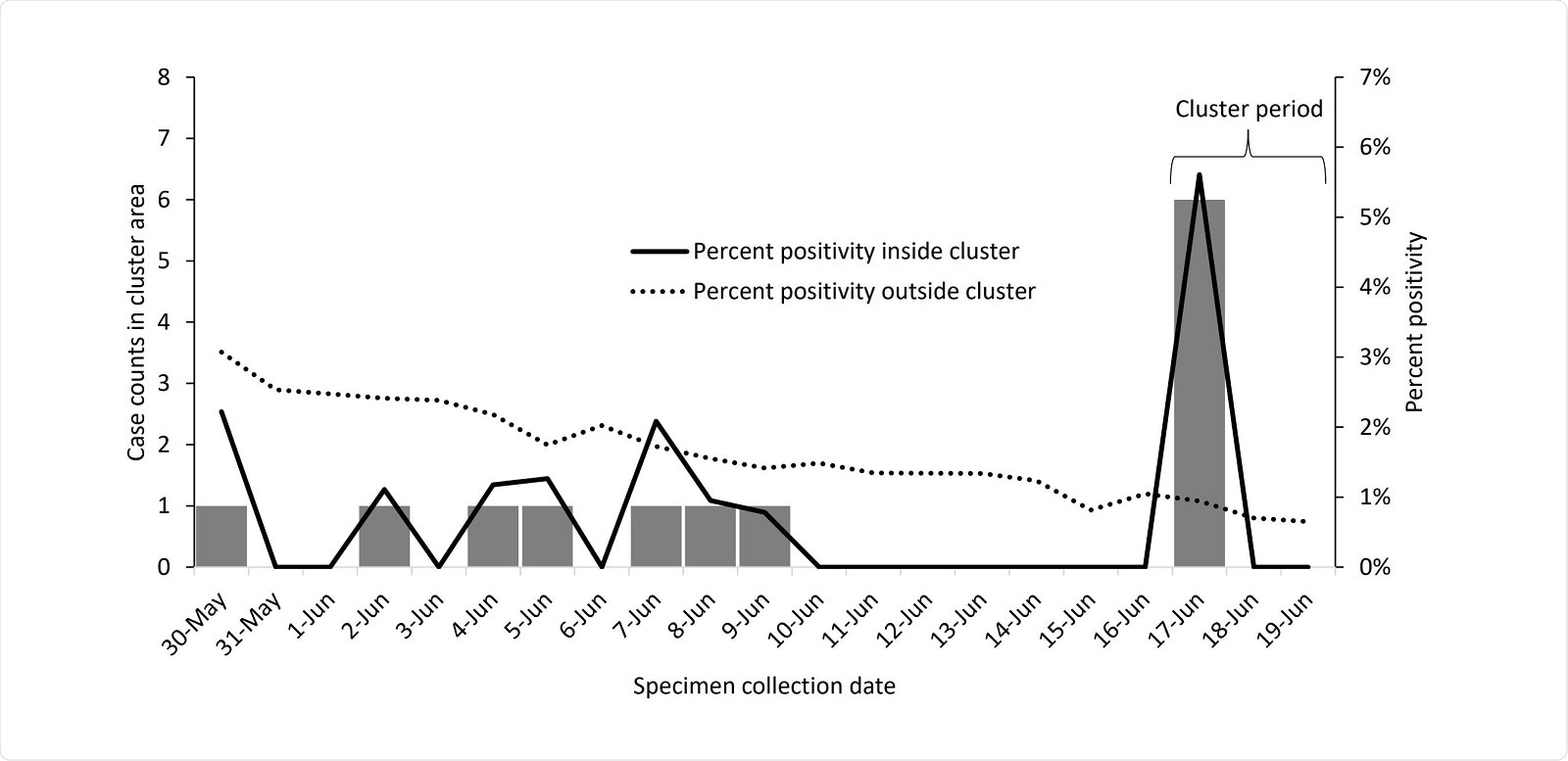
Cluster case counts and SARS-CoV-2 percent positivity inside and outside cluster area for cluster detected on June 22, 2020 in 5 census tracts in New York City, in which patients reported common attendance at a social gathering

This news article was a review of a preliminary scientific report that had not undergone peer-review at the time of publication. Since its initial publication, the scientific report has now been peer reviewed and accepted for publication in a Scientific Journal. Links to the preliminary and peer-reviewed reports are available in the Sources section at the bottom of this article. View Sources
The researchers used the free SaTScan software developed by Martin Kulldorff to generate daily analyses of case clusters by geographical location and time frame.
Spatial and temporal case distribution
Public health officials find it useful to be able to locate new cases by date and location, as such detailed data helps to monitor the spread of the disease and intervene in a targeted manner to contain outbreaks early.
This has been attempted using public data, using daily and county-based frameworks across the whole of the USA. Space-based clusters have also been traced using ZIP codes within New York City (NYC).
Case-only analysis fails as a monitoring strategy
The NYC Department of Health and Mental Hygiene (DOHMH) has put into routine use the case-only space-time permutation scan feature of the free SaTScan software, as part of its surveillance program aimed at picking up new outbreaks of reportable diseases, including COVID-19, Legionnaires’ disease and salmonella outbreaks.
However, in the case of COVID-19, the disparity in testing by both location and time parameters makes the case-only analysis unsuitable as a monitoring strategy. This is because any difference in testing rates will show up as a difference in disease rates as well.
Moreover, the researchers wanted to detect potential hotspots during the epidemic stage rather than only after the outbreak had stabilized or died down. Therefore, they adopted a new approach that could identify locations where the number of cases was going up, or showing a slower rate of fall compared to other parts of the city.
The study: community clusters
The researchers focused on using the software to set up a surveillance system, whereby community-based clusters could be detected, which had higher percent test positivity for SARS-CoV-2 at almost real-time conditions and with the location narrowed down to defined census tracts in NYC.
They adjusted for variability in testing. The system came into use from June 11, 2020, with the first common-exposure cluster of COVID-19 being detected on June 22.
The data was gathered from the DOHMH, which receives all the COVID-19 testing results from New York State residents via the New York State Electronic Clinical Laboratory Reporting System (ECLRS). These laboratory data includes the date of collection of the sample, and the residential address with other demographic characteristics of the patient. Many patients are interviewed to obtain patient symptoms and the date of onset.
Space-time scan statistic identifies clusters
The system uses a space-time scan statistic in the form of a cylindrical scan. The base of the cylinder occupies a geographical area, with the height depicting the time, therefore, enabling it to scan both dimensions over varying periods and areas. With each position, the number of cases in the cylinder is assessed relative to the expected count of cases, with the null hypothesis being no clusters.
A cluster is identified as most likely when the cylinder position shows the highest likelihood, and it is evaluated for significance after adjusting for the intrinsic presence of multiple tests because of the many cylinder positions assessed.
Daily analysis is required for the prospective detection of new hotspots. However, due to this practice, recurrence intervals were used in place of p-values to compensate for the multiple occurrences of the same test.
To facilitate the aim of detecting emerging clusters of infection, The researchers also adjusted their model for geographical alterations that remained the same over time. Time trends in percent positivity across NYC were also adjusted, since local rather than citywide outbreaks were the focus.
Detection of Emerging Hotspot
The software detected 28 clusters from June 11 to June 30. They defined the upper limit for a spatial cluster at half the number of individuals tested, but the clusters were still found to be within a small area of median radius approximately 0.7 km. During the study period, the percent positivity for the virus across the whole city was 1.3%, with a median percent positivity per cluster of 4.7%.
Interestingly, more than half of the individuals in 10/28 clusters were in the 18-34 age group, suggesting that this group showed less concern for social distancing. Over this period, citywide case counts fell. However, on June 22, a six-patient cluster of median age 40 was detected within a 0.6 km radius.
All patients in the cluster had been tested on June 17, and interviews showed that two of the patients had been at the same gathering, and social distancing had not been in practice. The DOHMH then initiated containment measures, such as testing and tracing of contacts, meeting the community members, and reinforcing the need to self-isolate and quarantine after possible exposure by health education.
Application and future efforts
The current study shows that automated analyses of infection clusters by space and time are capable of finding newly emerging small clusters in limited areas, promoting containment efforts in NYC. The researchers found that one in three clusters was dominated by young adults, which indicates that this age group must be targeted for the promotion of social distancing.
Secondly, though much effort is required to find clusters and only one cluster could be traced to a common origin for at least some of the infections, it is essential to detect local spread so that public health teams can narrow down the required field of interventions.
During June, we made several adjustments to improve signal prioritization, including increasing the minimum temporal cluster size from 2 to 3 days and increasing the minimum number of cases in clusters from 2 to 5 cases.”
They plan to further develop the system so as to make it easy for other health departments. They conclude, “Such local targeted, place-based approaches are necessary to minimize further transmission and to better protect people at high risk for severe illness, including older adults and people with underlying health conditions.”

This news article was a review of a preliminary scientific report that had not undergone peer-review at the time of publication. Since its initial publication, the scientific report has now been peer reviewed and accepted for publication in a Scientific Journal. Links to the preliminary and peer-reviewed reports are available in the Sources section at the bottom of this article. View Sources
Journal references:
- Preliminary scientific report.
Greene, S. K. et al. (2020). Detecting Emerging COVID-19 Community Outbreaks at High Spatiotemporal Resolution - New York City, June 2020. medRxiv preprint. doi: https://doi.org/10.1101/2020.07.18.20156901. https://www.medrxiv.org/content/10.1101/2020.07.18.20156901v1
- Peer reviewed and published scientific report.
Greene, Sharon K., Eric R. Peterson, Dominique Balan, Lucretia Jones, Gretchen M. Culp, Annie D. Fine, and Martin Kulldorff. 2021. “Detecting COVID-19 Clusters at High Spatiotemporal Resolution, New York City, New York, USA, June–July 2020.” Emerging Infectious Diseases 27 (5). https://doi.org/10.3201/eid2705.203583. https://wwwnc.cdc.gov/eid/article/27/5/20-3583_article.
Article Revisions
- Mar 23 2023 - The preprint preliminary research paper that this article was based upon was accepted for publication in a peer-reviewed Scientific Journal. This article was edited accordingly to include a link to the final peer-reviewed paper, now shown in the sources section.