In a recent study posted to the medRxiv* preprint server, a team of researchers predicts the evolution of coronavirus disease 2019 (COVID-19) mortality rates across countries using a biological science-guided machine learning-based approach.
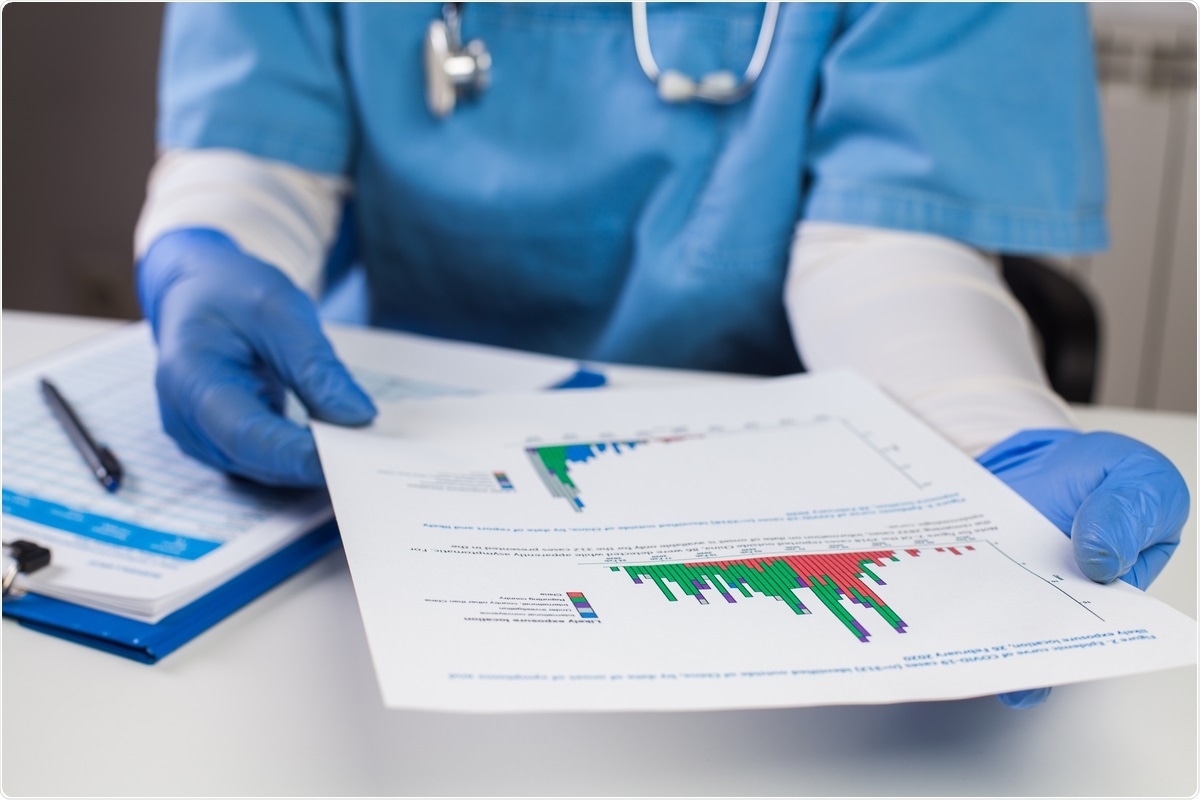
Study: Understanding Evolution of COVID-19 Driven Mortality Rate. Image Credit: Cryptographer / Shutterstock.com

*Important notice: medRxiv publishes preliminary scientific reports that are not peer-reviewed and, therefore, should not be regarded as conclusive, guide clinical practice/health-related behavior, or treated as established information.
In previous studies, several factors including the prevalence of autoimmune diseases, hazardous air pollutants such as fine particulate matter (PM2.5), ozone, and nitrogen dioxide, as well as dietary effects have been associated with an increased risk of COVID-19 related fatality. However, a study exploring multiple factors affecting COVID-19 mortality rates individually and interdependently is needed.
About the study
In the current study, researchers used a novel Fast Fourier Transformation (FFT) driven machine-learning algorithm to analyze the publically available data of COVID-19 mortality rate from 141 countries. They assessed the impact of eight biological and socioeconomic factors such as alcohol consumption, diabetes prevalence, gross domestic product (GDP) per capita, the global health index, meat consumption, milk consumption, PM2.5, and population density on the COVID-19 mortality rates.
The 141 countries assessed in the current study varied in size and population and spanned across five continents. Moreover, the machine learning model was trained in 121 of the 141 countries’ data, where the remaining 20 countries served as the validation set.
An FFT approach to solving an epidemiological model is novel and yielded the Fourier coefficients (an’ s and bn’ s) after testing evolutionary mortality rates of different countries. From the training data set, Fourier coefficients of the validation dataset were predicted, which underwent an Inverse Fast Fourier Transformation (IFFT) to predict the mortality rate. The researchers also compared these predicted mortality rates with actual COVID-19 mortality rates to evaluate the efficacy of the FFT approach.
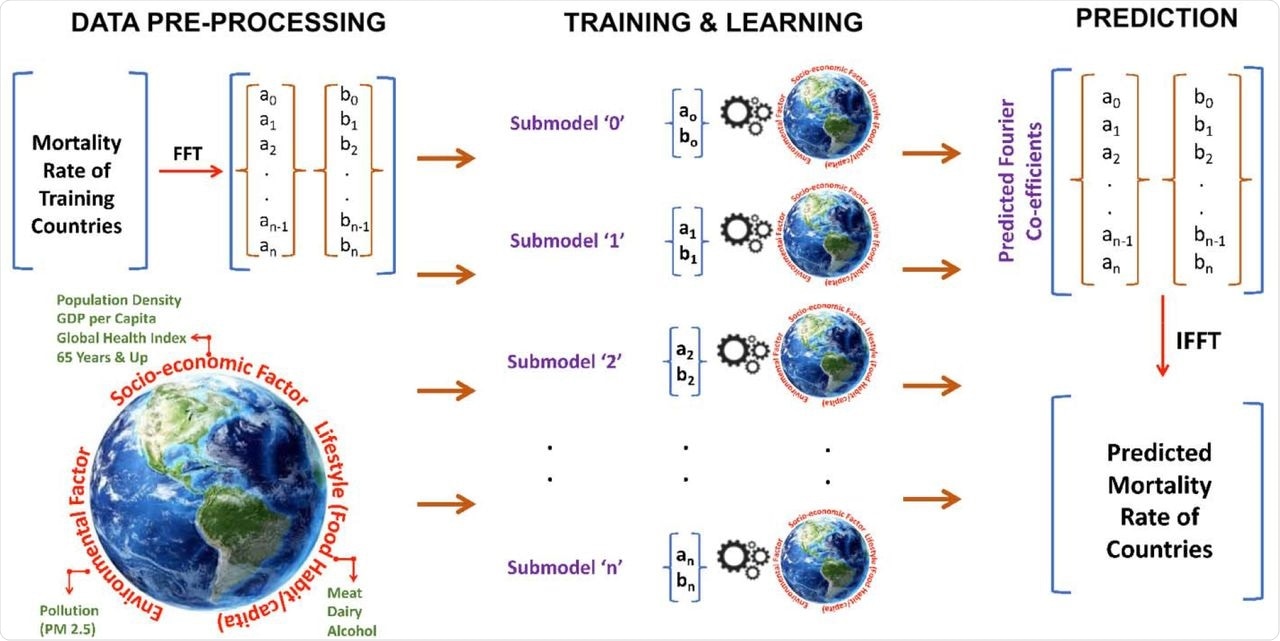
An overview of the scientific model is shown. The model has 3 distinct parts-data pre-processing, training & learning and prediction.
The death rate for each country was calculated as the ratio of the number of deaths per million to the number of COVID-19 cases per million of the respective country.
The current study was conducted from March 15, 2020, to March 15, 2021, while it presented an overall long-term holistic view of COVID-19 mortality rates from May 15, 2020, to February 14, 2021.
Study findings
The results demonstrated how the COVID-19-related mortality rate was closely dependent on a multitude of socioeconomic and biological factors, such as population density, GDP per capita, the global health index, and proportion of elderly (over 65 years) in the population, as well as environmental factors, lifestyle, and food habits. Interestingly, none of these parameters individually showed any noticeable or significant change that could be generalized with COVID-19 mortality.
The study findings clearly suggested that a single biological or socioeconomic factor cannot explain COVID-19 mortality rates across countries. Thus, for Slovenia, alcohol consumption and the global health index appeared to predict the mortality rates close to the actual data, while GDP per capita and PM2.5 closely predicted the mortality rate for the United States.
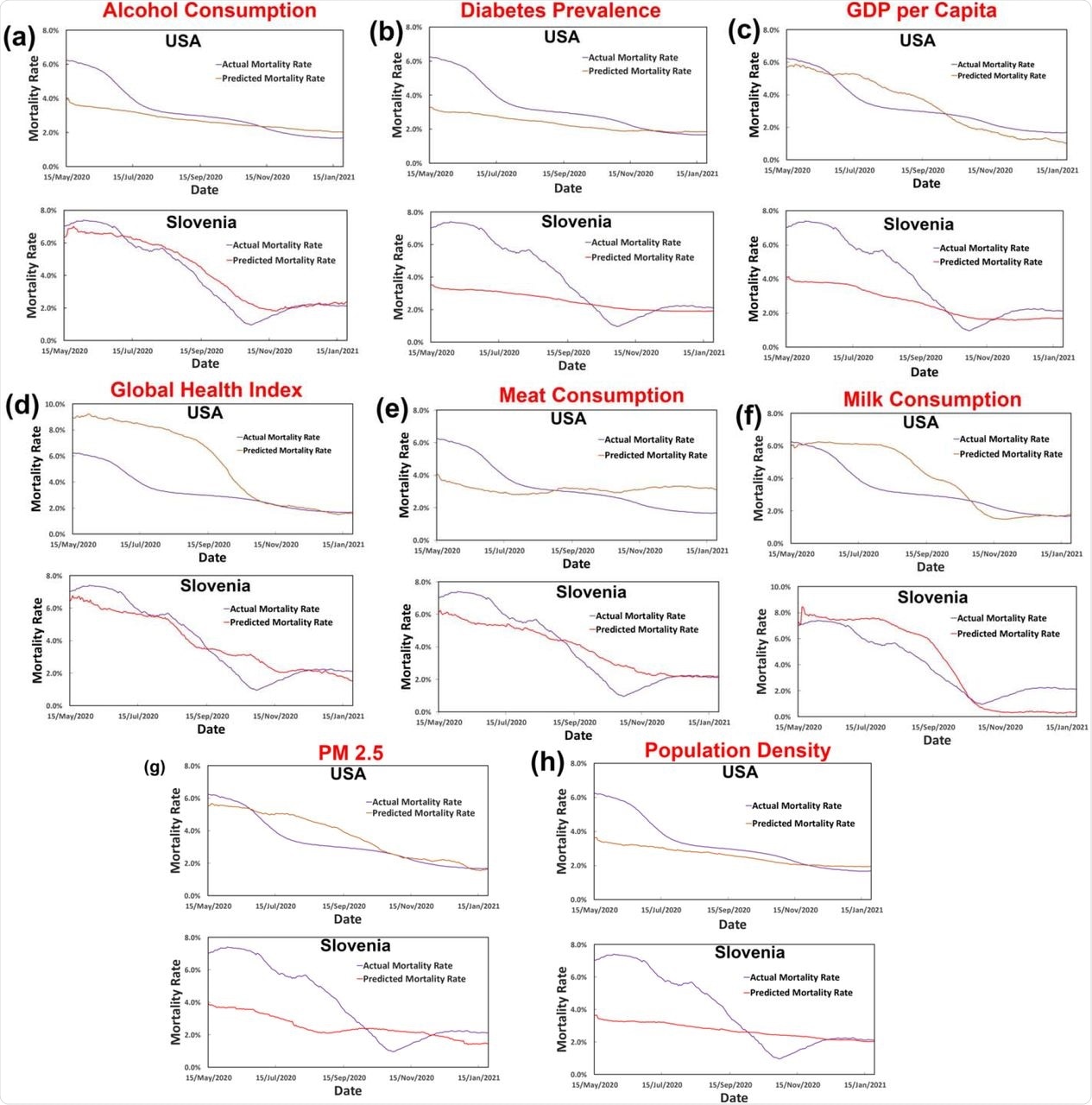
Prediction of COVID-19 related mortality rate using a single factor for both USA and Slovenia are shown, where the factors are – (a) alcohol consumption, (b) diabetes prevalence, (c) GDP per capita, (d) global health index, (e) meat consumption, (f) milk consumption, (g) PM 2.5 and (h) population density. None of the factors individually can describe the trend comprehensively.
The temporal evolution of the COVID-19 mortality rates was almost the same in all countries. While mortality rates during the initial phase of the study were the highest, they subsequently dipped and eventually plateaued, except in South Africa. These fluctuations in mortality rates are largely attributable to the surge of the severe acute respiratory syndrome coronavirus 2 (SARS-CoV-2) Beta variant.
The predicted COVID-19 mortality rates and actual mortality rates of 20 randomly selected countries were strikingly similar. To this end, the variations were due to the poor quality of COVID-19 data and physiological differences, such as blood type.
Conclusions
In the current study, the quality of COVID data used in the prediction model lacked granularity and specificity, thereby making it practically impossible to obtain the COVID-19 mortality rate or a trend for the world population.
The researchers utilized the Pandas software library for proper consolidation of data for each of the parameters. They gathered all data for a specific parameter for different countries together and, if the data corresponding to some country was missing (NULL), they removed that parameter. When NULL values corresponding to a country exceeded a certain threshold, that country was excluded from the analysis.
Despite extensive data pre-processing efforts, data was granular and lacked specificity. Yet, the study predictions successfully demonstrated the impact of eight influencing factors on mortality rates and mapped the evolutionary trend of COVID-19 mortality rates across the world population.
Overall, with these predictions, the authors managed to initiate conversations among policymakers, public health authorities, and world leaders. This information will subsequently allow countries to take proactive actions and make timely preparations for COVID-19 and other virus-related outbreaks in the future by taking a holistic view.

*Important notice: medRxiv publishes preliminary scientific reports that are not peer-reviewed and, therefore, should not be regarded as conclusive, guide clinical practice/health-related behavior, or treated as established information.