In a recent study published in Viruses, researchers discuss an open-source and automated bioinformatics pipeline to prospectively and routinely analyze and integrate heterogeneous human immunodeficiency virus (HIV)-1 sequence data. This approach was applied on 18 monthly datasets generated between January 2020 and June 2022 in Rhode Island (RI) in the United States.
The proposed pipeline facilitated routine collaboration between researchers and the RI Department of Health (RIDOH) in near real-time. This approach also allowed researchers to compare the effect of distinct phylogenetic methods and distance-only algorithms with datasets of HIV-1 sequences cluster analyses.
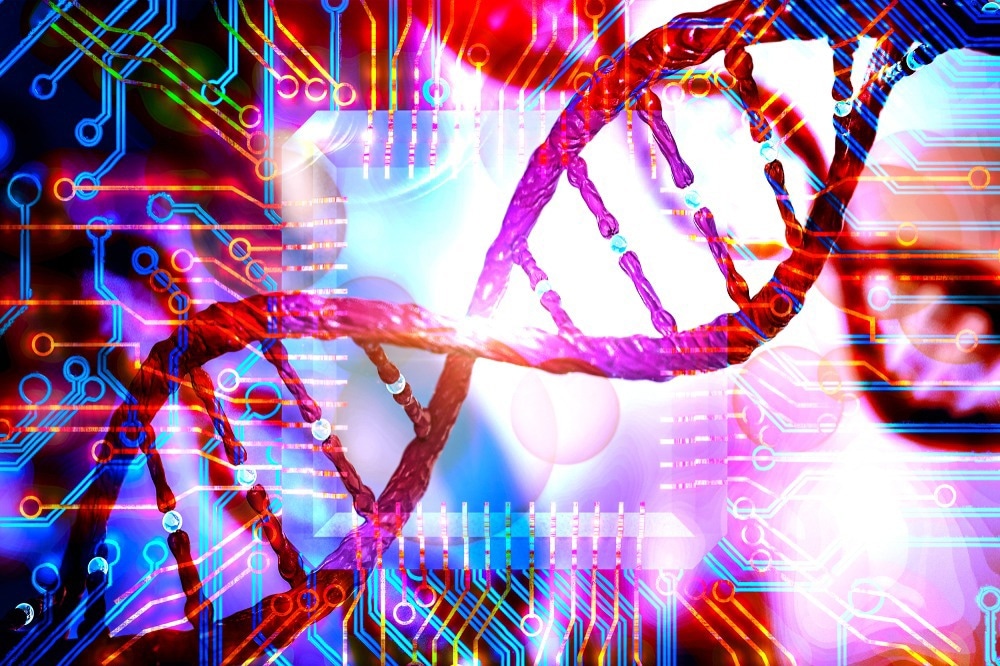
Study: An Automated Bioinformatics Pipeline Informing Near-Real-Time Public Health Responses to New HIV Diagnoses in a Statewide HIV Epidemic. Image Credit: CI Photos / Shutterstock.com
Background
Challenges associated with real-time data integration, analysis, and interpretation delay public health responses, particularly when HIV is considered. Thus, analyzing genomic HIV data or HIV-1 sequences could inform public health responses and ultimately overcome data management, computational, and analytical challenges.
Public health agencies routinely collect HIV-1 sequences during clinical care for drug resistance testing. The same samples could also help estimate viral evolution across individuals.
Just as contact tracing establishes social networks and serves as a proxy for the actual HIV transmission network, phylogenetic relationships among sequences could provide relevant information to guide public health responses. In fact, contact tracing is an independent source of information about social networks, which, in turn, could help detect undiagnosed or diagnosed out-of-care HIV cases.
About the study
In the present study, researchers source and integrate statewide molecular HIV data from clinical, sequence, and public health databases.
SQUAT principles were subsequently used to analyze this data and identify sequences with more than 5% stop codons, guanosine-to-adenosine hypermutation, atypical mutations, and exact edit nucleotides pairwise distance among new sequences. These sequences were then compared with historical molecular HIV-1 sequences.
Following quality analyses, the pipeline was used to detect molecular clusters in sequences recently added from new index cases. To this end, the pipeline used MAFFT v. 7.313 to perform sequence alignments of the initial single HIV-1 sequence multiple times for each patient.
The pipeline implemented five phylogenetic methods and cluster-defining parameters that favored false positive clusters and maximized available information. Likewise, the novel approach used HIV-TRACE v. 0.4.4 to perform distance-only sequence clustering.
At a 1.5% distance threshold, HIV-TRACE detected a similar number of clusters as the phylogenetic methods. Furthermore, this pipeline compared clustering between RI’s statewide dataset with a subset obtained from a single large clinic in RI to evaluate the effect of an augmented sampling density.
After data integration, each pipeline component automatically generated reports. While individual-level reports summarized clustering, demographics, and clinical information of newly added sequences, a population-level report provided statewide clustering summaries. This data identified cluster growth over time, thereby depicting cluster membership of new and previous index cases.
Results
The pipeline developed in the current study incorporated four new features unavailable in prior HIV cluster analysis automated approaches. First, it had a flagging step that explored sequence quality. Second, it implemented several phylogenetic and distance-only clustering methods.
The novel approach also detected clustered individuals using a combination of the five phylogenetic methods. Finally, this pipeline summarized clustering results using visual representations.
While cluster analyses employing distance-only methods also identified large viral transmission networks, this pipeline helped public health officials manage HIV cases in real time. In addition, the pipeline seamlessly removed obstacles to phylogenetic analysis while facilitating replicability.
As compared to distance-only methods, the proposed pipeline detected 76% more clustered HIV cases. More specifically, it identified 37 new HIV cases for case management discussions.
The pipeline also helped researchers examine the differences in cluster identification between a clinic-based and statewide dataset, thus indicating the importance of good sampling. The authors noted that RI’s high statewide sequence sampling density was beneficial.
It is also imperative for careful interpretation and longitudinal accumulation of cluster data for more robust findings compensating for sequence addition-induced reduction of clusters.
Conclusions
The management of the ongoing HIV epidemic is a priority of the U.S. Department of Health and Human Services. The multi-disciplinary approach adopted in this study facilitated case management to disrupt HIV transmission in near-real-time in RI. Furthermore, the approach could allow prospective evaluation of the benefits of phylogenetic data and evidence-based discussions to guide public health intervention strategies.
Optimal integration of genomic and clinical data, including bioinformatics, analytical, and wet laboratory data from healthcare and public health organizations, could improve health outcomes. The authors released this pipeline for automated HIV cluster analysis as an open-source package that has been made available at https://github.com/kantorlab/hiv-real-time-phylogeny
Journal reference:
- Howison, M., Gillani, F. S., Novitsky, V., et al. (2023). An Automated Bioinformatics Pipeline Informing Near-Real-Time Public Health Responses to New HIV Diagnoses in a Statewide HIV Epidemic. Viruses 15(737). doi:10.3390/v15030737