A study posted recently to the bioRxiv* preprint server demonstrated that the emergence of new spike variants of severe acute respiratory syndrome coronavirus-2 (SARS-CoV-2) can be predicted.
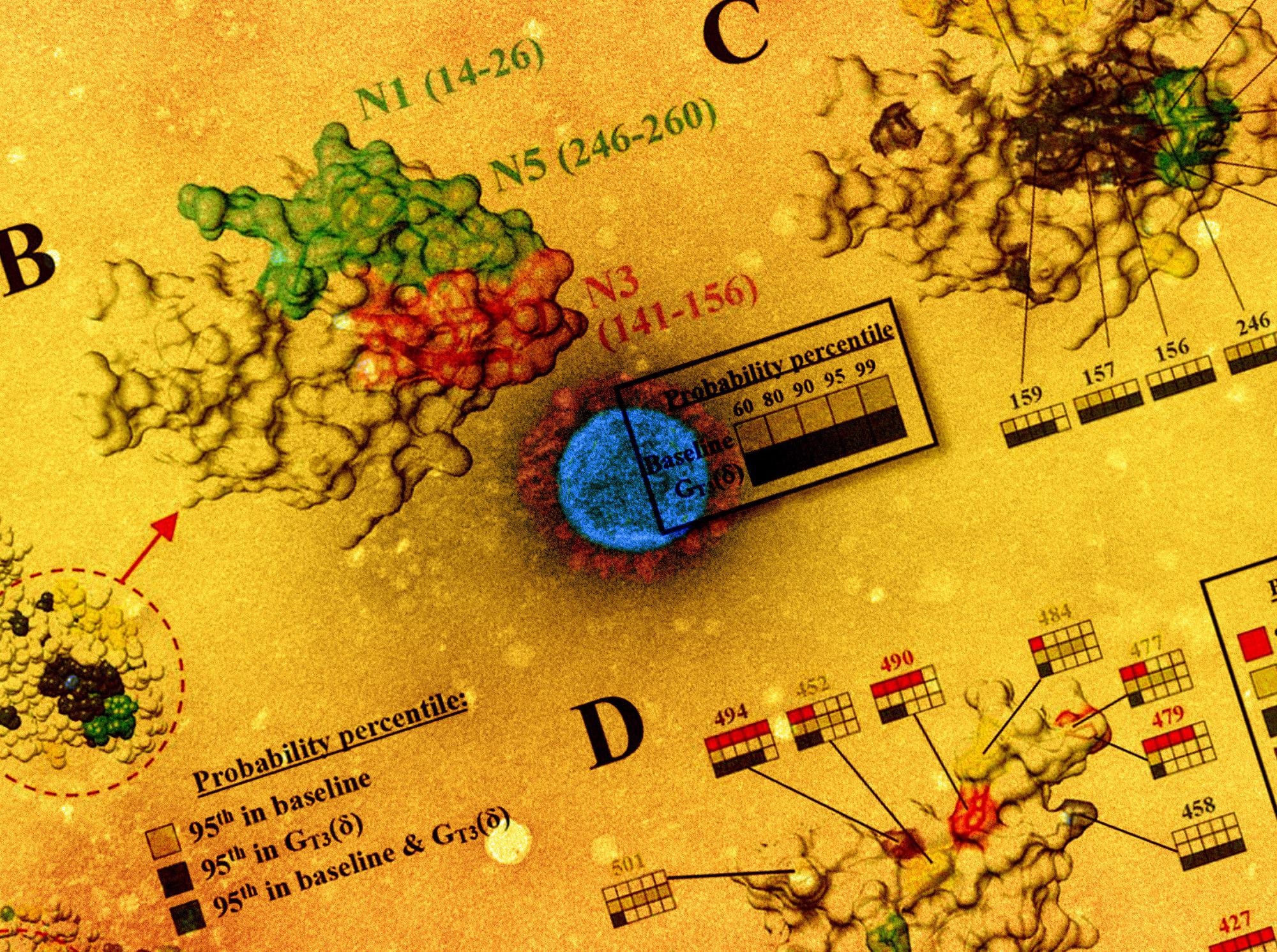
Study: Patterns of Volatility Across the Spike Protein Accurately Predict the Emergence of Mutations within SARS-CoV-2 Lineages. Image Credit: NIAID

*Important notice: bioRxiv publishes preliminary scientific reports that are not peer-reviewed and, therefore, should not be regarded as conclusive, guide clinical practice/health-related behavior, or treated as established information.
Throughout the coronavirus disease 2019 (COVID-19) pandemic, several SARS-CoV-2 variants have emerged. New variants present novel characteristics that may enhance their infectivity, transmissibility, and clinical disease severity and decrease the sensitivity to the vaccine or infection-induced immune responses. One such example is the latest SARS-CoV-2 variant, the Omicron, which has over 35 mutations and has rapidly spread across the globe, leading to a record-high number of infections.
Reports suggest that SARS-CoV-2 Omicron has evolved to a greater extent than previous variants, and it exhibits immune-evasive traits, increased transmissibility, and resistance to available vaccines and therapeutics. Further, as SARS-CoV-2 mutates and evolves, more variants might appear in the future. Therefore, research is required to predict the emergence of new variants to proactively design countermeasures against them.
The study
In the current study, researchers investigated whether new variants/mutations of the SARS-CoV-2 spike (S) protein could be forecast by studying the amino acid (aa) variability patterns in small virus clusters that chronologically and phylogenetically precede such events. Sequences of the spike from the initial phases of the COVID-19 pandemic were grouped into small clusters and the authors calculated 1) the extent of aa variability, 2) the aa variability at adjacent sites in the 3D structure of the S protein, and 3) positions where aa variability co-occurred with the site of interest.
The team obtained over 600,000 sequences of the S gene, filtered them, and identified around 16,808 unique S sequences. A maximum-likelihood tree was generated, and the tree was divided into groups with a distance of 0.004 nucleotide substitutions per site. A threshold of 0.0015 nucleotide substitutions per site was set to distinguish between baseline and terminal emergent groups. The sequences in baseline groups were clustered into subsets of 50 sequences and every site in S was assessed for the presence or absence of variability and a mean variability score i.e., volatility, was assigned for each position.
The researchers described two types of emerging mutations - 1) a mutation that is observed in the ancestral sequence of the group and at least in 50 % of the sequences in that cluster was termed as a group-dominant mutation or GDM and 2) a mutation which is not seen in the group ancestor and found in <50 % of group sequences was defined as a subgroup-emerging mutation (sGEM).
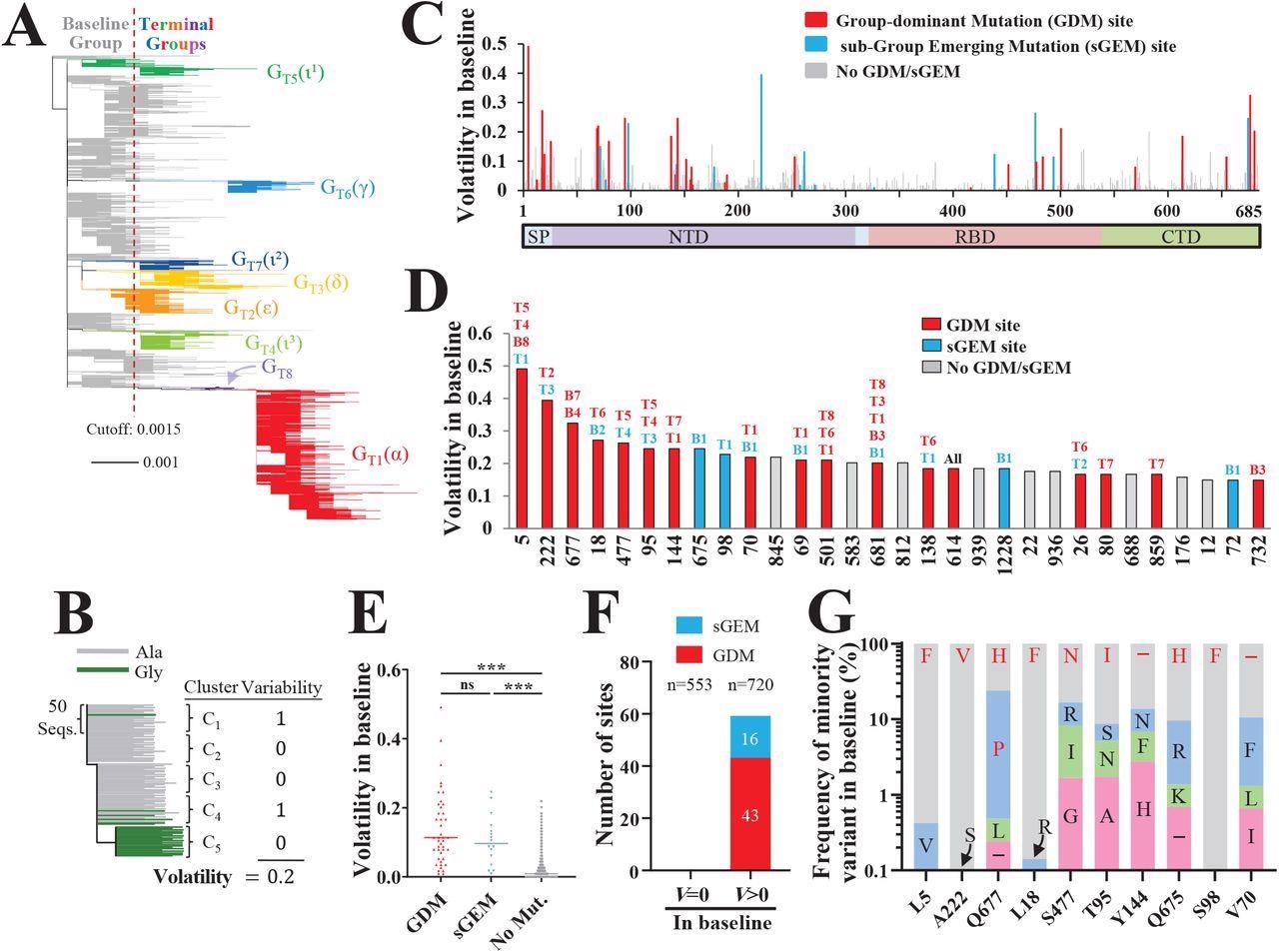
Spike positions with high volatility appear as sites of group-dominant or subgroupemerging mutations. (A) Phylogenetic tree based on 16,808 unique spike sequences. Terminal groups are colored and labeled, with their WHO variant designations in parentheses. (B) Schematic of our approach to calculate volatility for each position of spike. (C) Volatility values for all positions of spike subunit S1, calculated using the 114 baseline clusters (see values for S2 subunit in Figure S1C). (D) Thirty spike positions with the highest volatility values. The baseline (“B”) or terminal (“T”) groups that contain mutations at these positions are indicated. (E) Comparison of volatility values for spike positions that emerged with a GDM, sGEM or no such mutations. P-values in an unpaired T test: ***, P<0.0005; ****, P<0.00005; ns, not significant. (F) Number of sites that appeared with GDMs and sGEMs when volatility (V) in the baseline group was zero or larger than zero. The number of site in each subset (n) is indicated. (G) Frequencies of minority variants (nonancestral residues) at the ten positions of spike with the highest volatility values (see panel D). Frequencies are expressed as a percent of all sequences with a non-ancestral residue at the indicated position. The residues that emerged as GDMs or sGEMs are indicated in red font.
Results
The authors identified around 43 GDMs and 16 sGEMs across both the terminal and baseline groups, and notably, the sites with high volatility scores resulted in GDMs and sGEMs. The sites of GDMs and sGEMs were found to be more volatile (with high mean variability score) than other positions without such emerging mutations. It was noted that high positional volatility in the baseline group could phylogenetically precede the appearance of GDMs and sGEMs in the terminal group.
The baseline volatility scores of each aa were mapped onto the 3D structure of the S protein which revealed various clusters with high volatile sites particularly in the spike's N-terminal domain (NTD). The authors noted that the presence of a volatile site adjacent to these (mapped) sites increased their likelihood of volatility, suggesting that a highly volatile environment in the vicinity could influence the occurrence of new volatile sites.
Furthermore, the authors investigated the factors governing the emergence of a mutation as GDM and sGEM. A co-volatility network was constructed using P-values of Fisher's exact test that quantified the co-occurrence of volatile sites at any two S sites. They observed that the existence of high volatility, for any site in the S protein, at its network-associated position increases its odds to emerge as GDM or sGEM.
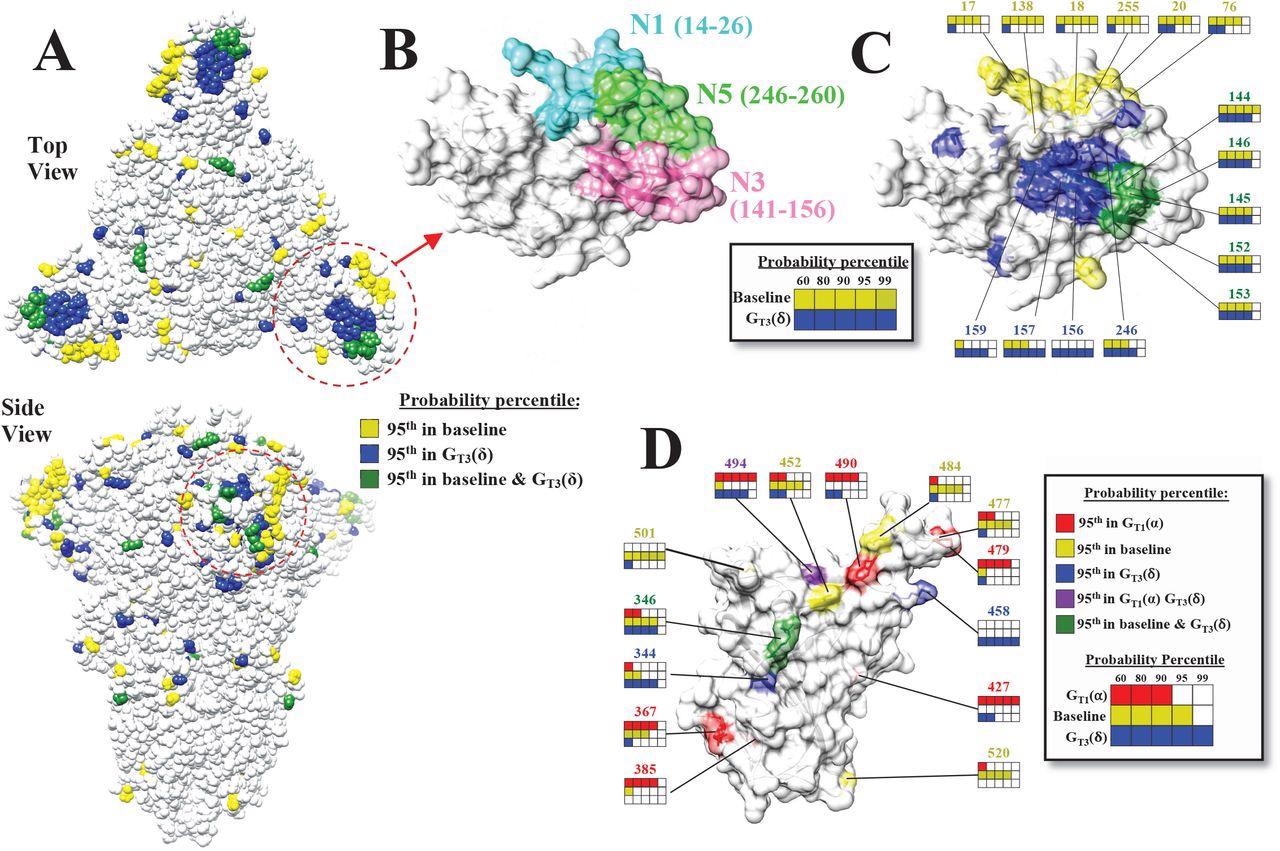
The mutational landscape of the SARS-CoV-2 spike is lineage-specific. (A) Distribution on the SARS-CoV-2 spike trimer (PDB ID 6ZGI) of positions with mutation probabilities in the 95th percentile, as calculated using sequences from the baseline and GT3(δ) groups. (B) Top view of the NTD supersite of neutralization, highlighting the N1, N3 and N5 loops and the residues that compose them. (C) Same view as in panel B. Spike positions with probabilities in the 95th percentile are colored as in panel A. The probability percentiles assigned to each position by the baseline group and by the GT3(δ) group are compared. (D) Side view of the RBD showing positions with mutation probabilities in the 95th percentile in at least one of the indicated groups.
Sequences from the early periods of the pandemic (December 2019 to September 2021) were used as early-phase sequences to forecast the emergence of new mutations (lineage-defining mutations or LDMs) in lineages that appeared between October 2020 and June 2021. Volatility profiles were evaluated, which showed that the high volatility at any position of S protein and its network- and spatial-associated sites temporally preceded the emergence of lineage-defining mutations in the population. Using this model, the authors were able to identify Omicron-mutations in the samples collected from March 2021 until November 2021.
Conclusions
The evolution of SARS-CoV-2 into newer variants is alarming as they present with enhanced pathogenic traits undermining the scope of current vaccines and therapeutics. Consequently, there is a growing demand for the development of variant-specific vaccines to address the emergent challenges. However, nucleic acid-based vaccines can be modified to be specific to SARS-CoV-2 variants but require additional clinical studies to test their safety and efficacy, which is time-consuming.
Therefore, tools to forecast the emergence of new mutations are required, and the present study has demonstrated a simple framework for such early predictions. Based on an early forecast of imminent mutations, designing immunogens specific to the lineages could be beneficial to mitigate the COVID-19 pandemic.

*Important notice: bioRxiv publishes preliminary scientific reports that are not peer-reviewed and, therefore, should not be regarded as conclusive, guide clinical practice/health-related behavior, or treated as established information.
Journal reference:
- Preliminary scientific report.
Patterns of Volatility Across the Spike Protein Accurately Predict the Emergence of Mutations within SARS-CoV-2 Lineages, Roberth Anthony Rojas Chávez, Mohammad Fili, Changze Han, Syed A. Rahman, Isaiah Guzman L Bicar, Guiping Hu, Jishnu Das, Grant D. Brown, Hillel Haim, bioRxiv, 2022.02.01.478697; DOI: https://doi.org/10.1101/2022.02.01.478697, https://www.biorxiv.org/content/10.1101/2022.02.01.478697v1