Please could you introduce yourself and tell us what inspired your latest research into antibiotic resistance?
Professor Borgwardt: I am a professor for Machine Learning at ETH Zürich in the Department of Biosystems Science and Engineering, which is located in Basel. We have strong collaborations with the neighboring University of Basel and the University Hospital Basel. As part of these collaborations, Prof. Adrian Egli, who heads the Clinical Microbiology Lab at the University Hospital, and I discussed approaches on how to use the big data that is generated every day in routine clinical diagnostics for personalized medicine.
We identified MALDI-TOF mass spectra, which are routinely used for pathogen species identification, as one such source of data. We hypothesized whether it also could be helpful in antimicrobial resistance prediction via machine learning, which was the starting point for this project. We then received funding from the Kantons of Basel-Stadt and Basel-Landschaft to explore this idea together in a joint team that combines expertise in machine learning and microbiology.
Antibiotic-resistant bacteria are rising rapidly across the globe and have been described by the World Health Organization (WHO) as one of the biggest threats to global health. Can you describe what is meant by the term antibiotic resistance and why have we seen the numbers of these resistant bacteria rise over recent years?
Professor Egli: Antibiotic resistance means that a bacterium can no longer be inhibited in its growth by an antimicrobial drug. Antibiotic resistance evolved over millions of years, as bacteria have learned to produce their own antibiotics to kill or slow down bacterial competitors. In order to not be affected, bacteria have developed a series of resistance mechanisms.
Due to the current (over-)usage of antibiotics in veterinary medicine, food production, and in human medicine, antibiotic-resistant strains have an advantage in certain situations and have successfully spread in different environments, including the human population, where antibiotic-resistant strains can cause a lot of harm due to a reduced susceptibility against such drugs. As a consequence, today, antibiotic resistance is seen as one of the most urgent and impactful threats to modern medicine.
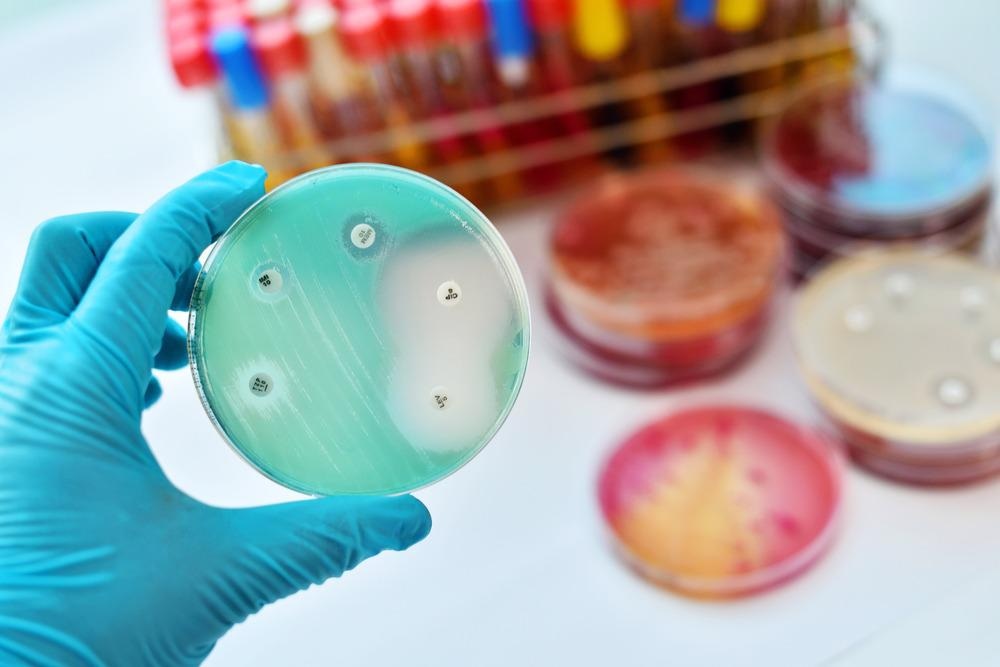
Image Credit: Jarun Ontakrai/Shutterstock.com
Rapid diagnostic tools are helping to curb the spread of antibiotic-resistant bacteria, however, these tools are often subject to limitations. What are some of the limitations associated with rapid diagnostic testing of bacteria?
Professor Egli: Current antibiotic resistance testing is based on culturing bacteria using specific agar plates and specific conditions e.g. aerobic incubation. From sample collection to availability of antibiotic resistance profiles this takes 48-72h. During this time, an untargeted antibiotic treatment is initiated. In order to cover all sorts of potential pathogens, an empiric antibiotic treatment is initiated which is usually very broad.
Broad-spectrum antibiotics, however, induce antibiotic resistance in a series of bacteria e.g. colonizing the patients, and in addition, such a non-targeted approach may also cause important side effects. The advantages of culture-based resistance tests are their low costs and decades of experience in laboratories.
In contrast, molecular diagnostics directly from a sample or a single bacterial colony offers a very fast, but narrow evaluation of the resistance mechanisms. In a PCR often just a few genes of interest are covered and this may be too narrow to capture the resistance mechanism in place. PCR-based diagnostics is also often quite expensive as it requires specialized equipment. The advantage of PCR-based resistance tests is their rapid speed.
Our proposed strategy is still dependent on culturing the bacteria but applies the tests about 24h earlier to conventional resistance testing - thereby providing a much more rapid diagnosis.
Your latest research looked into a new method to identify signs of antibiotic resistance. Can you describe how you carried out your latest research? What did you discover?
Professor Borgwardt: Our goal was to develop a machine learning system that can predict antimicrobial resistance faster than the current clinical workflow can measure it experimentally.
To this end, we combined hundreds of thousands of MALDI-TOF mass spectra of bacterial isolates from laboratories across northwestern Switzerland along with their experimentally determined resistance phenotypes. We used this big dataset to train a machine learning method to distinguish mass spectra from resistant and from sensitive samples. We showed that the resulting machine learning model could predict resistance with high accuracy, in particular for a number of clinically highly important species-antibiotics pairs.
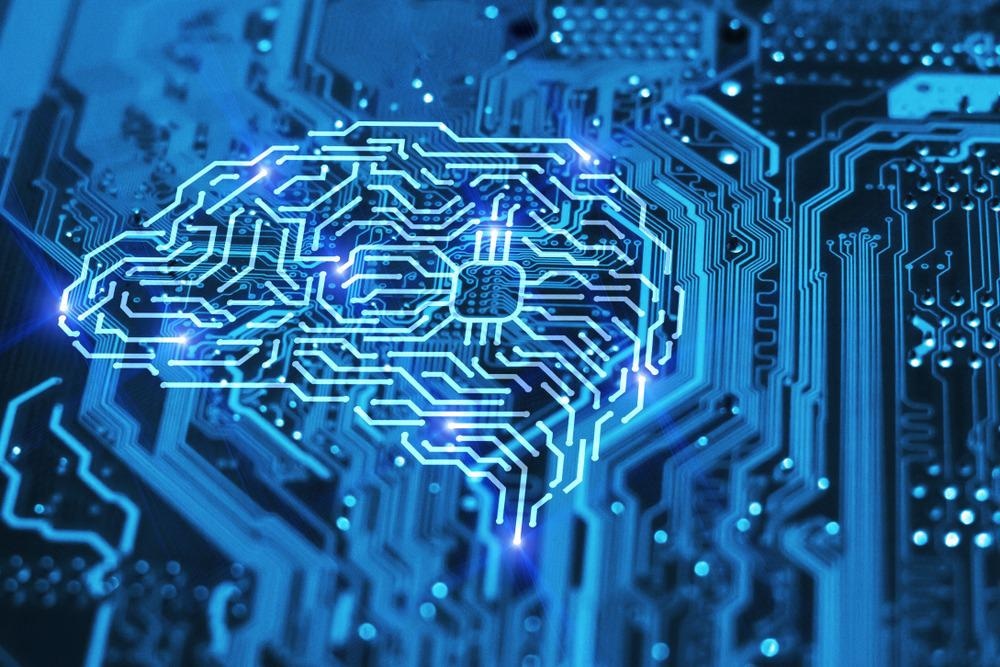
Image Credit: Michael Traitov/Shutterstock.com
Your new method is able to identify signs 24 hours earlier than previous methods. What advantages does this have not only for research but for the treatment of patients?
Professor Borgwardt: The prediction of resistance can be made once a MALDI-TOF mass spectrum has been measured for a bacterial isolate. The prediction is instant, it is a matter of seconds, and is therefore much faster than the regular clinical workflow, which would further grow the bacteria for 24 hours or more and expose them to antimicrobials in order to experimentally determine their resistance phenotypes.
For the clinician, this means that a prediction of antimicrobial resistance would be available much earlier than before. This offers the possibility to tailor the antimicrobial treatment to the (predicted) resistances of a pathogen much earlier, thereby improving antibiotic stewardship. We explored this point in a retrospective clinical study including 63 patients and found that the predictions of our algorithms would have caused an infectious diseases specialist to choose a different therapy in 9 cases.
In 8 of these cases, the change represented a beneficial de-escalation or continuation of the antibiotic treatment, and only in a single case would there have been an unnecessary escalation of the treatment.
You focused on the most important antibiotic-resistant bacteria including E.coli and MRSA. Why was this? Are you hoping to keep expanding your dataset to include other less known antibiotic-resistant bacteria?
Professor Egli: E. coli, Klebsiella pneumoniae, and Staphylococcus aureus are part of the ESKAPE group of pathogens. This group is notoriously known to develop antibiotic resistance. In the case of E. coli and K. pneumoniae the acquisition of a so-called extended-spectrum beta-lactamase (EBSL) and in the case of S. aureus, the development of methicillin resistance are very common and clinically important resistance mechanisms.
The dataset in this sense is biased to these common resistance mechanisms in Switzerland. Obviously, other resistance mechanisms of interest would include Vancomycin resistance in enterococci or Carbapenemase production in various Gram-negative bacteria. We currently plan a larger study to further expand this and validate our algorithm.
What role did artificial intelligence (AI) play in your research?
Professor Borgwardt: Artificial intelligence, in the form of machine learning, allowed us to develop a predictive system for antimicrobial resistance. It could detect subtle similarities in this big dataset of hundreds of thousands of MALDI-TOF mass spectra that are correlated with antimicrobial resistance. This combination of big medical data and machine learning algorithms allowed us to create a predictive system to address a fundamental clinical challenge.
Do you believe that your new method will potentially help us to further understand antibiotic resistance?
Professor Egli: We do not believe that the method per se will help us to better understand the evolution of antibiotic resistance, and it will also not replace the currently established and very reliable methods, but the new method will allow us to rapidly screen patients and provide an early assessment, which can be important in a critically ill patient - thereby the antibiotic therapy may be adapted according to this early available information from our algorithm. However, until this is clinical practice, we will need to validate our findings in a prospective study.
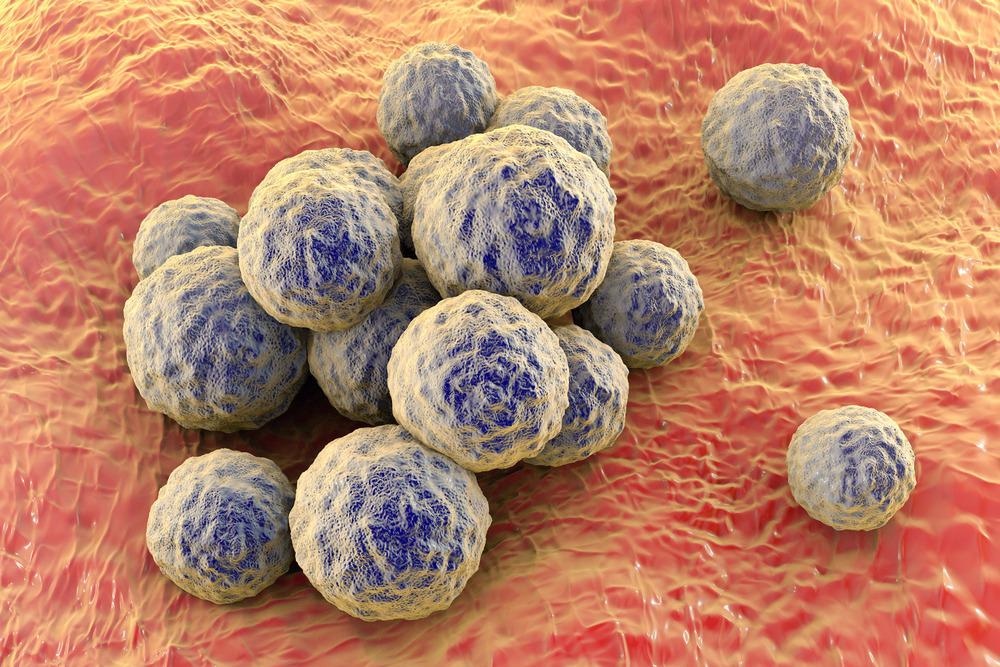
Image Credit: Kateryna Kon/Shutterstock.com
What are the next steps for you and your research?
Professor Borgwardt: From a clinical point of view, we are now planning this prospective clinical validation of our prediction approach. From a machine learning perspective, we will explore how to improve the robustness of our predictions across locations and time. It’s a project that combines exciting clinical with exciting machine learning questions.
In a related branch of work, Prof. Egli and I are co-coordinating the Personalized Swiss Sepsis Study, Switzerland’s effort to predict sepsis in intensive care units (ICUs) with machine learning. With a team of more than 20 PIs from all over Switzerland and data from the ICUs of all Swiss university hospitals, we are trying to create another example of the success of artificial intelligence in medicine.
Where can readers find more information?
Publication in Nature Medicine journal: https://doi.org/10.1038/s41591-021-01619-9
DRIAMS Dataset in Dryad repository: https://doi.org/10.5061/dryad.bzkh1899q
Personalized Swiss Sepsis Study: https://sepsis-network.ch/
About Professor Karsten Borgwardt
Karsten Borgwardt is a Full Professor of Machine Learning in the Life Sciences at ETH Zürich, in the Department of Biosystems Science and Engineering located in Basel. His work won several awards, including the 1 million EUR Krupp Award for Young Professors in 2013 and a Starting Grant 2014 from the ERC-backup scheme of the Swiss National Science Foundation..jpg)
Prof. Borgwardt has been and is leading large national and international research consortia, including the SPHN/PHRT driver project “Personalized Swiss Sepsis Study” (2018-2022) with Prof. Egli (USB), and two European Marie Curie Innovative Training Networks on Machine Learning in Medicine (2013-2016 and 2019-2022).
About Professor Adrian Egli
Prof. Egli is the head of Clinical Bacteriology and Mycology at the University Hospital Basel and an independent research group leader at the University of Basel. He is a PI of the SPHN/PHRT driver project “personalized swiss sepsis study”, jointly with Prof. Borgwardt (ETH). He is also a founding member of the Swiss Pathogen Surveillance Network (www.spsp.ch), which is a genomic data exchange platform for bacterial and viral pathogens..jpg)
He is an expert in bacterial and viral genomics in clinical microbiology and his research explores host-pathogen interactions, focusing on pathogen evolution and transmission.