In a recent article published in the journal Nature, researchers developed EVEscape, a tool to forecast which severe acute respiratory syndrome coronavirus 2 (SARS-CoV-2) strains have the highest potential to escape host immunity.
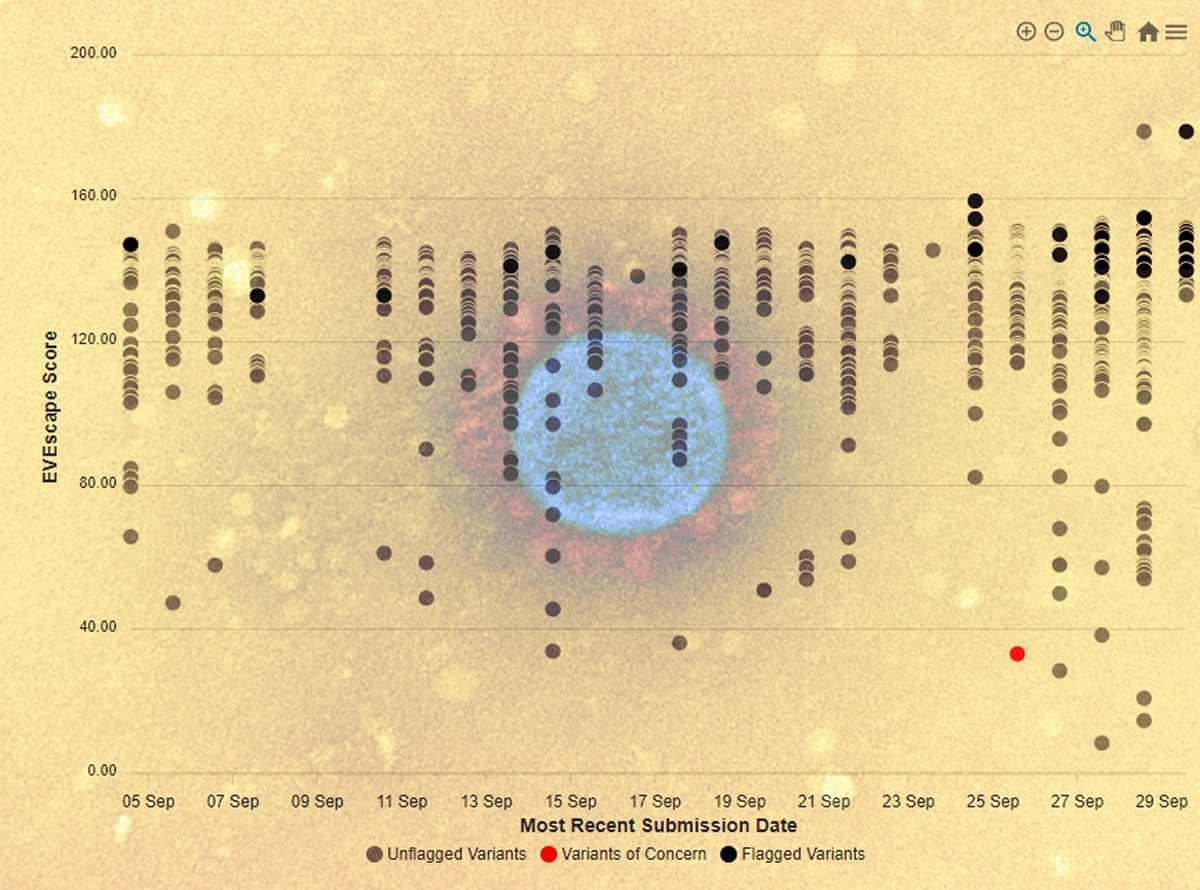
Study: Learning from prepandemic data to forecast viral escape. Image Credit: NAID and EVEscape
Background
Notably, three factors determine a viral variant's immunity evading potential: a mutation favoring viral fitness, disrupting antibody binding, and occurring in a region accessible to neutralizing antibodies.
However, given that viruses with pandemic potential (e.g., SARS-CoV-2) mutate rapidly, testing all their variants as they emerge is tedious, even when not considering the potential effects of all the mutations on their circulating strains.
Nonetheless, viral evolution, which gives rise to antibody escape mutations, affects a virus's reinfection rates and the duration of vaccine effectiveness. Thus, timely detection of viral variants with immune evasion potential is crucial to developing vaccines and therapeutics that effectively combat them.
EVScape working mechanism
EVscape used a method called EVE20 to predict the effects of substitution mutations in viral proteins on its fitness, i.e., sustained ability to replicate within the host and cause infection.
To do so, EVE20 learned the constraints from different aspects of viral protein function, such as protein expression, folding, and host receptor binding that governed structural integrity and functionality of all immunogenic viral proteins (antigens), e.g., SARS-CoV-2 spike (S) trimer glycoprotein. It also considered dependencies across various positions (epistasis) to capture the effects of mutations amid gradual viral strain background diversification from the ancestral strain. Exceptionally, EVEscape captured relevant epistasis and predicted mutant fitness for all viral strain backgrounds.
Further, EVEscape addressed the weaknesses of previous experimental methods, e.g., pseudovirus assays and deep mutational scans (DMSs), with limited ability for early prediction of immune escape. So, when the researchers evaluated EVE20's performance between experimental replicates (for human immunodeficiency virus [HIV], influenza, and SARS-CoV-2) using the Spearman correlation (ρ) and compared it with data from DMS experiments, its predictions moderately correlated with DMS data, with ρ values of 0.45 and 0.26 for protein expression and host receptor binding, respectively.
The second EVScape component, called antibody accessibility, identified potential antibody binding sites. It computed the accessibility of each amino acid residue, i.e., the likelihood of antibodies recognizing a mutated viral protein, using its negatively weighted amino acid residue(s)-contacts across three-dimensional structures accounting for protrusion from the core and conformational flexibility.
Furthermore, EVScape computed dissimilarity, i.e., the ability of the mutated viral protein to disrupt the binding of polyclonal antibodies, using variations in hydrophobicity and charge, which affect protein-protein interactions and is another effective metric of correlation with experimentally measured within-site escape.
EVScape predicted pandemic variations early on
The researchers trained EVScape on pre-pandemic data and then tested its capacity to make early predictions about immune evasion before these escape mutations occurred.
Within the whole S, EVEscape scores were biased towards the receptor-binding motif of the receptor-binding domain (RBD) and the neutralizing supersite in the N-terminal domain (NTD), implying it identified the most immunogenic domains of viral antigens without prior knowledge of any antibody epitopes.
EVScape accuracy in comparison to experimental methods
Despite being trained on historical viral genome sequences, EVEscape was as accurate as the latest DMS scans at anticipating pandemic variation (50% vs. 46%), even when considering the top decile of prediction.
EVEscape adaptations
The researchers replaced the EVE fitness component with TranceptEVE, a protein large language (LLM) model, with a remarkable ability to predict the effects of mutations, including insertions and deletions (indels) on SARS-CoV-2 S immune escape.
This model captured the most frequent indels at site 144 and in the top decile of random and pandemic indel predictions. They retrained EVE models with the addition of 11 million novel sequences, and even this model captured epistatic shifts between Wuhan and BA.2 strains.
EVEscape predictions for potential pandemics. Site-maximum EVEscape scores for Nipah Virus fusion protein (left) and Glycoprotein (right) depict regions of high EVEscape scores. Known escape mutations with experimental evidence (little is known about this understudied virus with pandemic potential) are highlighted with spheres.
Strain Forecasting
Furthermore, EVEscape identified strain-level escape mutation predictions for SARS-CoV-2 with consistently high accuracy. EVEscape scores were higher for more recent variants, reflecting their increased propensity for immune escape; accordingly, scores for Delta and Omicron were in the top 1% against pools of variants with random combinations of mutations.
Overall, EVEscape could rank emerging strains for their escape potential, highlighting the most concerning viral variants early for experimental characterization and incorporation into vaccine boosters.
Conclusions
To conclude, EVScape combined a deep generative model trained on historical (pre-pandemic) SARS-CoV-2 sequences (from sarbecoviruses to seasonal coronaviruses) with structural and biophysical information to predict immune evasion likelihood for unseen or yet-to-occur mutations in all viral antigenic proteins, e.g., SARS-CoV-2 spike (S) which, in turn, could facilitate timely vaccine and therapeutic design. Moreover, it did not rely on recent pandemic sequencing or antibodies (or sera), which became available when many people got infected or received vaccination.
Moreover, it adapted to multiple viruses; thus, researchers could validate its use for HIV, influenza, SARS-CoV-2, and some understudied viruses with pandemic potential, e.g., Nipah and Lassa. However, according to the researchers, EVScape will be best leveraged in synergy with experiments that measure immune evasion and when trained on enhanced pandemic surveillance data as it becomes available.
Journal reference:
- Thadani, N. N., Gurev, S., Notin, P., Youssef, N., Rollins, N. J., Ritter, D., Sander, C., Gal, Y., & Marks, D. S. (2023). Learning from prepandemic data to forecast viral escape. Nature, 1-8. doi: https://doi.org/10.1038/s41586-023-06617-0, https://www.nature.com/articles/s41586-023-06617-0