Results from sensitive RT-PCR tests can be similar in accuracy to the less sensitive antigen tests, providing valuable insight for testing policies in lower- and middle-income countries and regions.
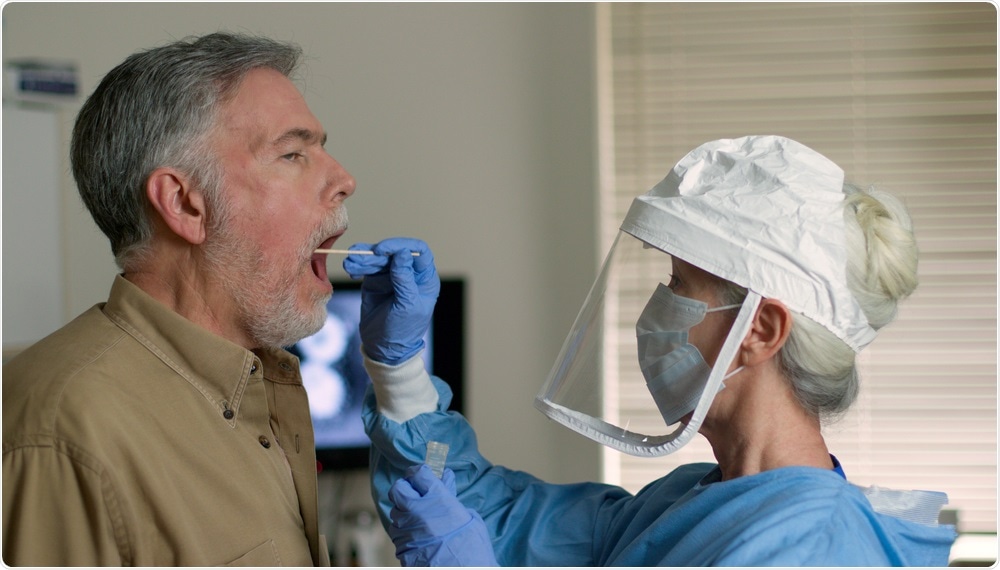
COVID-19 Test. Image Credit: JHDT Productions/Shutterstock.com
Comparing the trade-off between population size and sensitivity for COVID-19 tests
In an attempt to limit the spread of the COVID-19 pandemic, countries around the world have utilized a range of preventative measures including large-scale testing. Testing policies have varied widely, with varying effectiveness, which can be associated with how and when tests were conducted, as well as the type of testing used.
Within countries, testing policies often differ between states. In Indian for instance, states use different mixes of two COVID-19 tests; a very sensitive reverse-transcriptase polymerase-chain-reaction (RT-PCR), which measures RNA levels of the SARS-CoV-2 virus, and a less sensitive rapid antigen test that identifies protein markers on the outside of the virus.
Typically, the RT-PCR approach would be considered the most efficient, accurate, and therefore data can be used to better inform containment and treatment measures ultimately leading to fewer overall infections within the population. However, the RT-PCR tests are more expensive and are slower to provide results relative to the rapid antigen tests.
As such, the exact balance of test types required to obtain accurate epidemiological data needs to be determined. So far this has remained unclear, and the cost to sensitivity constraints have yet to be weighed with real-world data.
In a new analysis published in PLOS Computational Biology by Philip Cherian and Gautam Menon of Ashoka University in Sonipat, India, and Sandeep Krishna of the National Centre for Biological Sciences TIFR, Bangalore, India, the researchers suggest that, to minimize the number of infections in a population, the amount of testing matters more than the sensitivity of the tests that are used.
Cherian and colleagues developed a series of computational simulations to examine the infection rates of COVID-19 within a given population using varying combinations of tests. Importantly, the considered also considered the necessary economic trade-offs between test combinations.
The computational models accounted for population movement between locations before estimating the number of infected individuals that would occur by the end of a pandemic if each testing scenario played out.
Ultimately, the simulations indicated that only using rapid antigen tests could achieve similar total infections as using only RT-PCR tests under the single condition that the number of people tested is high enough.
Informing testing measures in lower and middle-income nations
The findings of the analysis suggest that lower and middle-income nations and regional governments may be able to achieve optimal outcomes from COVID-19 testing measures by focusing on ramping up testing using fewer sensitive tests which provide immediate results.
As long as the number of people is high enough, using faster and cheaper antigen tests could therefore provide a promising opportunity to limit the total number of infections. However, this does not exclude using a combination of mixes but rather emphasizes the importance of having large populations tested.
Indeed, authors note different mixes of tests that can yield the biggest reduction in the number of cases, especially given that the costs of testing are falling, which could help limit the economic downside of testing.
Tests are continually improving, and the tradeoffs are in favor of rapid testing, even if it is less sensitive. Modeling the effects of using different combinations of tests, keeping in mind their relative costs, can suggest specific policy changes that will have a substantial effect on changing the trajectory of the epidemic."
Menon
The findings of this study provide useful and promising information for testing policies, and further studies may want to adopt such models to other regions and countries. Moreover, adopting these models could also be useful when considering additional factors affecting testing accuracy, including the presence of variants, and the consideration of varying proportions of vaccinated individuals within populations.