In this interview, industry experts Johanna Uhari-Väänänen and Timo Bragge discuss how machine learning enhances behavioral analysis, drug discovery, and MRI segmentation in neuroscience research, improving accuracy, efficiency, and scalability.
Could you please introduce yourselves?
Johanna Uhari-Väänänen:
My name is Johanna Uhari-Väänänen, and I'm a group leader in Neuropharmacology at Charles River Labs. My previous research experience has focused on addiction and neurodegenerative diseases. Now, as an in vivo scientist and study director, I focus on preclinical central nervous system (CNS) disease models and their application in our clients' drug discovery projects.
Timo Bragge:
I am Timo Bragge, a senior data scientist at Charles River Labs. I have a background in medical physics, particuarly motion analysis and medical imaging. My work revolves around designing advanced data analysis systems for preclinical CNS research, particularly focusing on behavioral studies and machine learning applications.
Can you explain why machine learning is useful in behavioral science?
Johanna Uhari-Väänänen:
Behavioral tests used in neuroscience research and drug discovery often requires researchers to analyze specific behaviors and animal movement. Traditionally, this would be done via video recording, then manual annotation and scoring for selected behaviours or movement. Obviously this method is very time-consuming and prone to human error and bias.
Machine learning, on the other hand, allows us to automate this process. It can objectively classify behaviors, reduce researcher variability, and provide faster and more reliable results. Machine learning can even handle long-duration monitoring, like tracking behaviors over a 24-hour circadian cycle.
Machine learning can be applied in the analysis of various behviour tests and assessments, including learning and cognition tests such as the Morris water maze, motor function assessments, and even detecting subtle abnormalities such as seizures or freezing. Essentially, every test that benefits from animal tracking can use machine learning based analysis.

Image Credit: Anucha Tiemsom/Shutterstock.com
Could you please describe in more detail what machine learning is?
Timo Bragge:
Machine learning is a tool that teaches computers how to learn from data without being explicitly programmed. Unlike manual processing of behavior data, which can be inconsistent, machine learning ensures that the same input consistently produces the same output. This reliability becomes crucial when scaling up to large datasets, and enables parallel processing to reduce the time needed for complex data analysis.
Our work often uses Convolutional Neural Networks (CNNs), which are particularly useful for image processing. These networks break down images into smaller, manageable components and then apply filters to detect patterns, moving from simple shapes to more complex structures as you go deeper into the network.
For instance, we use DeepLabCut software, which relies on CNNs, to track animal behavior in a process known as supervised learning. We first provide the algorithm with known data for the behavior we want to identify by annotating key frames in videos, by marking specific points on the animal’s body, like its nose, ears, or tail. The software then learns to track these points to determine behaviors, and this can be applied automatically across new video data.
Can you explain how machine learning can help the detection of behaviors in neuroscience drug discovery?
Johanna Uhari-Väänänen:
Using the method Timo describes we can quickly and accurately use machine learning to analyze behaviors in a variety of different test paradigms. For example, a balance beam walking test is commonly used in our labs to look at changes in gait, balance and limb movements in movement or neuromuscular disorders such as Parkinson's disease. A mouse walks along a beam, next to a mirror so all limbs can be observed, and is recorded on video. We then train an algorithm to detect limb placement, and can compare minute changes in placement and gait across control groups, disease models and treatment with novel compounds. The use of machine learning allows us to greatly decrease the time taken for analysis, and to detect very small changes that would be hard to recognise with the naked eye.
Another application of machine learning that we have explored is the detection of social interactions between mice in the 3-chamber social interaction test. This test is commonly used in drug discovery for conditions where social behaviors may be impaired, for example autism spectrum disorders.
The experimental set up gives mice a choice of spending time with either a novel mouse or a novel object, with time spent interacting with another mouse measured to determine sociability. Using machine learning allows us to go beyond just detection of basic interaction, delving deep into more specific behaviors like exploration, nose-to-nose interactions, nose-to-tail and following.
How is Machine Learning used in MRI segmentation?
Timo Bragge:
Magnetic Resonance Imaging (MRI) is a translational and non-invasive imaging technique that we use routinely in drug discovery projects to examine brain anatomy and structure, brain atrophy in neurodegeneration, lesion formation, white matter density, vascular changes, and drug responses. It is a highly valuble tool but it generates huge quantities of data that need to be processed to get the decision-making data for our clients.
Although some manual correction may still be needed, the automation provided by machine learning and CNNs significantly speeds up the entire process, allowing us to handle large datasets much more efficiently. This is especially valuable in studies where we must analyze scans taken at different time points in the same animal in longitudinal studies, for example. It also allows us to more accurately identify specific brain areas across different animals, and different groups ensuring consistency.
About Johanna Uhari-Väänänen
Johanna Uhari-Väänänen earned her PhD in pharmacology from the University of Helsinki in 2019, focusing on brain mechanisms involved in ethanol addiction. She then spent three years as a postdoctoral researcher at the University of Helsinki, studying traumatic brain injury, Alzheimer’s disease, and Parkinson’s disease. In 2022, she joined Charles River as an In Vivo Scientist, working with animal models for CNS-related conditions.
About Timo Bragge 
Timo Bragge holds an MSc in Medical Physics from the University of Eastern Finland. He became part of Charles River in 2016 and has played a key role in developing pipelines for advanced data analysis in preclinical research on animal models of CNS-related conditions. His work encompasses fine motor kinematics, behavior, and preclinical imaging. Currently serving as a Principal Data Scientist, Timo leads the data analysis team.
About Charles River Laboratories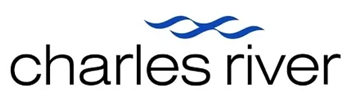
At Charles River, we are passionate about our role in improving the quality of people’s lives. Our dedicated team of preclinical neuroscience CRO scientists want the same thing as you do: to find a cure for the devastating diseases of the central nervous system. From basic research to regulatory approval, we have the leading science, range of services, and collaborative approach you need to discover and develop novel therapies.
We understand the challenges and complexities in the search of potential therapies for neurological disorders. The combination of our comprehensive neuroscience drug discovery services and expertise supports the creation of customizable, innovative and efficient solutions for your research. Our team of neuroscientists continues to establish the most relevant in vitro and in vivo models and assays of acute and chronic neurological diseases to help our partners identify and test new compounds in this challenging field.