By Neha MathurReviewed by Danielle Ellis, B.Sc.May 26 2022
In a recent study posted to the bioRxiv* pre-print server, researchers identified several new severe acute respiratory syndrome coronavirus 2 (SARS-CoV-2) epitopes displayed on human leukocyte antigens class I (HLA-I) molecules.
The recognition of these epitopes by the cluster of differentiation 8 (CD8)+ T-cell receptors (TCRs) is crucial to clear pathogenic infections and mounting a response to cancer immunotherapy.
Study: Predictions of immunogenicity reveal potent SARS-CoV-2 CD8+ T-cell epitopes. Image Credit: fusebulb / Shutterstock

This news article was a review of a preliminary scientific report that had not undergone peer-review at the time of publication. Since its initial publication, the scientific report has now been peer reviewed and accepted for publication in a Scientific Journal. Links to the preliminary and peer-reviewed reports are available in the Sources section at the bottom of this article. View Sources
Background
The epitopes or peptides presented on HLA-I molecules have several clinical applications. Thus, detailed knowledge of these epitopes could help design vaccines targeting neoepitopes derived from non-synonymous genetic mutations for cancer immunotherapy. Moreover, these epitopes could be used to select TCRs and reinfuse them into patients in need of T-cell therapy.
The diversity of HLA alleles and their presence in large numbers make it extremely challenging to identify epitopes specific to cancer and infectious diseases, including coronavirus disease 2019 (COVID-19). For instance, the number of potential class I epitopes of a given length in a pathogen roughly equals its proteome length. Despite advancements in the screening methods of potential epitope candidates, the most common approach is to preselect them based on HLA-I ligand predictors.
About the study
In the present study, researchers curated an enormous dataset of naturally presented HLA-I ligands and experimentally verified class I neo-epitopes. They integrated this data with new algorithms to train and improve predictors of antigen presentation and TCR recognition used to date. The researchers applied these tools to SARS-CoV-2 proteins to predict and validate several epitopes. Further, they characterized these epitopes for TCR functional avidity, cross-reactivity, and clonality.
The researchers retrieved immunogenic and non-immunogenic neo-epitopes obtained from several neo-antigen studies and completed by neo-epitope data from the immune epitope database (IEDB). They obtained a total of 596 experimentally verified neo-epitopes, with 10 8-mers, 391 9-mers, 148 10-mers, 47 11-mers, and 6084 experimentally verified non-immunogenic peptides.
The researchers screened 24 HLA-I peptidomics studies to fetch 244 samples of naturally presented HLA-I ligands of lengths between eight to 14-mers. They used the MixMHCp, a motif deconvolution algorithm, to process these samples and identify shared HLA-I motifs across samples having the same alleles. The final dataset of HLA-I ligands comprised 258,814 unique peptides.
The team calculated peptide length distributions for all HLA-I ligands from mono- and poly-allelic samples separately. They trained a predictor of antigen presentation termed MixMHCpred v2.2 and a predictor of immunogenicity called PRIME2.0. They benchmarked MixMHCpred v2.2 using two external datasets not included in the training of any antigen presentation predictor. They used four-fold excess of randomly selected peptides from the human proteome as negatives to compute receiver operating curves (ROC) and positive predictive values (PPV).
The difference in the performance of HLA-I ligand predictors originated from differences in modeling binding specificities or peptide length distributions. Therefore, the researchers computed the Euclidean distance between motifs predicted by each predictor at different %rank thresholds and those observed experimentally in HLA-I peptidomics data.
It is noteworthy that the team expressed the final score of an epitope as a %rank, which depicted how the predicted binding of an epitope compared to random peptides from the human proteome.
Study findings
Compared to other predictors, MixMHCpred2.2 predicted lower distances for HLA-I binding motifs, indicating that the only significant difference was at the level of peptide length distributions. However, motifs predicted with HLAthena and MixMHCpred2.0.2 were not very distant from those observed in HLA-I peptidomics data.
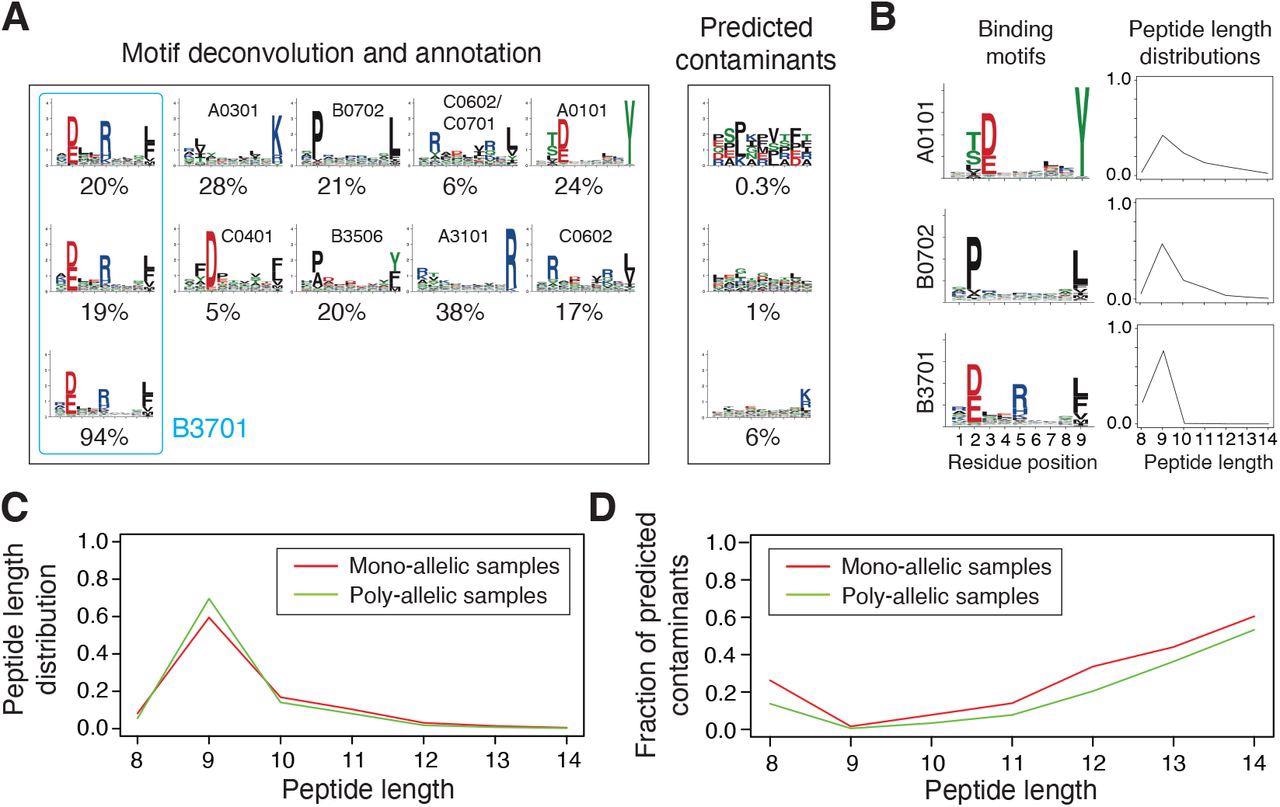
Integration and curation of HLA-I peptidomics data reveal binding motifs and peptide length distributions for more than hundred alleles. (A) Motif deconvolution includes identification of motifs and predicted contaminants with MixMHCp, as well as motif annotation by identifying shared motifs across samples sharing the same allele. The example shows the deconvolved motifs in two poly-allelic samples that share the HLA-B*37:01 allele (‘donor1’ and ‘HCC1143’ in Dataset S1), as well as the mono-allelic HLA-B*37:01 sample. (B) Examples of binding motifs and peptide length distributions obtained by motif deconvolution and used to train MixMHCpred2.2. (C) Peptide length distributions for alleles observed in both mono-allelic and poly-allelic HLA-I peptidomics data. Each curve represents the average peptide length distribution across these alleles. (D) Fraction of predicted contaminants across different lengths (average over all samples).
MixMHCpred2.0.2 over-represented longer peptides for high %rank while HLAthena under-represented nine-mers and over-represented eight-, 10- and 11-mers across all % rank thresholds. The discrepant observations indicated that integrating peptide lengths was crucial to accurately capture the length distribution of naturally presented HLA-I ligands across different alleles.
Analysis of data used to train PRIME2.0 confirmed the importance of aromatic and hydrophobic residues, especially tryptophan, in the region recognized by the TCR, reflecting its ability to engage in stable molecular interactions with the TCR. Conversely, for low-affinity HLA-I ligands, the presence of amino acids favoring TCR recognition and counterbalancing the lower stability of the peptide-HLA-I complexes became important.
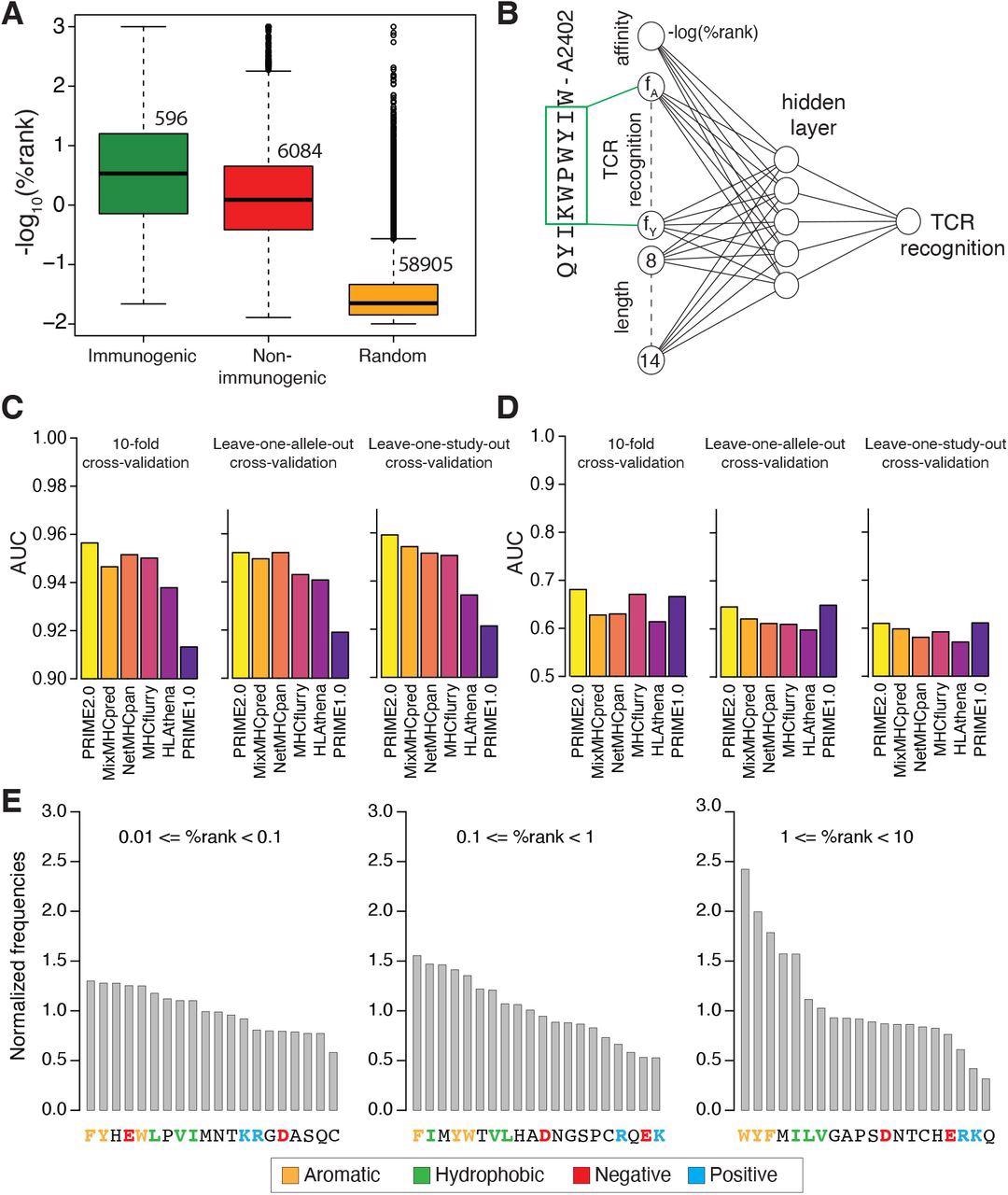
Models of TCR recognition improve predictions of neo-epitopes. (A) experimentally validated immunogenic (green) and non-immunogenic (red) peptides, as well as random peptides (orange) used to train PRIME. (B) Architecture of neural network of PRIME2.0. The first input node corresponds to the predicted binding to the HLA-I allele (-log(%rank) from MixMHCpred2.2). The next 20 nodes correspond to amino acid frequencies on residues with minimal impact on predicted affinity to the HLA-I allele (green box). These positions were determined as previously described (Schmidt et al., 2021). The last seven nodes correspond to the length of the peptide (i.e., 8 to 14, one-hot encoding). (C) Benchmarking of PRIME2.0 based on 10-fold cross-validation, leave-one-allele-out cross-validation and leave-one-study-out cross-validation. Each bar shows the average AUC within the different types of cross-validations (see also Figure S4A). (D) Same cross-validation as in (C) after excluding randomly generated negatives in the test set (see also Figure S4B). (E) Normalized amino acid frequencies at positions with minimal impact on predicted affinity to HLA-I for immunogenic versus non-immunogenic peptides used to train PRIME2.0 within different ranges of predicted HLA-I binding (%rank of MixMHCpred).
A monoclonal population of antigen-experienced CD8+ T cells with an effector or memory phenotype recognized SARS-CoV-2 epitope QYIKWPWYIW, which had high homology with SARS-CoV-1 epitope QYIKWPWYVW and was 100% conserved among all SARS-CoV-2 variants. The findings suggest that CD8+ T-cell responses induced by prior pathogenic infection, vaccination, or cross-reactivity with SARS-CoV-1 were effective against all SARS-CoV-2 variants.
Conclusion
To summarize, several existing tools are accurate at HLA-I ligand predictions. However, predicting TCR recognition remains challenging because of the smaller size of the training data and other factors, such as co-receptors, and cytokines, that influence TCR recognition. Therefore, sustained efforts to develop high-quality immunogenicity training data and better machine learning frameworks are needed to further improve class I epitope predictions.

This news article was a review of a preliminary scientific report that had not undergone peer-review at the time of publication. Since its initial publication, the scientific report has now been peer reviewed and accepted for publication in a Scientific Journal. Links to the preliminary and peer-reviewed reports are available in the Sources section at the bottom of this article. View Sources
Journal references:
- Preliminary scientific report.
Predictions of immunogenicity reveal potent SARS-CoV-2 CD8+ T-cell epitopes, David Gfeller, Julien Schmidt, Giancarlo Croce, Philippe Guillaume, Sara Bobisse, Raphael Genolet, Lise Queiroz, Julien Cesbron, Julien Racle, Alexandre Harari, bioRxiv pre-print 2022, DOI: https://doi.org/10.1101/2022.05.23.492800, https://www.biorxiv.org/content/10.1101/2022.05.23.492800v1
- Peer reviewed and published scientific report.
Gfeller, David, Julien Schmidt, Giancarlo Croce, Philippe Guillaume, Sara Bobisse, Raphael Genolet, Lise Queiroz, Julien Cesbron, Julien Racle, and Alexandre Harari. 2023. “Improved Predictions of Antigen Presentation and TCR Recognition with MixMHCpred2.2 and PRIME2.0 Reveal Potent SARS-CoV-2 CD8+ T-Cell Epitopes.” Cell Systems 14 (1): 72-83.e5. https://doi.org/10.1016/j.cels.2022.12.002. https://www.cell.com/cell-systems/fulltext/S2405-4712(22)00470-7.
Article Revisions
- May 13 2023 - The preprint preliminary research paper that this article was based upon was accepted for publication in a peer-reviewed Scientific Journal. This article was edited accordingly to include a link to the final peer-reviewed paper, now shown in the sources section.