One of the main challenges that all pharmaceutical companies face is how to boost the probability of designing new drugs that will successfully make it to market. The drug discovery pipeline is wrought with difficulties that ultimately result in less than 12% of drugs making it to market as part of a development process that often takes over ten years.1
Image Credit: Zyabich/Shutterstock
There are several bottlenecks in the drug development process, such as working out the mode of action of a new therapeutic and its molecular targets and identifying off-target effects. This work requires extensive laboratory hours, numerous assays, and testing and is highly time-consuming. Even if both laboratory and animal tests prove promising, any candidate will face numerous regulatory and safety hurdles before further testing can be approved.
The high-risk and labor-intensive nature of early-stage drug development is why drug repurposing, the process by which an already approved drug is used for another purpose, is often considered an attractive alternative.2 Another approach is accelerating parts of the drug discovery process by combining automation and analytical technologies.
Automation in Pharmaceuticals
The uptake in automation surged across the chemical and life science fields as part of the ‘Industry 4.0 revolution’. Targets for automation in the pharmaceutical industry include the replacement of repetitive bench experiments with robotics and using automated sampling and analysis of analytical experiments.
Recent work at AstraZeneca, which will be presented at Pittcon, a leading international laboratory science conference, has demonstrated how automation can help boost the efficiency of pharmaceutical analysis for preclinical and clinical drug candidates. Alongside this, the company has been automating the preparation and management of samples to enhance efficiency in evaluating the developability of drug compounds.
Other work from GlaxoSmithKline has focused on how automation, in partnership with machine learning, can create a combination of high throughput analytics with automated data analysis for a complete sample analysis pipeline. With a greater diversity of chemical species being used in drugs, such pipelines need to be able to deal with a greater number of sample types.
One of the challenges preventing the uptake of automation in pharmaceuticals is the interdisciplinary nature of the problem. Expertise in traditional chemometrics analysis is required, as well as advanced instrumentation, potentially new robotics or sampling methods, and knowledge of the most recent developments in machine learning and artificial intelligence approaches.
The wide-ranging nature of the required knowledge is why conferences like Pittcon are so critical. Such conferences can help address the open challenges in this research field by bringing experts across a broad range of disciplines for both keynote talks and discussion sessions.
Computational Analysis
The appeal to replace lab-based elements in drug discovery and development with computational calculations is largely driven by costs. With ever-greater amounts of computational power becoming available, as well as improvements in the efficiencies of algorithms, calculations on large and complex biosystems are becoming increasingly tractable.
The complex biological environments a potential drug molecule will experience are incredibly difficult to model to an extremely high degree of precision. This means that a pure ab initio computational approach is often unfeasible for drug development and testing as there is insufficient confidence in the computational results to allow the lab experiments to be fully replaced.
Teams of researchers at GlaxoSmithKline have therefore been adopting a hybrid approach inspired by biological screening approaches.3 Using multi-well plates to perform automated pH-solubility measurements – critical parameters for whether or not a drug is likely to work – alongside analytical methods like HPLC, the team then automated the analysis of the pKa values and logD parameters to create a complete high-throughput scheme.
However, for preclinical drug screening, computational approaches are more widely used.4 Simulations using methods such as molecular dynamics can be used to evaluate the ADMET properties that indicate how the pharmaceutical compound will behave when ingested in the body. Either known structures from existing libraries can be used for evaluation, or simulations can also be used to investigate a range of theoretical structures that might bind with the biological target of interest.
Automation of analytical instrumentation will allow researchers to screen wide numbers of compounds more rapidly and provide experimental data that can be used in the QSAR models that are often used with calculated datasets.
Important developments in improving the throughput for automated pharmaceutical screening have helped advance current powder handling technologies.5 Dispensing accurate masses of powders has been challenging to automate and achieve good repeatability and accuracy. Still, several commercial platforms are viable technologies for this, opening a range of possibilities for automated synthesis and analysis platforms.
To find out how you can register for Pittcon and details on the event itself, visit the homepage, which can be found here. Information on the conference schedule and details of all speakers can be found in the Technical Program.
References and Further Reading
- Congressional Budget Office (2021) Research and Development in the Pharmaceutical Industry, https://www.cbo.gov/publication/57126, accessed January 2023
- Strittmatter, S. M. (2014). Overcoming drug development bottlenecks with repurposing: Old drugs learn new tricks. Nature Medicine, 20(6), 590–591. https://doi.org/10.1038/nm.3595
- Bahr, M. N., Nandkeolyar, A., Kenna, J. K., Nevins, N., Da Vià, L., Işık, M., Chodera, J. D., & Mobley, D. L. (2021). Automated high throughput pKa and distribution coefficient measurements of pharmaceutical compounds for the SAMPL8 blind prediction challenge. Journal of Computer-Aided Molecular Design, 35(11), 1141–1155. https://doi.org/10.1007/s10822-021-00427-0
- Wu, F., Zhou, Y., Li, L., Shen, X., Chen, G., Wang, X., Liang, X., Tan, M., & Huang, Z. (2020). Computational Approaches in Preclinical Studies on Drug Discovery and Development. Frontiers in Chemistry, 8,726 https://doi.org/10.3389/fchem.2020.00726
- Bahr, M. N., Morris, M. A., Tu, N. P., & Nandkeolyar, A. (2020). Recent advances in high-throughput automated powder dispensing platforms for pharmaceutical applications. Organic Process Research and Development, 24(11), 2752–2761. https://doi.org/10.1021/acs.oprd.0c00411
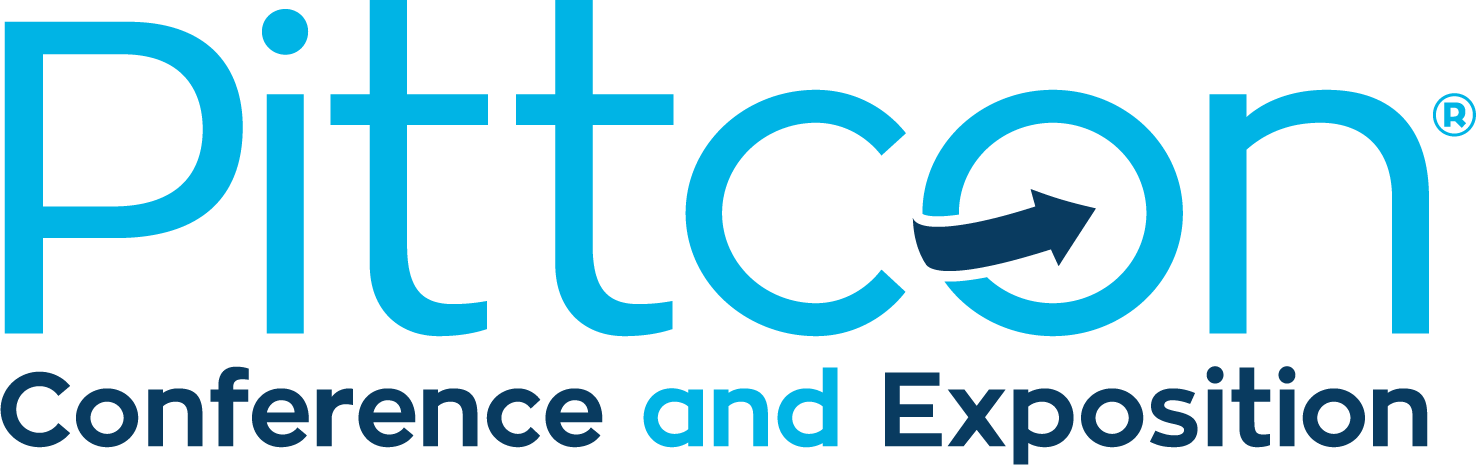
About Pittcon
Pittcon® is a registered trademark of The Pittsburgh Conference on Analytical Chemistry and Applied Spectroscopy, a Pennsylvania non-profit organization. Co-sponsored by the Spectroscopy Society of Pittsburgh and the Society for Analytical Chemists of Pittsburgh, Pittcon is the premier annual conference and exposition on laboratory science.
Proceeds from Pittcon fund science education and outreach at all levels, kindergarten through adult. Pittcon donates more than a million dollars a year to provide financial and administrative support for various science outreach activities including science equipment grants, research grants, scholarships and internships for students, awards to teachers and professors, and grants to public science centers, libraries and museums.
Visit pittcon.org for more information.
Sponsored Content Policy: News-Medical.net publishes articles and related content that may be derived from sources where we have existing commercial relationships, provided such content adds value to the core editorial ethos of News-Medical.Net which is to educate and inform site visitors interested in medical research, science, medical devices and treatments.