Scientists in the United States have conducted a simulation study to estimate the number of coronavirus disease 2019 (COVID-19) deaths that could have been prevented by increasing vaccination coverage in the country.
The study is currently available on the Research Square preprint* server while under consideration for publication in the journal Scientific Reports.
Study: Estimating vaccine-preventable COVID-19 deaths under counterfactual vaccination scenarios in the United States. Image Credit: Gargantiopa / Shutterstock

*Important notice: Research Square publishes preliminary scientific reports that are not peer-reviewed and, therefore, should not be regarded as conclusive, guide clinical practice/health-related behavior, or treated as established information.
Background
The deployment of effective COVID-19 vaccines was initiated in the United States in early 2021. Although there was a shortage in vaccine supply at the initial phase of deployment, the country successfully increased its vaccine coverage by mid-April 2021.
Studies conducted in real-world setups have shown that COVID-19 vaccines are more than 80% effective in preventing severe disease and hospitalization and over 90% effective in preventing COVID-19-related deaths.
Vaccine hesitancy has been observed among a significant proportion of US adults despite these benefits. An accurate estimation of vaccine-preventable COVID-19 deaths would be helpful in informing policymakers to increase vaccine uptake in the general population.
In the current study, scientists have simulated state-level COVID-19 mortality and vaccination frequency to estimate the number of COVID-19 deaths that could have been prevented by increasing COVID-19 vaccine coverage in the United States.
Study design
The simulation model was developed based on two assumptions. One assumption was that a state could maintain the peak indefinitely upon reaching peak vaccination capacity. Moreover, for a given state, the model assumed that the vaccination rate is maintained at its peak until 85%, 90%, or 100% of the state population is vaccinated.
Another assumption was that there would be no change in the effective reproduction number of COVID-19 cases. The effective reproductive number refers to the number of secondary cases from an index case in a given population of both susceptible and non-susceptible individuals.
The model was used to estimate the number of vaccine-preventable deaths both at the state- and national levels between January 2021 and April 2022.
Important observations
Considering that 100% of the US adult population became fully vaccinated during the study period, the model predicted that about 50% of COVID-19 deaths could have been prevented nationally.
Considering a vaccine coverage rate of 85%, the model predicted that about 28% of actual COVID-19 deaths could have been prevented in the country.
The model identified a significant variation in the proportion of vaccine-preventable deaths between states, with Massachusetts showing 25% and Alaska showing 74% vaccine-preventable deaths.
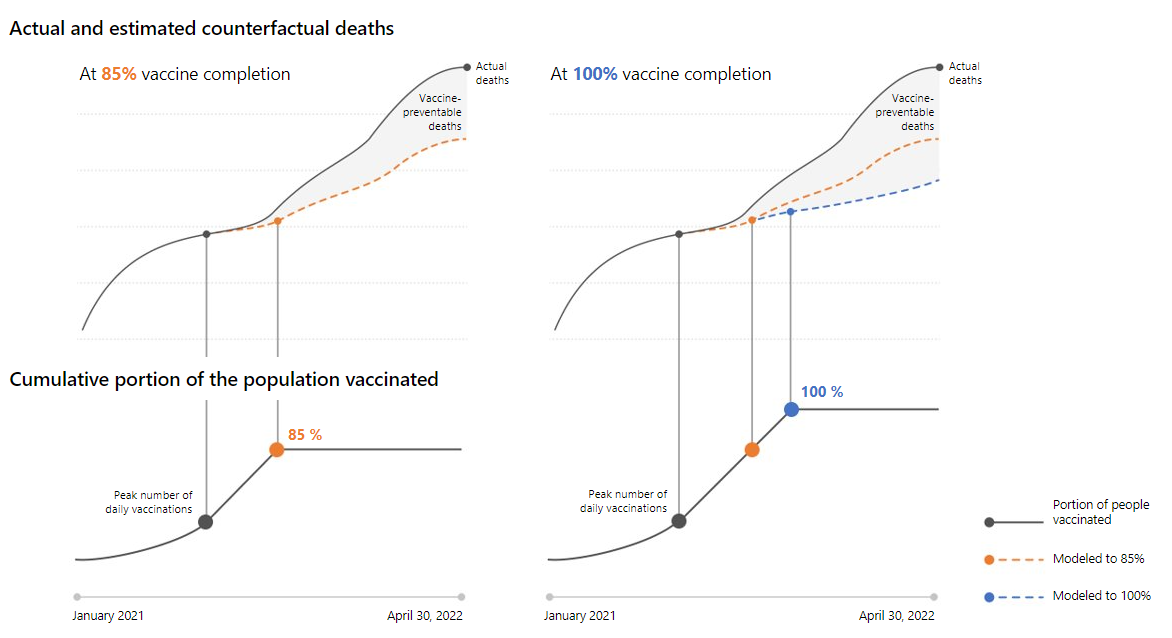
Demonstration of the model, showing the process for estimating vaccine-preventable deaths had 85% (left, orange) or 100% (right, blue) of the adult population been vaccinated. The figure is a representation and does not reflect data for a particular state.
Study significance
The study demonstrates that about 50% of COVID-19 deaths could have been prevented in the United States by maintaining the population-level vaccination rate at its peak. In addition, the high effectiveness of COVID-19 vaccines observed in the study would help inform policymakers to increase vaccine coverage at both state- and national levels.
As mentioned by the scientists, the study does not include age- and race-specific data. Thus, the study could not explore the effect of age and race on vaccine efficacy.

*Important notice: Research Square publishes preliminary scientific reports that are not peer-reviewed and, therefore, should not be regarded as conclusive, guide clinical practice/health-related behavior, or treated as established information.