A new study posted to the medRxiv* preprint server applies MAGICAL for identifying modulated host response regulatory circuits in response to Staphylococcus aureus. S. aureus is a bacteria that is mainly resistant to traditional antibiotics; therefore, infection with this pathogen is often severe and associated with high mortality rates. As a hierarchical Bayesian approach, MAGICAL maps disease-associated transcription factors, chromatin sites, and genes as regulatory circuits based on paired RNA-seq and ATAC-seq data.
The team of researchers from across the United States also aimed to differentiate the circuit responses to methicillin-susceptible (MSSA) and methicillin-resistant S. aureus (MRSA).
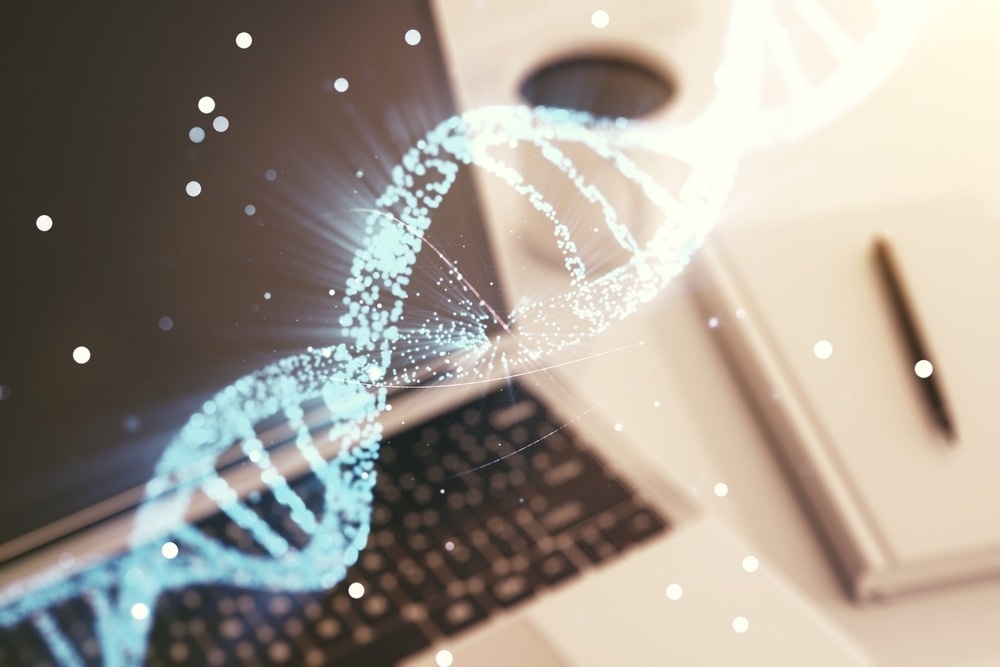
Study: Mapping disease regulatory circuits at cell-type resolution from single-cell multiomics data. Image Credit: Pixels Hunter / Shutterstock.com

*Important notice: medRxiv publishes preliminary scientific reports that are not peer-reviewed and, therefore, should not be regarded as conclusive, guide clinical practice/health-related behavior, or treated as established information.
What is MAGICAL?
Regulatory circuits can be formed by downstream target genes, transcription factors, and chromatin regulatory domains. Dysregulation of these circuits occurs in specific cell types during disease states.
The impact of disease on regulatory circuits can be determined by mapping regulatory domains with chromatin accessibility changes to altered gene expression concerning cell-type resolution and genomic looping.
Identifying differentially expressed genes and differential chromatin sites within individual cell types has improved with the help of single-cell data that characterize disease states. However, current approaches cannot resolve high-resolution regulation changes within individual cell types, thus preventing determining regulatory circuits affected by disease or different disease states.
To overcome these limitations, Multiome Accessibility Gene Integration Calling And Looping (MAGICAL) was developed to model gene expression variation and chromatin accessibility to identify circuits that differ between conditions.
MAGICAL introduces hidden variables to model noise and signal scATAC-seq and scRNA-seq data. Moreover, MAGICAL reconstructs regulatory circuits at the cell-type resolution, as they are cell-type specific.
About the study
The current study included 21 patients with culture-confirmed S. aureus bloodstream infection, of which 11 were MSSAs and 10 were MRSA. Twenty-three healthy controls of the same age group as the patients were also included.
Peripheral blood mononuclear cells (PBMCs) obtained from the participants’ blood samples were thawed, followed by nuclei isolation. After that, S. aureus scRNA-seq and scATAC-seq data generation, data integration, and cell type annotation were performed.
The selection of differentially accessible chromatin sites (DAS) and differentially expressed genes (DEG) was performed under contrast conditions. MAGICAL constructed regulatory circuits by searching 870 human motifs.
Subsequently, MAGICAL was applied to a 10X PBMC single-cell multiome dataset and a GM12878 cell line SHARE-seq dataset for benchmarking. MAGICAL was also applied to a public PBMC coronavirus disease 2019 (COVID-19) single-cell multi-omics dataset to analyze disease-associated circuit genes and chromatin sites.
Validation of chromatin sites associated with mild COVID-19 was based on PBMC scATAC-seq data from a separate COVID-19 cohort study. In addition, information on Promoter-capture HiC interactions detected in B-cells, CD8 T-cells, CD4 T-cells, and CD14 monocytes, as well as HiCHIP interactions, was obtained.
This was followed by an assessment of the enrichment of genome-wide association studies (GWAS) loci of inflammatory diseases, which are located at circuit chromatin sites in each cell type. Finally, circuit genes were selected to predict S. aureus infection and antibiotic sensitivity.
Study findings
MAGICAL-inferred linkages between genes and chromatin sites correspond highly to experimental 3D chromatin interactions. The circuit genes also accurately determined different disease states.
MAGICAL outperformed both TRIPOD on the application to a 10X multiome single-cell dataset and FigR on the application to a GM12878 SHARE-seq dataset. Moreover, the elimination of topologically associated domains (TAD) did not impact the performance of MAGICAL.
MAGICAL was also capable of determining the regulatory circuits of severe and mild COVID-19 groups separately. Furthermore, MAGICAL accurately determined chromatin sites and genes modulated by severe and mild COVID-19.
This approach selected high-confidence regulatory circuits in each cell type for three contrasts, which included MRSA vs. MSSA, MSSA vs. control, and MRSA vs. control. Moreover, CD14 monocytes, known to play an essential role in response to S. aureus, had the highest number of regulatory circuits in MAGICAL.
MAGICAL identified activating protein 1 (AP-1) complex proteins as the most important regulators in CD14 monocytes. Results from the enrichment analysis also indicated cytokine signaling to be mediated by AP-1 factors, along with inflammatory responses in the macrophages to be most enriched.
MAGICAL modeled regulatory effects of distal and proximal regions on circuit genes and enriching circuit genes with epi-genes. MAGICAL also identified regulatory processes crucial for the host response to S. aureus sepsis and determined regulatory differences in the host immune response that were closely related to MSSA and MRSA infections.
Conclusions
The current study demonstrates that MAGICAL is an important single-cell multi-omics data analysis tool. This method can perform contrast-condition and single-condition analysis, thus providing a high-resolution approach for systematically studying the regulatory control mechanisms of different disease conditions.

*Important notice: medRxiv publishes preliminary scientific reports that are not peer-reviewed and, therefore, should not be regarded as conclusive, guide clinical practice/health-related behavior, or treated as established information.