In a recent article published in the journal Sensors, researchers perform a scoping review on the shared relationship between artificial intelligence (AI) and neuroscience, emphasizing their convergence and possible applications.
The researchers extensively searched existing literature relevant to the objective of this review. As a result, the final dataset comprised 185 publications, 173 of which were from scientific databases, and the remaining 12 were hyperlink references from Google.
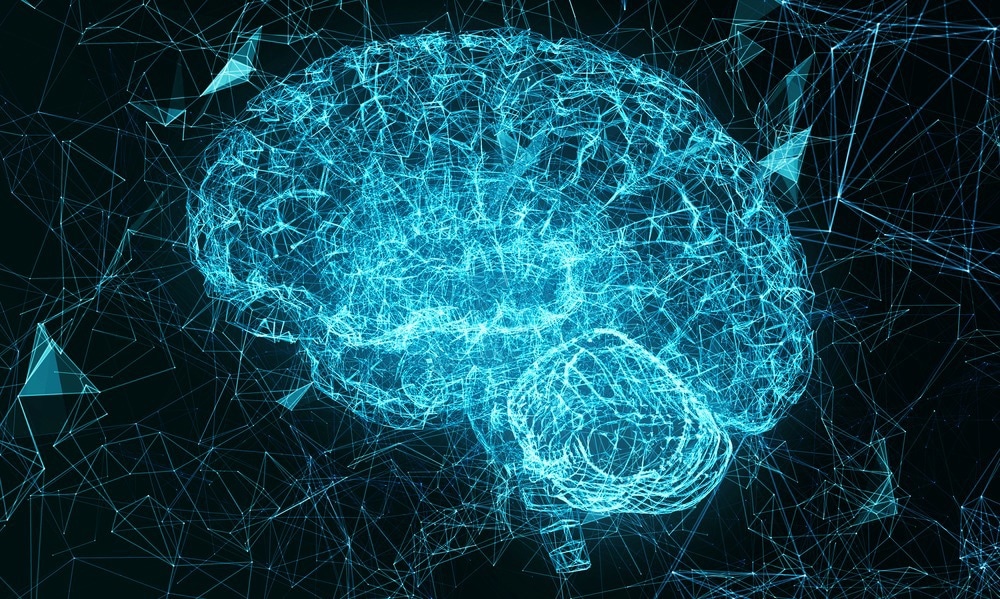
Study: Convergence of Artificial Intelligence and Neuroscience towards the Diagnosis of Neurological Disorders—A Scoping Review. Image Credit: Maxim Gaigul / Shutterstock.com
Background
Neuroscience principles have uplifted the AI field and vice versa. For example, neuroscience has helped researchers validate existing AI-based models. Likewise, biological neural networks have helped develop deep neural network architectures with several applications, such as text processing and speech recognition.
AI-based systems have helped neuroscientists test their hypotheses and analyze neuroimaging data, which, in turn, help with the early prediction and diagnosis of psychiatric disorders. Furthermore, these AI systems set up an interface with the brain, extract neurological signals, and generate commands that help devices, such as robotic arms, to move paralyzed human parts.
Computer scientists have drawn inspiration from reinforcement learning (RL) in human and animal models to develop algorithms for artificial systems, which alleviates the need for explicit instruction when learning complex strategies. RL has been successfully integrated into various robot-based surgery and gaming applications.
Overall, owing to the ability of AI to analyze complex data and extract hidden patterns, this technology appears to be the ideal choice for neuroscience data analysis.
Neuroscience inspired the design of AI systems
Similar to neurons in the human brain, artificial neural networks (ANNs) have several interconnected units that work in parallel. Likewise, the concept of Hebbian learning and brain structure helped Frank Rosenblatt design a simple ANN called perceptron in the 1950s.
Scientists subsequently modified perceptron networks to form multi-layer perceptron (MLP). In MLP, the output of one layer is fed to the next layer through the hidden layer until the last layer produces the expected results.
The human brain's working memory feature inspired scientists to design a recurrent neural network (RNN). The RNN architecture allowed the use of the past output as input to predict the subsequent output. Notably, one type of RNN known as the long short-term memory (LSTM) network can handle long-term dependencies, such as those that exist in text summarization.
Scientists found inspiration for convolutional neural networks (CNN) from the architecture of the brain's ventral visual stream. In RL, an intelligent agent like a computer understands the state of the environment to dictate the action. Moreover, the computer learns to repeat some tasks based on rewards and avoid others based on penalties.
In deep RL, a neural network provides a gradient-descent-based nonlinear mapping between the current states of the environment and all feasible actions. Although deep RL has enabled the machine recognition of sounds, text, and images, this technology consumes immense computing resources.
As an alternative, spiking neural networks (SNNs) are being developed, as they work like biological neurons and are more energy efficient. SNNs transmit information in the form of an electric signal to another artificial neuron when its membrane potential hits a particular threshold.
How is AI used to study neuroscience?
Several AI-assisted brain computer/machine interface (BCI) applications have been developed to help people with neuromuscular disorders, such as cerebral palsy or spinal cord injuries. Furthermore, AI has been widely used for controlling prostheses, as demonstrated by BrainGate, which is an implant that allows users to control limb movements.
Detecting neurological infections like meningitis is tedious due to their wide range of symptoms. However, AI-based approaches using various predictor variables such as cerebrospinal fluid (CSF) neutrophils, lymphocytes, and neutrophil-to-lymphocyte ratio (NLR) could predict the type of meningitis with high precision. AI is also associated with several benefits when applied to neuro-oncology, as it can potentially provide precise initial diagnoses and therapeutic options.
Conclusions
The current study highlighted the power of AI models in neuroscience, despite the exceptional size, scope, and architecture of brain data.
The researchers also discuss existing challenges and emphasize the need for a multidisciplinary approach using AI, neuroscience, and systems biology to create interlinked datasets. Such an approach could provide an in-depth understanding of structures and cognitive functions, thereby increasing the efficiency of AI models at the clinical assessment level.
Importantly, there remains an urgent need for new standards within existing regulations to assess the safety of AI systems.
Journal reference:
- Surianarayanan, C., Lawrence, J. J., Chelliah, P. R., et al. (2023). Convergence of Artificial Intelligence and Neuroscience towards the Diagnosis of Neurological Disorders—A Scoping Review. Sensors 23(3062). doi:10.3390/s23063062