Data is one of the most valuable assets your company possesses. This is particularly true for scientific data, which is the accumulation of many hours of parallel investment in resources, such as time and equipment.
The data collated can come in a number of forms, some of which are simple (such as a measurement of weight), and some of which are more complex (such as HPLC data).
Collecting and gaining insight from these whole data sets is a necessary task for improving workflows, ensuring quality records and troubleshooting issues with reproducibility. At present, there is mounting interest being shown in reliable data archival solutions.
The results of one study showed that two years after a publication, the likelihood of accessing raw data from a scientific study falls by 17%, and goes on to repeat this drop year on year.1 Scientists accept that the most significant cause of this is lost data, whether this is the loss of paper documentation or electronic media.
How should your company put in place reliable data management solutions to guarantee ongoing access to one of its most highly valued resources?
Introduction
Whichever method is employed by your team to capture raw data from your studies, some key factors to consider with data collection systems include:
- Does the approach preserve the integrity of the data?
- Is the data stored in a secure fashion?
- Are team members able to access the data readily?
- Is remote access to the data possible?
- Does the method meet compliance standards for FDA 21 CFR Part 11 in relation to electronic records?
- Does the data capture approach assist in meeting compliance with other regulatory standards, including the Food Safety Modernization Act (FSMA), Joint Commission on Accreditation of Healthcare Organizations (JCAHO), World Health Organization (WHO), as appropriate?
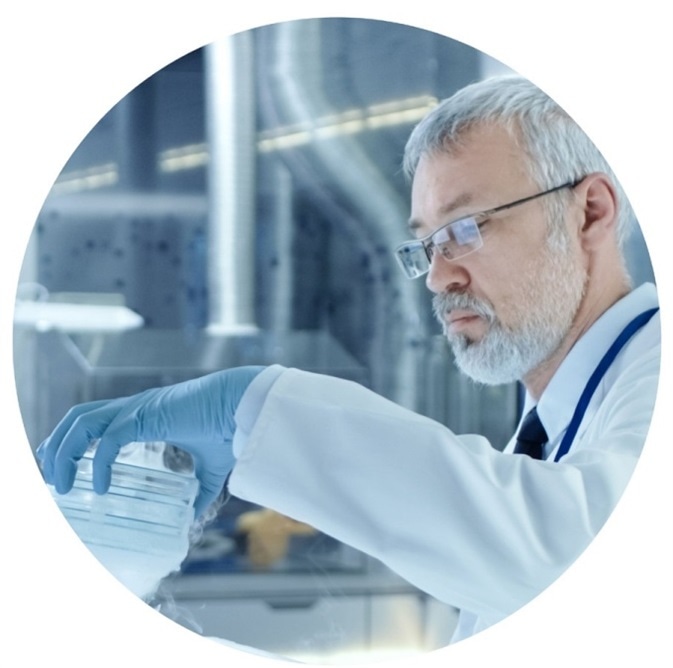
Four frequently employed methods for scientific data collection include:
- Manual entry into forms or notebooks
- Equipment-generated records
- Manual entry into electronic laboratory notebooks (ELN) or enterprise resource management (ERP) systems
- Wireless Internet of Things (IoT) add-on devices
This article will offer an outline of each method, how it is typically employed, and the benefits and drawbacks of each method.
Manual Entry into Forms for Notebooks
This is the simplest approach for logging scientific data and usually involves a technician or other specified person noting down the values that are output by a variety of types of scientific equipment.
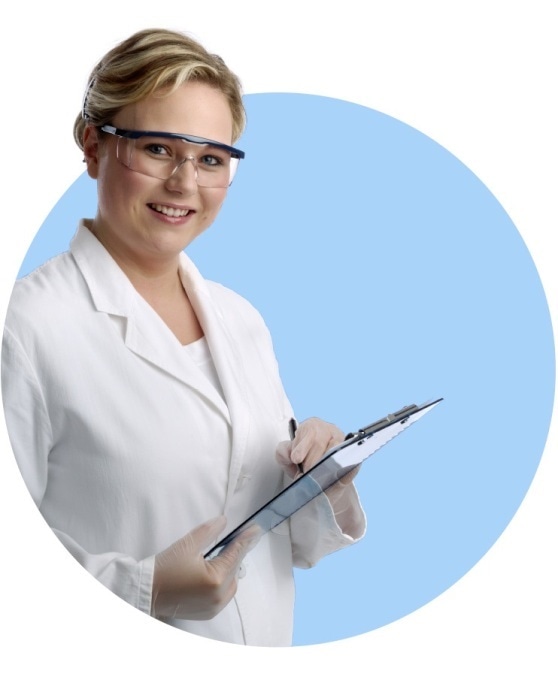
Forms or laboratory notebooks are used to manually log these readings, and thus the capital cost of this method is comparatively low. It is also possible to establish quality systems to accept forms and laboratory notebooks as official records.
Although this approach offers a simple way to record data, these are a number of concerns that make it a less desirable option, most significantly, its vulnerability to human error.
Laboratory workers are often absorbed by the time-sensitive nature of the task they are carrying out, and as such, may forget to note down equipment values, or even accidentally log inaccurate values or units of measurement, jeopardizing the integrity of the data.
Another issue with manual data collection is the security and accessibility of the data. Paper-based records in any form can be misplaced or permanently lost.
Furthermore, siloed data is also a concern. Records that are paper-based are not easily accessible by team members, and for those based outside of the office, remote access to the data is entirely impossible.
Metadata, including equipment settings, maintenance and calibration records are often missed out in manual data collection, resulting in incomplete records. Lastly, paper-based systems are simply not an appropriate method with which to capture large, complex data sets.
Pros
Overall, the pros of manual data collection are that it offers an easy, cost-effective and well-established method which may be compliant with quality systems.
Cons
The downsides of manual data collection are that it is the method most vulnerable to human errors and carries the risk of poor data security and data silos. It may also result in an incomplete data structure and is not well-suited to large, complex data sets.
Equipment-Generated Records
Another possible method of data capture is to print or export values and/or files from scientific equipment. Printed records are subsequently attached to forms or laboratory notebooks, while electronic files can be kept on USB memory sticks.
This process has an advantage over manual data entry into forms or notebooks in that it offers greater data integrity, since records are directly exported from the equipment itself. As is the case with manual entries, this process is highly-adaptable and records can be acknowledged as quality records.
However, although this method does address one issue from the manual entry approach, many of the other difficulties remain the same. The concern of poor data security remains unresolved, as paper-based records and USB memory sticks can still be lost or misplaced.
Additionally, when working within complex protocols, staff may forget to print or export data, again resulting in the loss of data. The issue of data silos also remains, as neither paper-based records nor USB memory sticks offer a suitable solution to this problem.
Finally, large, complex data sets still cannot be managed using this approach, particularly where paper-based records are in use.
Pros
Overall, the pros of equipment generated records are that it offers an easy, cost-effective and well-established method which may be compliant with quality systems and which reduces the risk of human error.
Cons
The downsides of this method are that it carries the risk of poor data security and data silos. It may also result in an incomplete data structure and is not well-suited to large, complex data sets.
Manual Entry into Electronic Laboratory Notebook (ELN) or Enterprise Resource Planning (ERP) System
For management of data collection and analysis, some organizations make use of Electronic Laboratory Notebooks (ELNs) or Enterprise Resource Planning (ERP) systems. With this process, it is possible to effectively eliminate some of the concerns raised with the previous approaches.
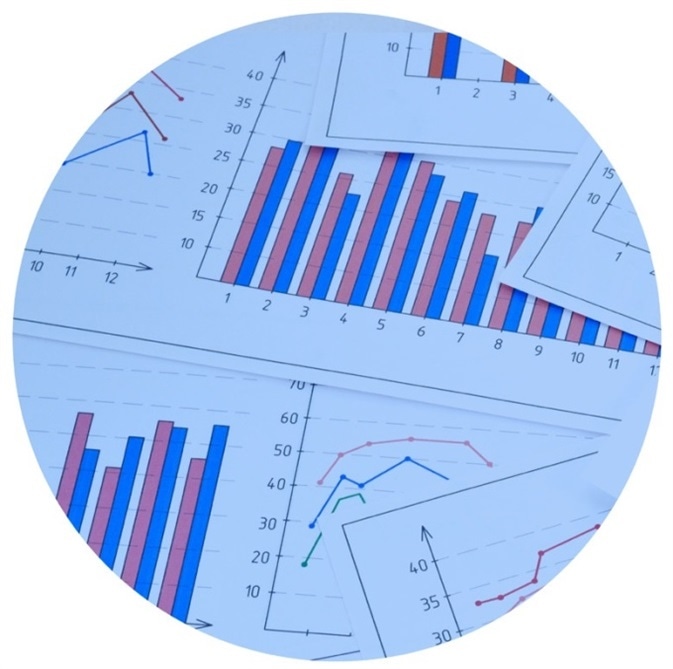
It is possible to avoid the issue of data silos by inputting data into an ELN or ERP system, as these allow data access to be given to selected individuals. It is also possible to set up remote data access with web-based interfaces. This process, as with those mentioned above, may be acceptable for quality systems.
The main disadvantage of ELN and ERP systems is that they are more expensive to set up than the earlier mentioned methods. These costs include not just initial set-up expenses, but also the possibility of monthly subscription charges.
Furthermore, concerns with data integrity are not avoided through manual data entry into ELNs and ERP systems, as they are still vulnerable to human error.
Pros
Overall, the pros of ELN and ERP systems are that they can uphold data security while reducing the risk of data silos. They are appropriate for use with complex data sets, and may be compliant with quality systems.
Cons
The downsides of these methods are that they are less cost-effective and that the risk of human error in data entry is still apparent.
Wireless IoT Add-On Devices
The most recently developed method for capturing data from scientific equipment is with the use of wireless IoT add-on devices. For this approach, compact IoT-equipped instruments are connected to scientific instruments for the exporting of data.
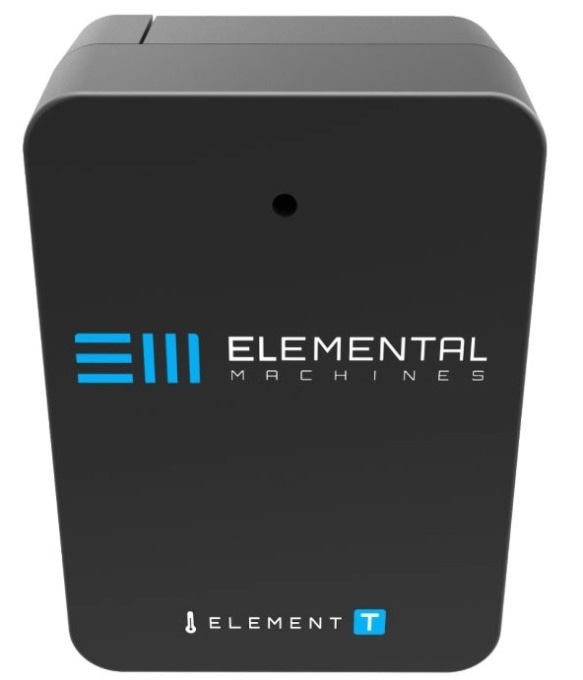
These IoT devices collect both equipment data and metadata (including calibration, QC data and sample results) automatically, and relay it to a cloud-based dashboard for access which is time and location independent.
As these devices are constantly available for data capture, it is possible for staff to monitor data collection remotely, including overnight and at the weekend.
With these systems, it is even possible to enable email and/or SMS alerts when data alters from user-specified conditions. This improves levels of reproducibility and allows for more effective troubleshooting.
Automated data collection is valuable even at times when staff are available on-site, as this allows them to be freed up for performance of other, higher value tasks.
One thing that must be considered when considering IoT add-on devices is the initial upfront expenditure. This must, however, be considered alongside the cost savings that will be achieved through automated data collection.
Pros
Overall, the pros of IoT add-on devices are that they offer automated data collection with a high level of data security and low risk of data silos. They are compatible with complex data sets, may be compliant with quality systems, and support 21 CFR Part 11 workflows and other regulatory standards.
Cons
The sole downside identified with the use of this method is the somewhat higher initial cost of setup.
Reference
- Vines, TH et al. (2013). The Availability of Research Data Declines Rapidly with Article Age. Current Biology, 21(1), 94-97.
About Elemental Machines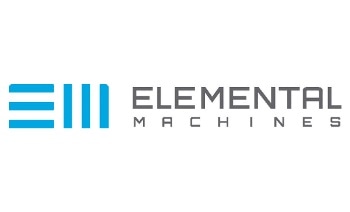
Elemental Machines is helping scientists improve experimental reproducibility and accelerate scientific discovery.
From early research and discovery to manufacturing, everyone in biology and chemistry-based industries knows that the physical environment can affect the entire product lifecycle. What they don’t know is exactly how, or the cumulative cost of not knowing -- higher R&D expenses, slower time to market for innovative products and life-saving therapies, yield loss during manufacturing, and more.
With a deep understanding of these issues, Elemental Machines is delivering unprecedented insight into complex processes, helping customers refine and accelerate their work across all phases of product innovation.
By gathering and synthesizing environmental data into actionable information, Elemental Machines provides critical insights that improve transparency, repeatability and outcomes, and save customers time and money.
Track contextual variables (temperature, humidity, air pressure and light) in the research lab or monitor critical equipment performance (freezers, refrigerators, ovens, and incubators) for easy access to performance data, as well as alerts if readings are out of range.
Sponsored Content Policy: News-Medical.net publishes articles and related content that may be derived from sources where we have existing commercial relationships, provided such content adds value to the core editorial ethos of News-Medical.Net which is to educate and inform site visitors interested in medical research, science, medical devices and treatments.