In a recent study posted to the medRxiv* preprint server, researchers applied deep learning methods and identified genetic variants linked to severe acute respiratory syndrome coronavirus-2 (SARS-CoV-2)-caused mortality.
The coronavirus disease 2019 (COVID-19) pandemic, caused by SARS-CoV-2, has globally resulted in more than 518 million cases and over 6.25 million deaths to date. Researchers have observed that older people, men, Asians, Blacks, and other ethnic minorities are at higher risk of COVID-19-related mortality. In addition, it has been observed that host genetic determinants affect infection and disease severity risk.
Although several researchers have explored the genetic associations to COVID-19 outcomes in genome-wide association studies (GWAS), they focused only on the effects of single-nucleotide polymorphisms (SNPs) on phenotypes. Hence, evaluating and identifying host genetic factors related to heterogeneous susceptibility to SARS-CoV-2 and severity might augment our current understanding of COVID-19 and facilitate the development of drugs.
Study: Deep learning identified genetic variants associated with COVID-19 related mortality. Image Credit: issaro prakalung / Shutterstock

This news article was a review of a preliminary scientific report that had not undergone peer-review at the time of publication. Since its initial publication, the scientific report has now been peer reviewed and accepted for publication in a Scientific Journal. Links to the preliminary and peer-reviewed reports are available in the Sources section at the bottom of this article. View Sources
About the study
In the present study, researchers implemented a novel approach termed deep learning-based ranking and aggregation method for identifying genetic variants (DRAG). Three steps are involved in the DRAG process: SNP-set partition, selection of optimal SNP subsets, and determination of groups of variants (hereafter referred to as super variants).
The whole dataset (complete set) was partitioned into 1) discovery and 2) verification sets in a 2:1 ratio. The discovery set encompassed data on 17,627 COVID-19 survivors and 1104 deaths, and the verification set contained 8814 fatalities and 552 survivors. First, DRAG was trained to identify initial candidates in the first half of the discovery set. Then, logistic regression was implemented in the second half of the set to find initial optimal super variants. These were then extracted and aggregated into super variants on the verification dataset. A super variant was considered verified if a 0.05 level of significance was obtained for its logistic regression coefficient.
Results
The authors identified more than 28,000 White individuals of British ancestry infected with SARS-CoV-2 from the UK Biobank. The team considered more than 8.23 million SNPs and grouped them into 2,734 SNP sets of 1 mega base-pair length each. About 15 super variants were identified in the discovery set with p-values ≤ 0.05 and were validated in the verification set. Upon validation, all detected super variants had p-values < 0.05 including one which showed a p-value < 0.003.
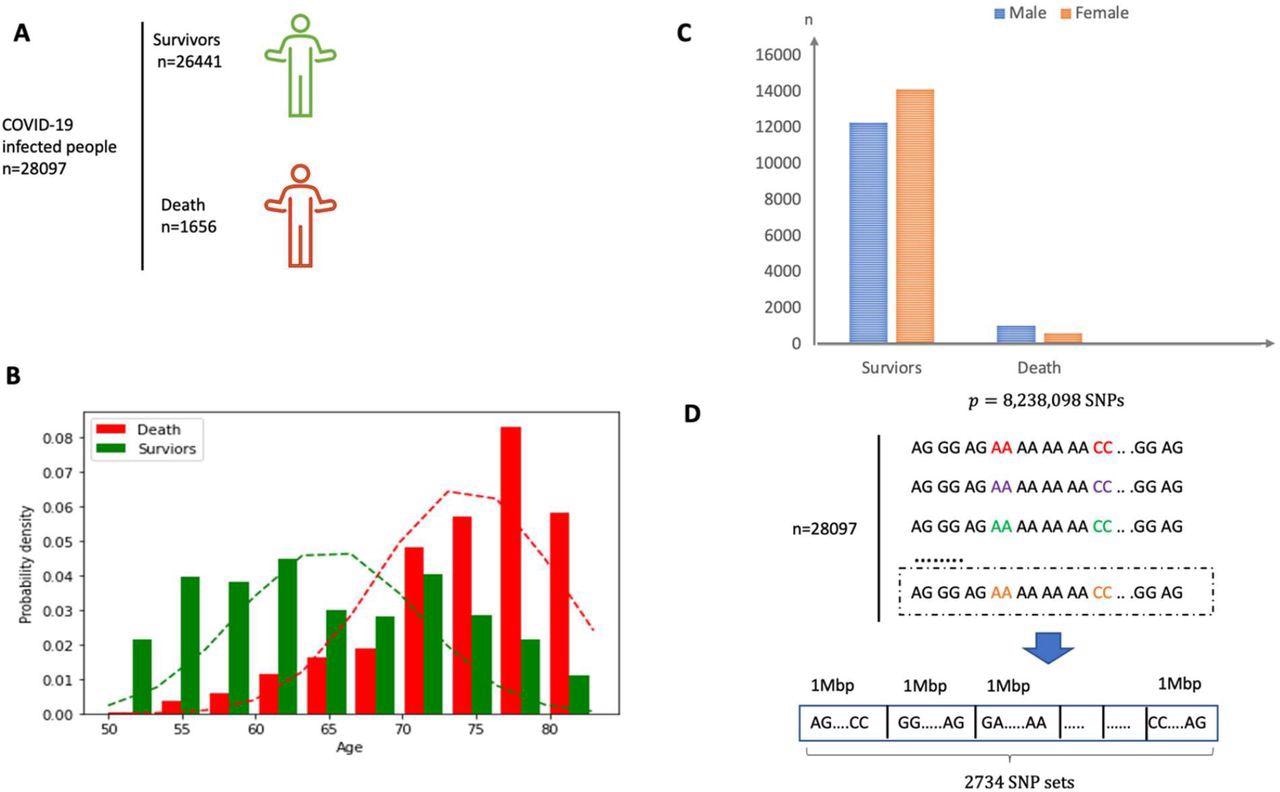
(A) Overview of the participants included and the samples and data collected. (B) Sex distribution in both survivor and death group. (C) Age distribution in both survivor and death group. The mean of age for death group is around 75 years old. (D) The SNP dataset are divided into 2734 non-overlapping local sets according to the physical position and each set consists of SNPs within a segment of physical length 1 Mbp.
Four genetic variants reported with COVID-19 outcomes were identified at or near zinc finger and BTB domain containing 16 (ZBTB16), taste 2 receptor member 1 (TAS2R1), long intergenic non-protein coding RNA 1320 (LINC01320), and neural cell adhesion molecule 1 (NCAM1). The super variant chr11_114 contained seven SNPs, including one in the intron of the NCAM1 gene and the other as the intronic variant of ZBTB16.
Previous studies speculated the possibility of molecular mimicry between SARS-CoV-2’s envelope protein and NCAM1. Likewise, ZBTB16, critical for immune system development, was lately found upregulated in the tears of COVID-19 patients. The intron-less TAS2R1 gene encodes a bitter taste receptor, a transmembrane protein. Those reporting weak or no bitter tastes were at higher odds of testing COVID-19-positive and requiring hospitalization.
The researchers found eight novel genes that might be associated with COVID-19 mortality. These were DExD/H-box 60 like (DDX60L), heat shock protein family a member 9 (HSPA9), LncRNA associated with SART3 regulation of splicing (LASTR), GLI family zinc finger 3 (GLI3), ArfGAP with GTPase domain, ankyrin repeat and PH domain 3 (AGAP3), mono-ADP ribosylhydrolase 2 (MACROD2), nucleoporin 93 (NUP93), and ELOVL fatty acid elongase 5 (ELOVL5).
chr4_170 super variant has four SNPs, including one in the intron of DDX60L. Although DDX60L function is poorly defined, it has been reportedly involved in antiviral immunity. The super variant chr5_138 comprises eight SNPs, one of them upstream of HSPA9.
Variations in the HSPA9 gene might affect COVID-19 severity, given that knockdown of HSPA9 results in the decline of B cells. Of the four SNPs in the chr6_54 super variant, one is present in the intergenic sequence of ELOVL5. A prior GWAS noted the association of this gene with lung carcinoma, and it is known that lung cancers modestly increase the COVID-19 mortality risk.
The chr20_15 super variant is composed of eight SNPs, all of which lie in the intronic sequences of the nearby MACROD2 gene. chr16_57 super variant comprises nine SNPs, one in the NUP93 gene’s intron. One study noted disruption of NUP93 localization from nuclear pore complex by nonstructural protein 1 (nsp1) of SARS-CoV, and the authors posit similar disruptive activity by SARS-CoV-2 on NUP93. SNPs in other super variants lie close to LASTR (chr7_43), GLI3 (chr7_151), and AGAP3 (chr10_6). These three genes were reported to be related to pulmonary function.
Next, the authors conducted GWAS and identified five loci on the fifth chromosome associated with COVID-19 mortality. Lastly, in a simulations study, the authors observed DRAG to be superior to and outperforming the established method of tree-based analysis of rare variants (TARV) by a large margin, implying that DRAG could easily handle complex interactions of SNPs even from enormous data.
Conclusions
To summarize the findings, a deep learning (DRAG) method was developed to study the relationship between COVID-19-induced mortality and genetic variants. The team identified 15 super variants and evaluated the association with SARS-CoV-2-related mortality. The restricted ethnic composition of the study population limits the generalizability of the results. Notably, the association between the identified genetic variants and disease outcomes was not functionally validated, warranting more investigations in the future. These findings provide glimpses into the molecular pathogenesis of COVID-19, which may have implications for its treatment in clinical practice.

This news article was a review of a preliminary scientific report that had not undergone peer-review at the time of publication. Since its initial publication, the scientific report has now been peer reviewed and accepted for publication in a Scientific Journal. Links to the preliminary and peer-reviewed reports are available in the Sources section at the bottom of this article. View Sources
Journal references:
- Preliminary scientific report.
Deep learning identified genetic variants associated with COVID-19 related mortality. Zihuan Liu, Wei Dai, Shiying Wang, Yisha Yao, Heping Zhang, medRxiv 2022, DOI: https://doi.org/10.1101/2022.05.05.22274731, https://www.medrxiv.org/content/10.1101/2022.05.05.22274731v1
- Peer reviewed and published scientific report.
Liu, Zihuan, Wei Dai, Shiying Wang, Yisha Yao, and Heping Zhang. 2023. “Deep Learning Identified Genetic Variants for COVID‐19‐Related Mortality among 28,097 Affected Cases in UK Biobank.” Genetic Epidemiology, January. https://doi.org/10.1002/gepi.22515. https://onlinelibrary.wiley.com/doi/abs/10.1002/gepi.22515.
Article Revisions
- May 13 2023 - The preprint preliminary research paper that this article was based upon was accepted for publication in a peer-reviewed Scientific Journal. This article was edited accordingly to include a link to the final peer-reviewed paper, now shown in the sources section.