Sponsored Content by PittconFeb 16 2018
An interview with Prof. Christy Landes, conducted by Stuart Milne, BA.
Can you give a brief introduction to your work in single-molecule spectroscopy, and how it can help examine the link between structure and function in biological molecules?
I’ve been working in single-molecule spectroscopy since my postdoc at UT Austin in 2004. My postdoc training threw me straight into the deep end. I showed up at UT and my boss said, "You're going to work on this protein that is important for HIV's virulence", and I had to learn and see what a virus was, and then I had to learn what single-molecule spectroscopy was.
The moment that I really got into it, I understood, first of all, how different biomolecules and biopolymers are from normal molecules.
For any material that exhibits intrinsic structural or dynamic heterogeneity, only single molecule methods make it possible to directly correlate conformational changes with dynamic information like reaction rate constants or product specificity. Ensemble methods would average out all of those properties.
The clearest example is with an enzyme that switches between bound (active) and unbound (inactive) conformations in the presence of its target. If we measured using standard methods, we could extract, at best, the average conformation (which would be something in between the bound/unbound forms and neither useful nor accurate) and the equilibrium binding coefficient. With single molecule methods, in the best case we could extract each conformation and the equilibrium rate constants for the forward and reverse process.
© molekuul_be/Shutterstock.com
As a spectroscopist, if I'm studying say acetone, every molecule of acetone is exactly the same. If you're studying vibrations, there's obviously a thermal distribution of vibrational energies in all the acetone molecules in a bottle or a vial. If you’re studying biopolymers or biomolecules like proteins or DNAs, their structure is constantly changing depending on their targets. You can't capture the intricacies of the structural changes as a function of the reaction happening with standard spectroscopy. All you ever get is an average.
It's very similar to if you want to understand the structure of a protein. Protein crystallography is incredibly powerful, but it involves freezing the protein structure into what is usually considered the most probably confirmation, which is one of the low energy structures that protein can form. If you want to actually understand how the protein is performing its function, its function is exactly related to its structure and how the structure changes. To be able to see that dynamically, the only way to do it really is with single-molecule spectroscopy.
What first interested you about single-molecule spectroscopy, and how did you come to work in the field?
I became fascinated with the challenges associated with the method, but also its strengths and how it fundamentally links statistical mechanics to experimental observables. With ensemble methods, you measure average values and extract statistical relevance, but with single molecule methods, it is possible to design an experiment in which the probability distribution is your direct observable.
What are the main challenges in measuring the fluorescence signals from a single molecule, or a single chemical reaction event?
I'm a spectroscopist at heart and so of course learning something new and unprecedented about a biomolecule is important and interesting. But the thing that really gets me excited is tackling the challenges that are associated with doing these types of measurements and figuring out new and interesting ways to solve those problems.
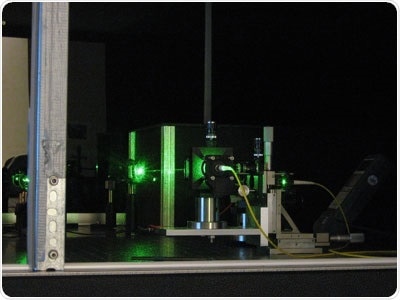
© http://www.lrg.rice.edu/
There are three main challenges. In single-molecule fluorescence spectroscopy or microscopy, the quantum yield of a fluorescent molecule can approach 100%, but still that means if you're only measuring the fluorescence from a single molecule, that's very few photon counts per unit of time and space. Collecting those photons accurately and precisely and being able to distinguish emitted photons from the noise that's associated with every type of measurement and background that's associated with every type of measurement is a very interesting challenging problem that single-molecule spectroscopists are working on.
The second is data processing: most measurements involve high volumes of data that is almost empty of information. Twenty, even ten years ago, if you’re a spectroscopist you would work all day and all night to align the laser so that you could collect a few data points at 3:00 in the morning and be thankful for your 10 data points that you would then try to then fit to an exponential function or a Gaussian function. Single-molecule spectroscopy has advanced so quickly that we're able collect terabytes of data in just a couple of hours, which weren't even possible to store just a few years ago.
Almost by definition, most of your sample has to be nothing but background in order to get single molecule resolution. Processing a terabyte of data in which most of the bits of data are background, but hidden within are small amounts of incredibly rich details a very interesting information theory problem. We're active in developing new data analysis methods based on information theoretic principles.
The third is that labelling and handling single biomolecules introduces the possibility for the biomolecule to alter the label’s quantum yield and for the tagging molecule to alter the biofunctionality. You can’t totally solve this problem, but we must minimize it and quantify it, which involves a lot of tedious but important control measurements.
How has the hardware and/or software influenced the ability to process these large data sets?
I really think it's both, at least that's my opinion. One of the reasons this is possible is that data storage is cheap now. I remember how much I prized my floppy disk. I kept rewriting it and reformatting it to make sure that I would have my 1.44 megabytes worth of information. A lot of this is incredible advances in hardware, but then also the data piece that I'm super excited about.
Everyone throws around this term big data, but it really is true that we're drowning in our ability to collect and save such huge volumes of data that we need to come up with new ways for how to process that data. Actually, I think for the future we need to come up with new ways for how to actually collect it. I think the ideal spectroscopy experiment is adaptive in that you use smart algorithms while you're collecting the data.
If you can get to a point where the software and algorithms are thinking intelligently enough to do what you want it to do without you even asking to do it, then that processing of data becomes even quicker.
I think in the future collection software will be smart enough to know when it has a good molecule and needs better spatial resolution and better time resolution and will adapt to collect better, finer grained information when you need it, and coarser grained information when you don't.
We're working on using some machine learning to teach our collection software when things look good and when things don't look good, when we need better resolution and when we don't.
Your talk at Pittcon 2018 will revolve around the use of Resolving Interfacial Protein Dynamics by Super Temporal-Resolved Microscopy – can you explain what STReM is, and how it allows you to observe dynamics of single molecules?
STReM stands for Super Time-Resolved Microscopy, and as STORM, PALM, and other methods are designed to improve spatial resolution of optical microscopy, we desire to improve the time resolution. STReM makes use of point spread function engineering to encode fast events into each camera frame.
To some extent, it's actually very related to some of the data science principles that underlie what I mentioned above. The double-helix point spread function with 3D super-resolution microscopy method was developed by a group at the University of Colorado and now lots of other people are designing their own phase masks, and point spread functions. The thing we have in common is signal processing principles at the center.
One of the things that happens in signal processing is all the time you go back and forth between real space and Fourier space because all sorts of say noise filtering happens in Fourier space because you can separate noise from signals in Fourier space that you can't in signal space. That's why, for example, FT IR or FTN NMR is so much better than regular IR and regular NMR.
What these guys at Colorado recognized is that if they manipulated the phase of the light from their microscope, they could compress three-dimensional information about their sample into the two-dimensional point spread function that they collected on their camera at the image plane. It's super smart. You're doing exactly the same measurements with exactly the same laser or lamp on exactly the same sample in the microscope.
The only difference is that you allow yourself some room at the detection part of your microscope to manipulate the phase of a light such that your point spread function is no longer an Airy disk but this two-lobed double-helix point spread function, and the angle that point spread function tells you the three-dimensional position of your sample in your sample plane even though you're only doing a 2D image. It's genius. It's super cool.
STReM is built on that principle. I'm interested in dynamics. I want to see how proteins interact with interfaces, and lots of times that happens faster than my camera frame. Cameras are great and they're getting better all the time, but a camera is very expensive, and so you can't buy a new camera every six months to get slightly better time resolution. What we realized we could do is manipulate the phase of the fluorescence light to get better time resolution.
In other words, very similar to the double-helix 3D method, we are encoding faster information into a slower image. The point spread function is no longer a Gaussian or airy disk. It's these two lobes, and now the two lobes rotate around the center, and the angle tells you the arrival time of your molecule faster than the camera frame. You can get 20 times better time resolution with the same old camera that everybody uses.
What molecules/reactions have you been able to observe so far using this technique?
We are interested in understanding protein dynamics at polymer interfaces for the purpose of driving predictive protein separations. The improved time resolution of STReM is revealing intermediates that are suggestive of adsorption-mediated conformational rearrangements.
Protein separations are getting increasingly important as the pharmaceutical industry moves away from organic molecules as therapeutics. The industry is moving towards therapeutics that are often proteins or peptides or sometimes they're DNA or RNA aptamer-based. Separating a protein therapeutic from all the other stuff in the growth medium is hugely time-intensive because at the moment there's no way to optimize a separation predicatively. It's done empirically. There's a bunch of empirical parameters that are just constantly messed with to try to optimize it.
Our long-term goal is to find a way to be predictive about protein separations. What that means underneath is being able to exactly quantify exactly what a protein does at that membrane interface in terms of its structure, its chemistry, its physics, how it interacts with other proteins in a competitive manner. Some of those things are faster than our camera can measure. Ideally we can catch everything, and that's why we need faster time resolution.
How does STReM compare to other single-molecule techniques you’ve employed before, such as FRET or super-resolution microscopy?
STReM is compatible with a wide range of super-resolution methods in that it is possible to combine 2D or 3D super-localization but with the faster time resolution of STReM. There remains the challenge of fitting or localizing more complicated point spread functions.
FRET is a way to measure distance. You would like to understand how protein structures change on the 1 to 10 nanometer scale, but we still don't have a microscope to measure 1 to 10 nanometers. Essentially, you take advantage of this dipole-induced dipole interaction that just happens to be over that length scale, and the math of that energy transfer process actually gets you the distance so you don't have to resolve it. FRET is also a math trick, to me anyway.
The STReM technique, because it involves fluorescence, is very compatible with FRET or any other type of super resolution microscopy. The only challenge is that traditional microscopy approximates that Airy point spread function that you get at the image plane as a Gaussian and it's easy to fit a Gaussian.
Any undergrad with Excel can fit a Gaussian and identify the center and width of the peak, which is what you need for super resolution microscopy, and it's also what you need for FRET for that matter. The point spread functions that you get with these more complicated techniques like STReM or the 3D double-helix are more complicated to fit. Again, the math is more interesting and challenging.
You could do STReM with FRET. You could do STReM with PALM or STORM. People are using that double-helix with STORM, I think, to get 3D images with STORM, but your point spread function fitting and localization is more complicated.
What are the next steps for your own research? And how do you think your findings will contribute to a better understanding in the wider field of biochemistry?
Our big picture goal is to achieve enough mechanistic understanding of protein dynamics at polymer interfaces to transition to a predictive theory of protein chromatography. Protein separation and purification accounts for billions of dollars every year in industrial and bench scale efforts due to the fact that each separation must be optimized empirically.
Where can our readers learn more about your work?
Our newest work on protein dynamics is still under review, but our paper introducing STReM can be found here:
How important is Pittcon as an event for people like yourself to share your research and collaborate on the new ideas?
In the US for general science and I would say medical science, Pittcon is the place to go to get information about the newest ways to measure things. It's the only meeting that's all about measurement. If I have something I need to measure, I go to Pittcon to learn the newest ways to measure it. If I have a new way to measure, I go to Pittcon to make sure that everybody knows that I have a new instrument or method to measure something. It's the only place.
About Christy F. Landes
Christy F. Landes is a Professor in the Departments of Chemistry and Electrical and Computer Engineering at Rice University in Houston, TX. After graduating from George Mason University in 1998, she completed a Ph.D. in Physical Chemistry from the Georgia Institute of Technology in 2003 under the direction of National Academy member Prof. Mostafa El-Sayed.
She was a postdoctoral researcher at the University of Oregon and an NIH postdoctoral fellow at the University of Texas at Austin, under the direction of National Academy members Prof. Geraldine Richmond and Prof. Paul Barbara, respectively, before joining the University of Houston as an assistant professor in 2006.
She moved to her current position at Rice in 2009, earning an NSF CAREER award for her tenure-track work in 2011 and the ACS Early Career Award in Experimental Physical Chemistry in 2016.
About Pittcon
Pittcon® is a registered trademark of The Pittsburgh Conference on Analytical Chemistry and Applied Spectroscopy, a Pennsylvania non-profit organization. Co-sponsored by the Spectroscopy Society of Pittsburgh and the Society for Analytical Chemists of Pittsburgh, Pittcon is the premier annual conference and exposition on laboratory science.
Proceeds from Pittcon fund science education and outreach at all levels, kindergarten through adult. Pittcon donates more than a million dollars a year to provide financial and administrative support for various science outreach activities including science equipment grants, research grants, scholarships and internships for students, awards to teachers and professors, and grants to public science centers, libraries and museums.
Visit pittcon.org for more information.